Edge Intelligence-Based E-Health Wireless Sensor Network Systems.
ICIS(2023)
摘要
In order to support delay-sensitive applications of healthcare internet of things (HIoT) network systems, it is necessary to allow e-health wearable devices to process their computational applications at an artificial intelligence (AI) based mobile edge computing (MEC) server. Existing works mainly focused on optimising the offloading decisions of all users while minimising their corresponding energy consumption and/or transmission/processing delays. However, the huge data volumes offloading and/or privacy-preserving data processing have been largely ignored. In this paper, we consider an edge intelligence-based e-health wireless sensor network system, where the queues at the MEC server follow M/M/1 queueing model. To minimise energy consumption among all e-health wearable devices, we propose to jointly optimise the FL model task offloading decisions of all e-health wearable devices while guaranteeing delay constraints (which include the transmission delay, queueing delay and processing delay) and global/local FL training accuracy. This is achieved by devising an FL-based edge intelligence queueing offloading decision optimisation algorithm. Simulation results demonstrate that our proposed algorithm achieves a much lower maximum service delay than the benchmarks.
更多查看译文
关键词
Edge AI,e-health,edge computing,resource allocation
AI 理解论文
溯源树
样例
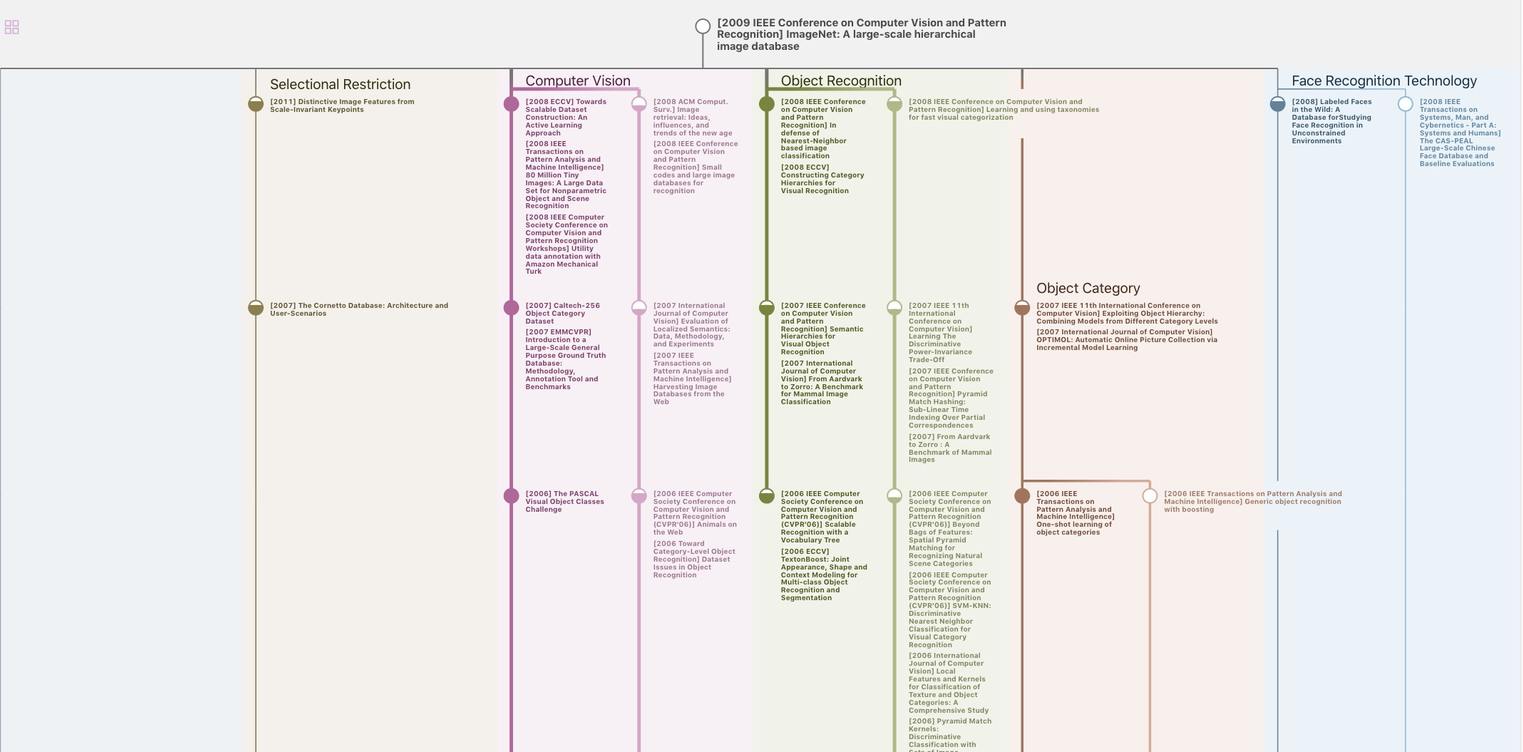
生成溯源树,研究论文发展脉络
Chat Paper
正在生成论文摘要