Variational Information Pursuit with Large Language and Multimodal Models for Interpretable Predictions
CoRR(2023)
摘要
Variational Information Pursuit (V-IP) is a framework for making interpretable predictions by design by sequentially selecting a short chain of task-relevant, user-defined and interpretable queries about the data that are most informative for the task. While this allows for built-in interpretability in predictive models, applying V-IP to any task requires data samples with dense concept-labeling by domain experts, limiting the application of V-IP to small-scale tasks where manual data annotation is feasible. In this work, we extend the V-IP framework with Foundational Models (FMs) to address this limitation. More specifically, we use a two-step process, by first leveraging Large Language Models (LLMs) to generate a sufficiently large candidate set of task-relevant interpretable concepts, then using Large Multimodal Models to annotate each data sample by semantic similarity with each concept in the generated concept set. While other interpretable-by-design frameworks such as Concept Bottleneck Models (CBMs) require an additional step of removing repetitive and non-discriminative concepts to have good interpretability and test performance, we mathematically and empirically justify that, with a sufficiently informative and task-relevant query (concept) set, the proposed FM+V-IP method does not require any type of concept filtering. In addition, we show that FM+V-IP with LLM generated concepts can achieve better test performance than V-IP with human annotated concepts, demonstrating the effectiveness of LLMs at generating efficient query sets. Finally, when compared to other interpretable-by-design frameworks such as CBMs, FM+V-IP can achieve competitive test performance using fewer number of concepts/queries in both cases with filtered or unfiltered concept sets.
更多查看译文
关键词
multimodal
AI 理解论文
溯源树
样例
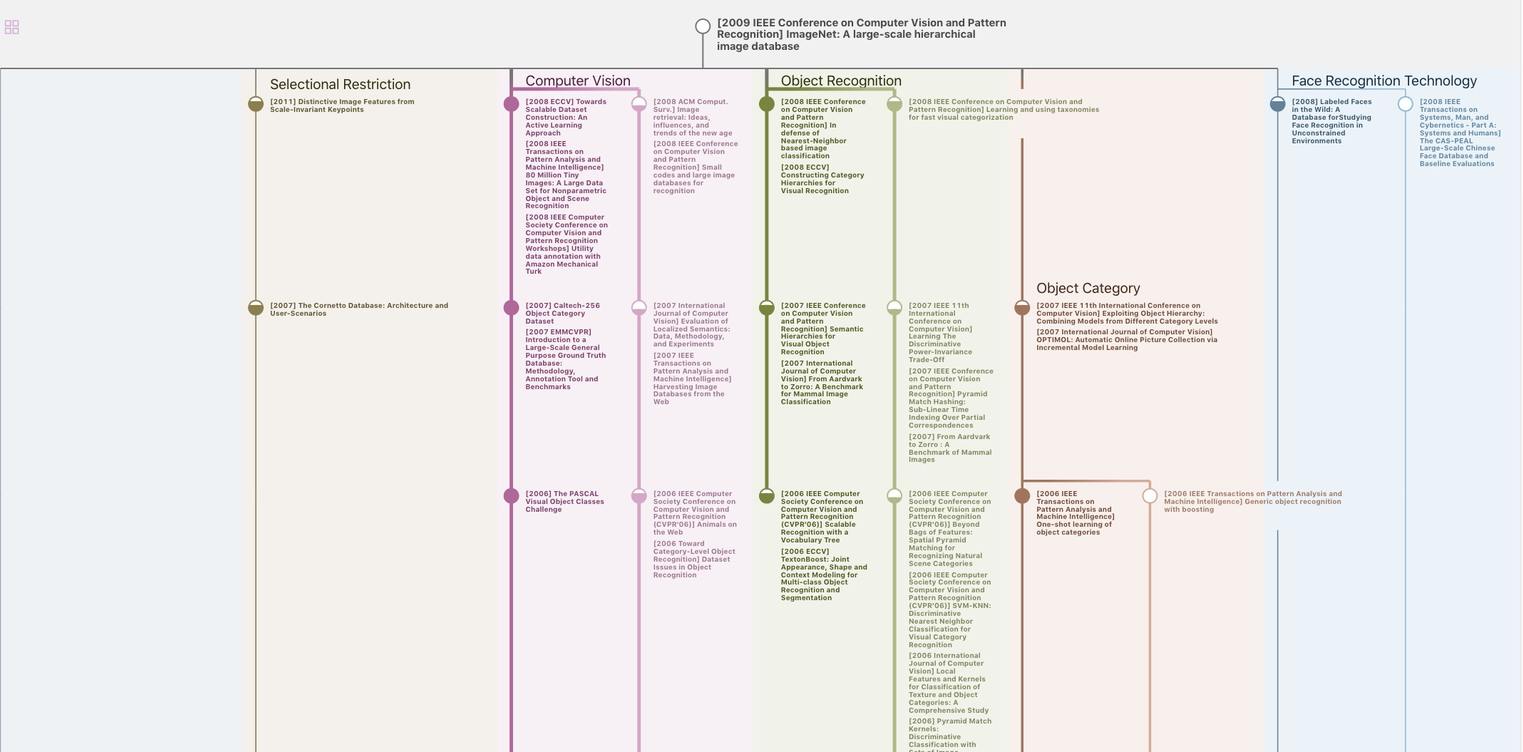
生成溯源树,研究论文发展脉络
Chat Paper
正在生成论文摘要