Self-training and Multi-level Adversarial Network for Domain Adaptive Remote Sensing Image Segmentation
Neural processing letters/Neural Processing Letters(2023)
Abstract
Unsupervised domain adaptive (UDA) image segmentation has received more and more attention in recent years. Domain adaptive methods can align the features of data in different domains, so that segmentation models can be migrated to data in other domains without incurring additional labeling costs. The traditional adversarial training uses the global alignment strategy to align the feature space, which may cause some categories to be incorrectly mapped. At the same time, in high-spatial resolution remote sensing images (RSI), the same category from different scenes (such as urban and rural areas) may have completely different distributions, which severely limits the accuracy of UDA. In order to solve these problems, in this paper: (1) a multi-level adversarial network at category-level is proposed, aiming at integrating feature information in different dimensions, studying the joint distribution at category-level, and aligning each category with adaptive adversarial loss in different dimensional spaces. (2) Use covariance regularization to optimize self-training. A method combining self-training with adversarial training is proposed, optimizes the domain adaptation effect, reduces the negative impact of false pseudo-label iteration caused by self-training. We demonstrated the latest performance of semantic segmentation on challenging LoveDA datasets. Experiments on “urban-to-rural” and “rural-to-urban” show that our method has better performance than the most advanced methods.
MoreTranslated text
Key words
Domain adaptation,Semantic segmentation,Remote sensing images,Self-training,Adversarial network
AI Read Science
Must-Reading Tree
Example
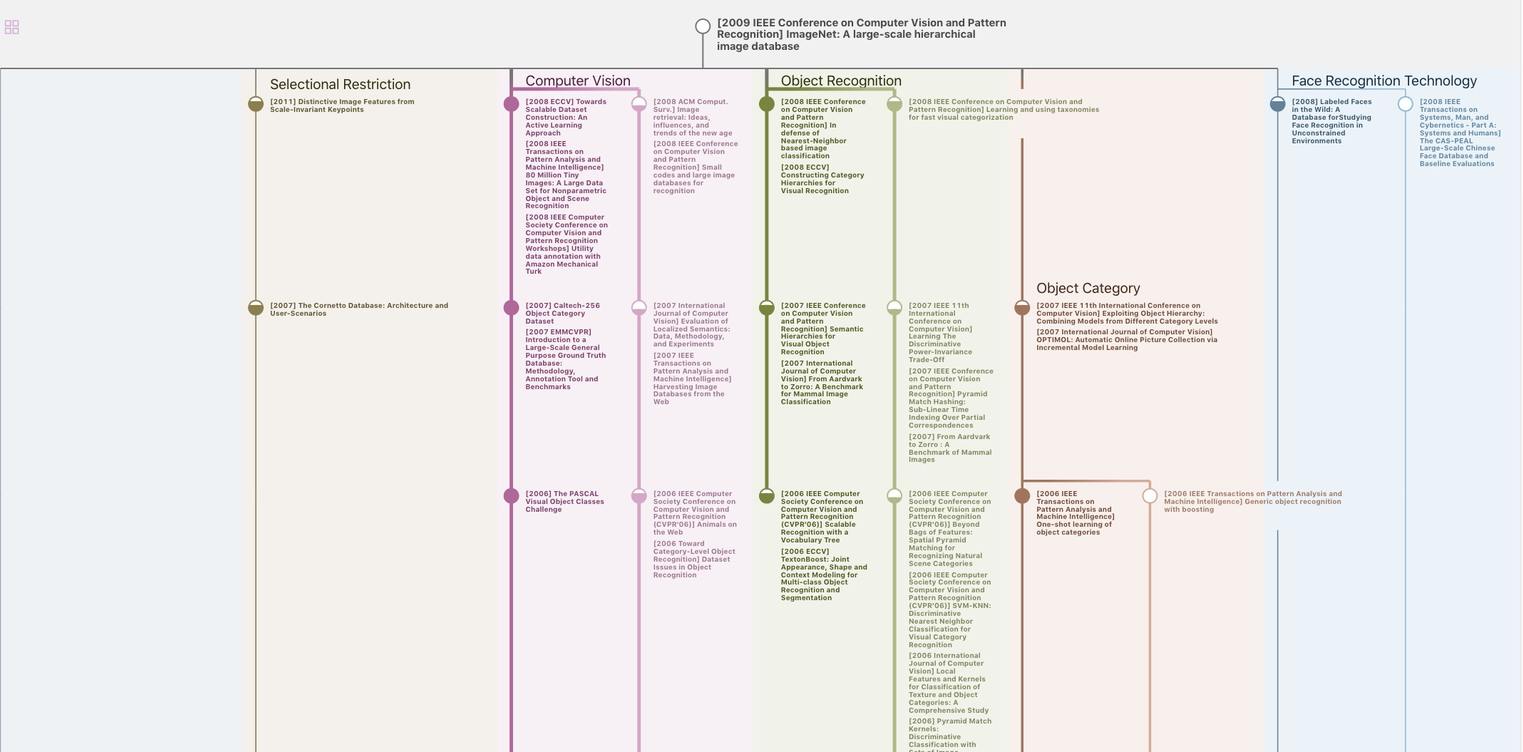
Generate MRT to find the research sequence of this paper
Chat Paper
Summary is being generated by the instructions you defined