A comprehensive review of seismic inversion based on neural networks
EARTH SCIENCE INFORMATICS(2023)
Abstract
Seismic inversion is one of the fundamental techniques for solving geophysics problems. To obtain the elastic parameters or petrophysical parameters, it is necessary to establish a direct or indirect mapping that is usually nonlinear between the observed data and the inversion parameters in seismic inversion. The traditional model-based inversion method, which is calculating complex and expensive, relies on strict physics theories. Neural networks are an excellent artificial intelligence method for establishing nonlinear mapping. Theoretically, any linear and nonlinear functions can be fitted with neural networks. Compared with model-based inversion methods, the inversion efficiency and accuracy can be improved remarkably using neural networks with their powerful ability to discover and extract features from big data. By reviewing the application of fully-connected neural networks, probabilistic neural networks, convolutional neural networks, recurrent neural networks, generative neural networks, and physics-based neural networks in seismic inversion, we provide a comprehensive overview of neural network methods to seismic inversion, including the basic principles of different neural networks, types of seismic inversion, seismic datasets, and the general framework of neural networks for seismic inversion. In addition, the future trends of seismic inversion based on neural networks are also discussed, including the application of image segmentation networks and generative adversarial networks in seismic inversion.
MoreTranslated text
Key words
Seismic inversion,Neural networks,Deep learning,Artificial intelligence,Geophysics
AI Read Science
Must-Reading Tree
Example
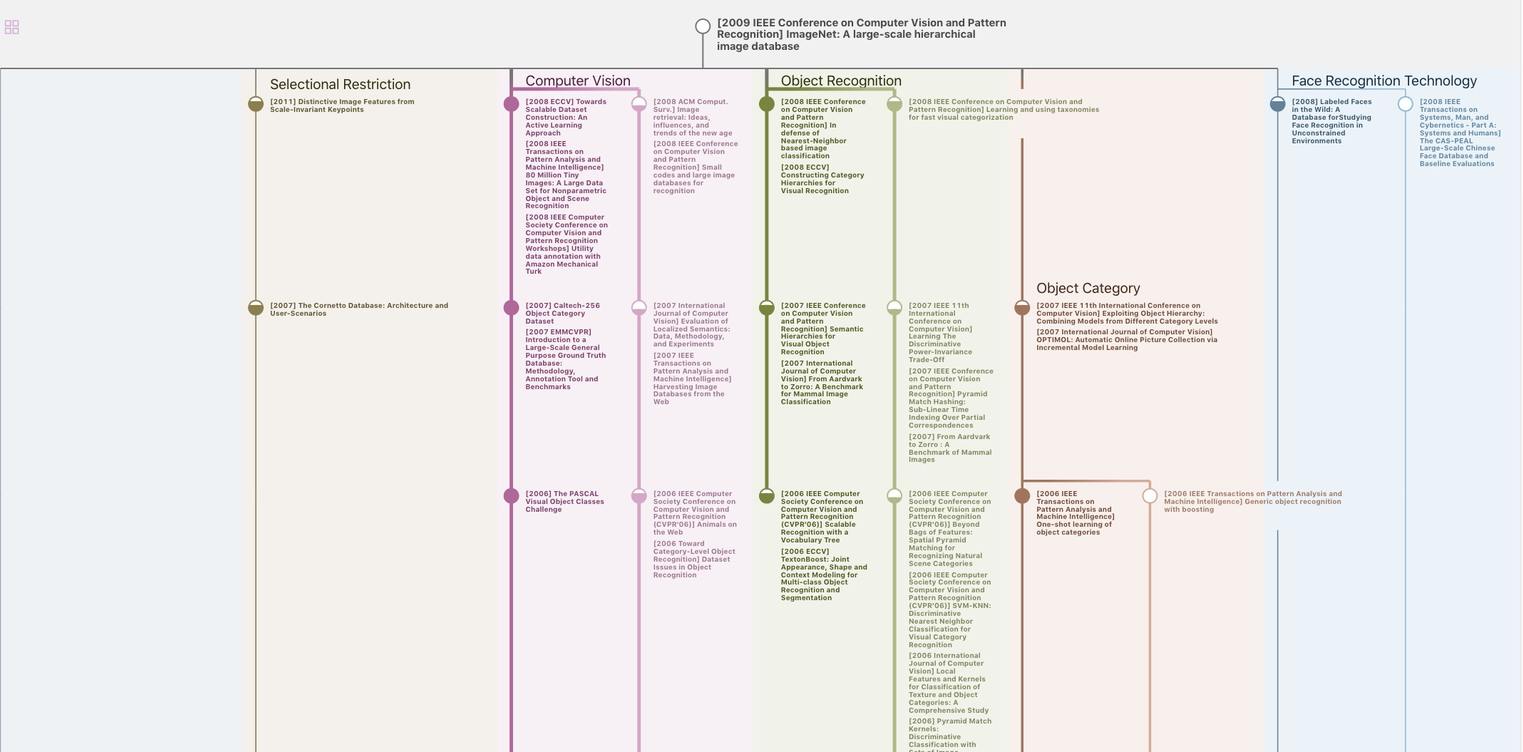
Generate MRT to find the research sequence of this paper
Chat Paper
Summary is being generated by the instructions you defined