Enabling local learning for generative-replay-based continual learning with a recurrent model of the insect memory center.
ICONS(2023)
摘要
Continual learning without catastrophic forgetting of previous experiences is an open general challenge for artificial neural networks, but is especially under-explored for artificial neural networks suitable to implement on neuromorphic platforms. An algorithmic understanding of how continual learning occurs in biological neural networks can inform solutions for artificial neural networks, especially in neuromorphic platforms whose biomimetic computing architectures lend themselves to more biofidelic algorithms. In this work, we derive an approach for generative-replay-based continual learning with a three-factor local learning rule based on recurrent connectivity in the insect's memory center, and characterize the model with a CIFAR-100 class incremental continual learning task. First, we investigate the properties of the model's internal representations and find that the high dimensional sparse representations enable this form of generative replay, and that these representations can be binary as required on spiking neuromorphic platforms with little detriment to model performance. Next, we derive a three-factor local learning rule by introducing simplifying assumptions to network updates from error backpropagation optimization which makes the learning rule biologically plausible (i.e., without weight transport) and amenable to neuromorphic implementation. Finally, we find that these simplifications enhance performance during gradient-based optimization for continual learning and when implemented locally achieve this increased performance. Overall, the outcomes of this work can inform a more detailed understanding for continual learning in this biological circuit, as well as introduce general approaches for neuromorphic continual learning.
更多查看译文
AI 理解论文
溯源树
样例
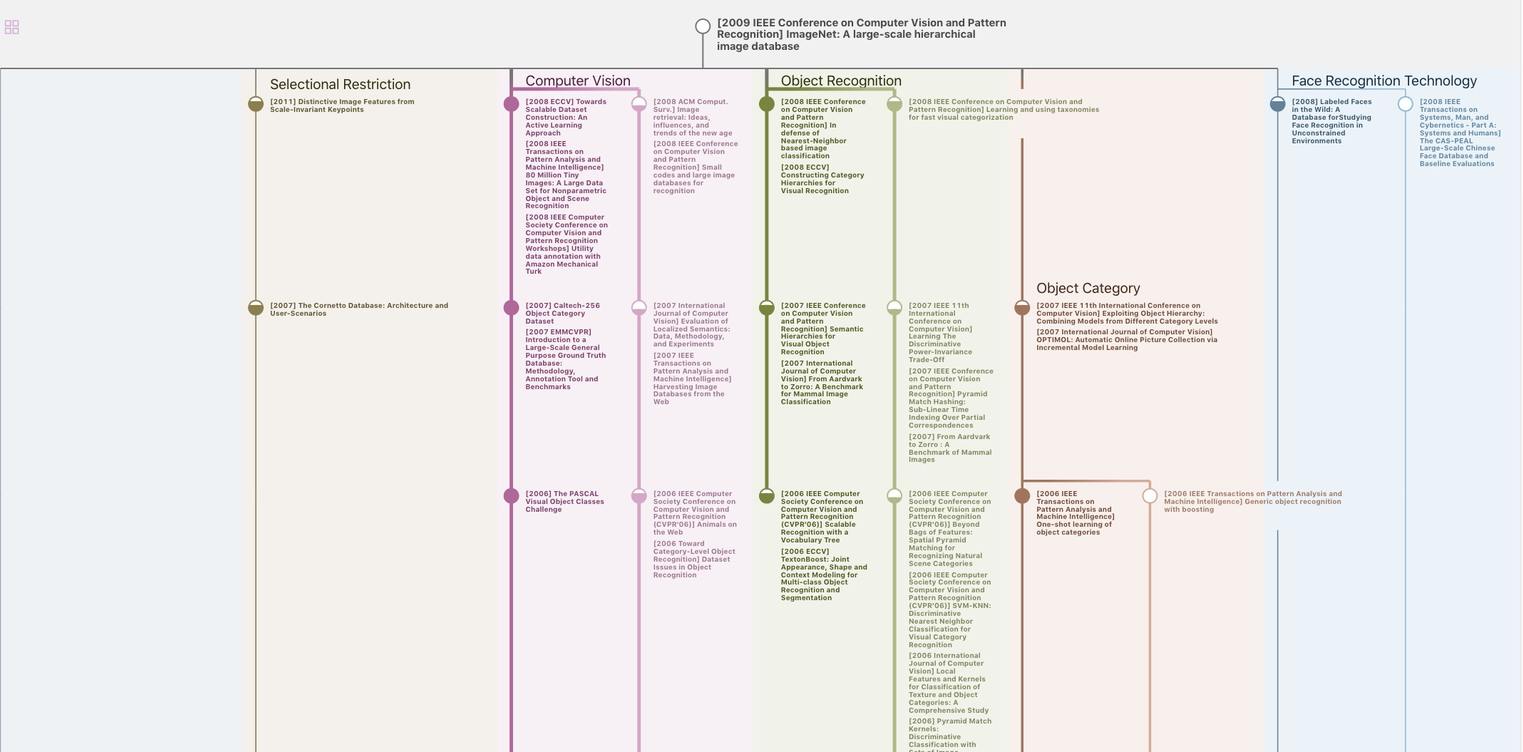
生成溯源树,研究论文发展脉络
Chat Paper
正在生成论文摘要