Calpric: Inclusive and Fine-grained Labeling of Privacy Policies with Crowdsourcing and Active Learning
PROCEEDINGS OF THE 32ND USENIX SECURITY SYMPOSIUM(2023)
摘要
A significant challenge to training accurate deep learning models on privacy policies is the cost and difficulty of obtaining a large and comprehensive set of training data. To address these challenges, we present Calpric, which combines automatic text selection and segmentation, active learning and the use of crowdsourced annotators to generate a large, balanced training set for Android privacy policies at low cost. Automated text selection and segmentation simplifies the labeling task, enabling untrained annotators from crowdsourcing platforms, like Amazon's Mechanical Turk, to be competitive with trained annotators, such as law students, and also reduces inter-annotator agreement, which decreases labeling cost. Having reliable labels for training enables the use of active learning, which uses fewer training samples to efficiently cover the input space, further reducing cost and improving class and data category balance in the data set. The combination of these techniques allows Calpric to produce models that are accurate over a wider range of data categories, and provide more detailed, fine-grained labels than previous work. Our crowdsourcing process enables Calpric to attain reliable labeled data at a cost of roughly $0.92-$1.71 per labeled text segment. Calpric's training process also generates a labeled data set of 16K privacy policy text segments across 9 data categories with balanced assertions and denials.
更多查看译文
AI 理解论文
溯源树
样例
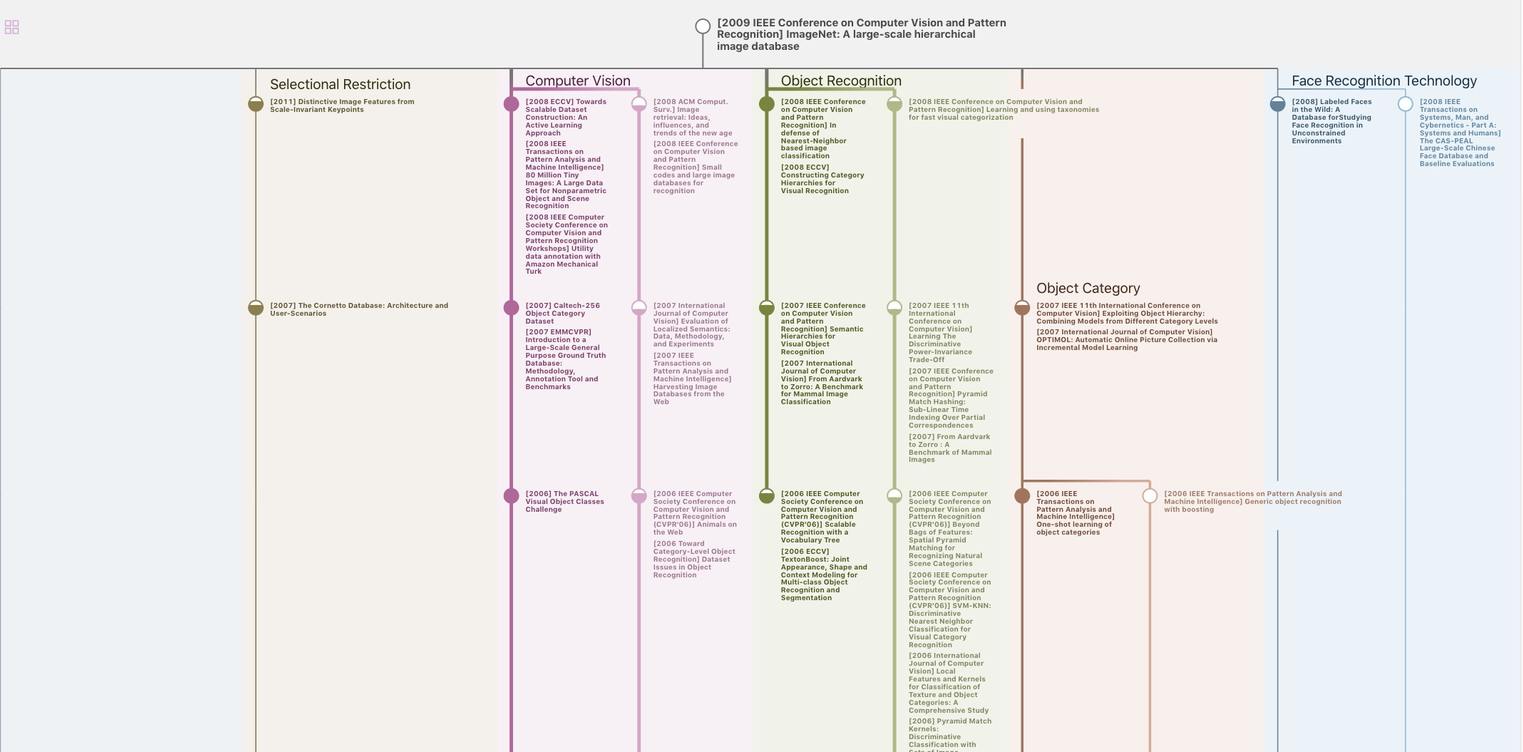
生成溯源树,研究论文发展脉络
Chat Paper
正在生成论文摘要