Physics-Informed Neural Network for the Transient Diffusivity Equation in Reservoir Engineering
arXiv (Cornell University)(2023)
Abstract
Physics-Informed machine learning models have recently emerged with some interesting and unique features that can be applied to reservoir engineering. In particular, a physics-informed neural network (PINN) leverages the fact that neural networks are a type of universal function approximators that can embed the knowledge of any physical laws that govern a given data-set in the learning process, and can be described by partial differential equations. The transient diffusivity equation is a fundamental equation in reservoir engineering and the general solution to this equation forms the basis for Pressure Transient Analysis (PTA). The diffusivity equation is derived by combining three physical principles, the continuity equation, Darcy's equation, and the equation of state for a slightly compressible liquid. Obtaining general solutions to this equation is imperative to understand flow regimes in a porous media. Analytical solutions of the transient diffusivity equation usually are very hard to obtain due to the steep gradients of the pressure near the well. In this work we apply physics-informed neural networks to the one and two dimensional diffusivity equation and demonstrate that decomposing the space domain into very few subdomains can overcome the stiffness problem of the equation. Additionally, we demonstrate that the inverse capabilities of PINNs can estimate missing physics such as permeability and sealing fault similar to buildup tests without shutting in the well.
MoreTranslated text
Key words
transient diffusivity equation
AI Read Science
Must-Reading Tree
Example
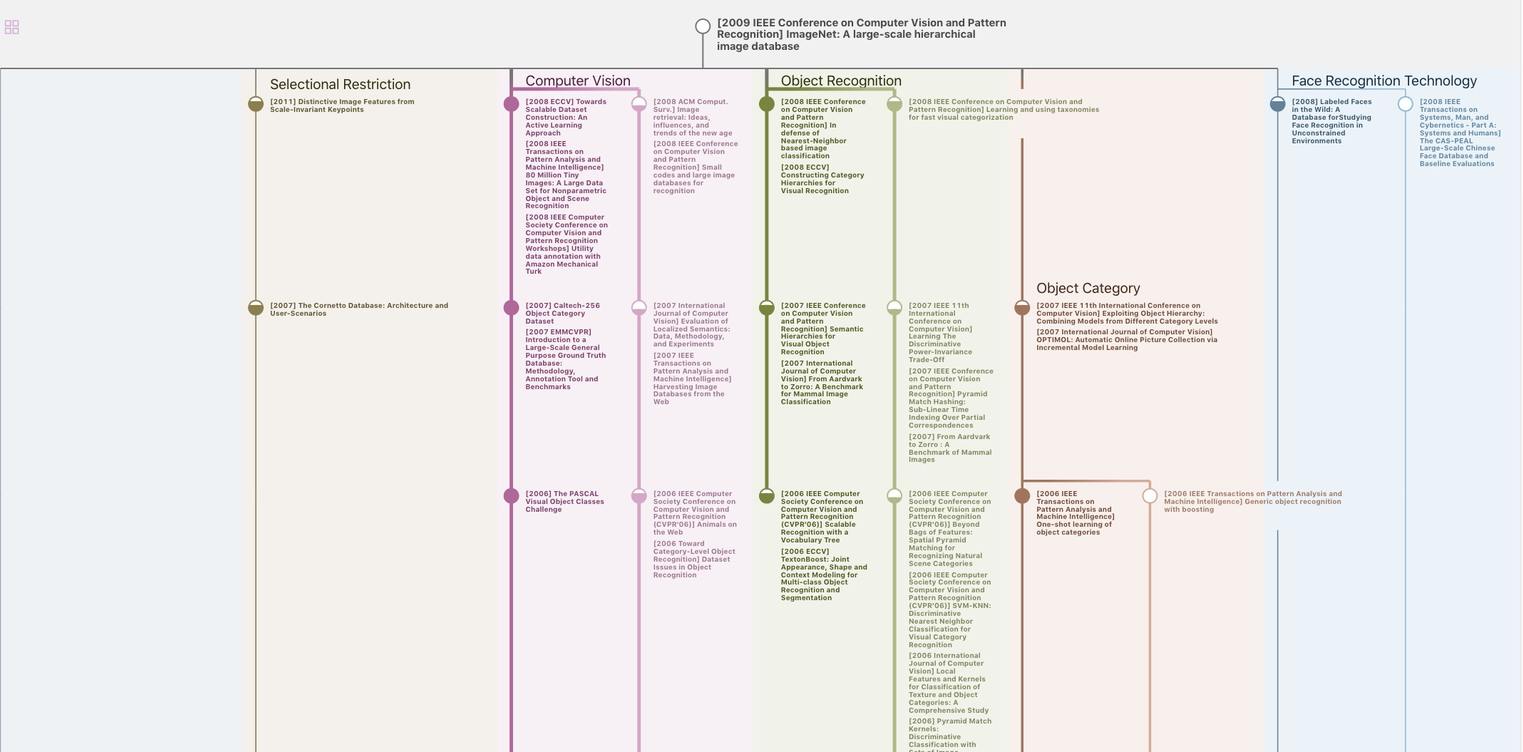
Generate MRT to find the research sequence of this paper
Chat Paper
Summary is being generated by the instructions you defined