Evaluation of Robustness of Deep Learning-Based Object Detection Models for Invertebrate Grazers Detection and Monitoring
KOREAN JOURNAL OF REMOTE SENSING(2023)
摘要
The degradation of coastal ecosystems and fishery environments is accelerating due to the recent phenomenon of invertebrate grazers. To effectively monitor and implement preventive measures for this phenomenon, the adoption of remote sensing-based monitoring technology for extensive maritime areas is imperative. In this study, we compared and analyzed the robustness of deep learningbased object detection models for detecting and monitoring invertebrate grazers from underwater videos. We constructed an image dataset targeting seven representative species of invertebrate grazers in the coastal waters of South Korea and trained deep learning-based object detection models, You Only Look Once (YOLO)v7 and YOLOv8, using this dataset. We evaluated the detection performance and speed of a total of six YOLO models (YOLOv7, YOLOv7x, YOLOv8s, YOLOv8m, YOLOv8l, YOLOv8x) and conducted robustness evaluations considering various image distortions that may occur during underwater filming. The evaluation results showed that the YOLOv8 models demonstrated higher detection speed (approximately 71 to 141 FPS [frame per second]) compared to the number of parameters. In terms of detection performance, the YOLOv8 models (mean average precision [mAP] 0.848 to 0.882) exhibited better performance than the YOLOv7 models (mAP 0.847 to 0.850). Regarding model robustness, it was observed that the YOLOv7 models were more robust to shape distortions, while the YOLOv8 models were relatively more robust to color distortions. Therefore, considering that shape distortions occur less frequently in underwater video recordings while color distortions are more frequent in coastal areas, it can be concluded that utilizing YOLOv8 models is a valid choice for invertebrate grazer detection and monitoring in coastal waters.
更多查看译文
关键词
Deep learning,Object detection,Invertebrate grazers,Robustness test,(jsw@iremtech,co,kr)
AI 理解论文
溯源树
样例
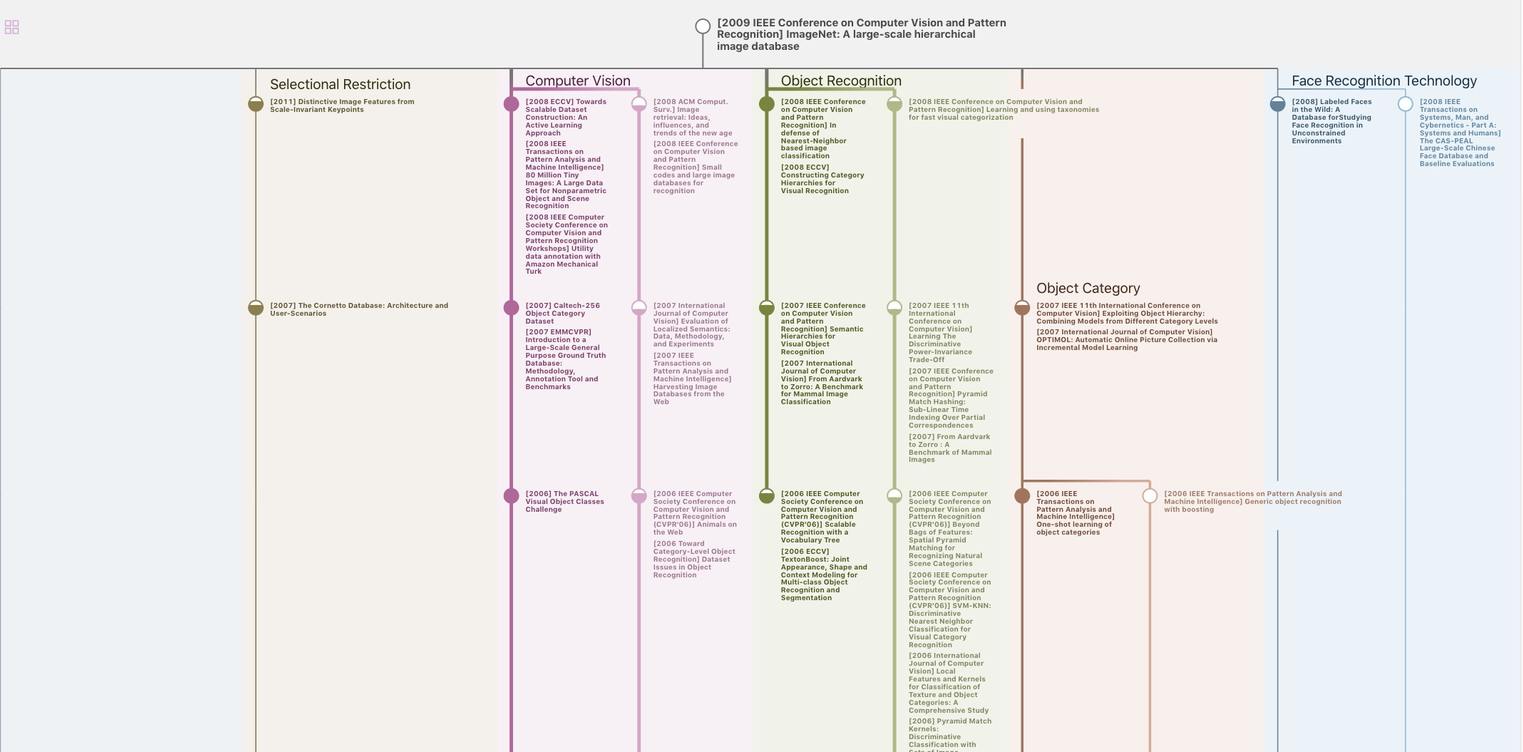
生成溯源树,研究论文发展脉络
Chat Paper
正在生成论文摘要