Shaping the concentration of petroleum hydrocarbon pollution in soil: A machine learning and resistivity-based prediction method
Journal of environmental management(2023)
摘要
A new method relying on machine learning and resistivity to predict concentrations of petroleum hydrocarbon pollution in soil was proposed as a means of investigation and monitoring. Currently, determining pollutant concentrations in soil is primarily achieved through costly sampling and testing of numerous borehole samples, which carries the risk of further contamination by penetrating the aquifer. Additionally, conventional petroleum hydrocarbon geophysical surveys struggle to establish a correlation between survey results and pollutant concentration. To overcome these limitations, three machine learning models (KNN, RF, and XGBOOST) were combined with the geoelectrical method to predict petroleum hydrocarbon concentrations in the source area. The results demonstrate that the resistivity-based prediction method utilizing machine learning is effective, as validated by R-squared values of 0.91 and 0.94 for the test and validation sets, respectively, and a root mean squared error of 0.19. Furthermore, this study confirmed the feasibility of the approach using actual site data, along with a discussion of its advantages and limitations, establishing it as an inexpensive option to investigate and monitor changes in petroleum hydrocarbon concentration in soil.
更多查看译文
关键词
Petroleum hydrocarbon,Resistivity,Organic pollutants,Machine learning,Regression prediction
AI 理解论文
溯源树
样例
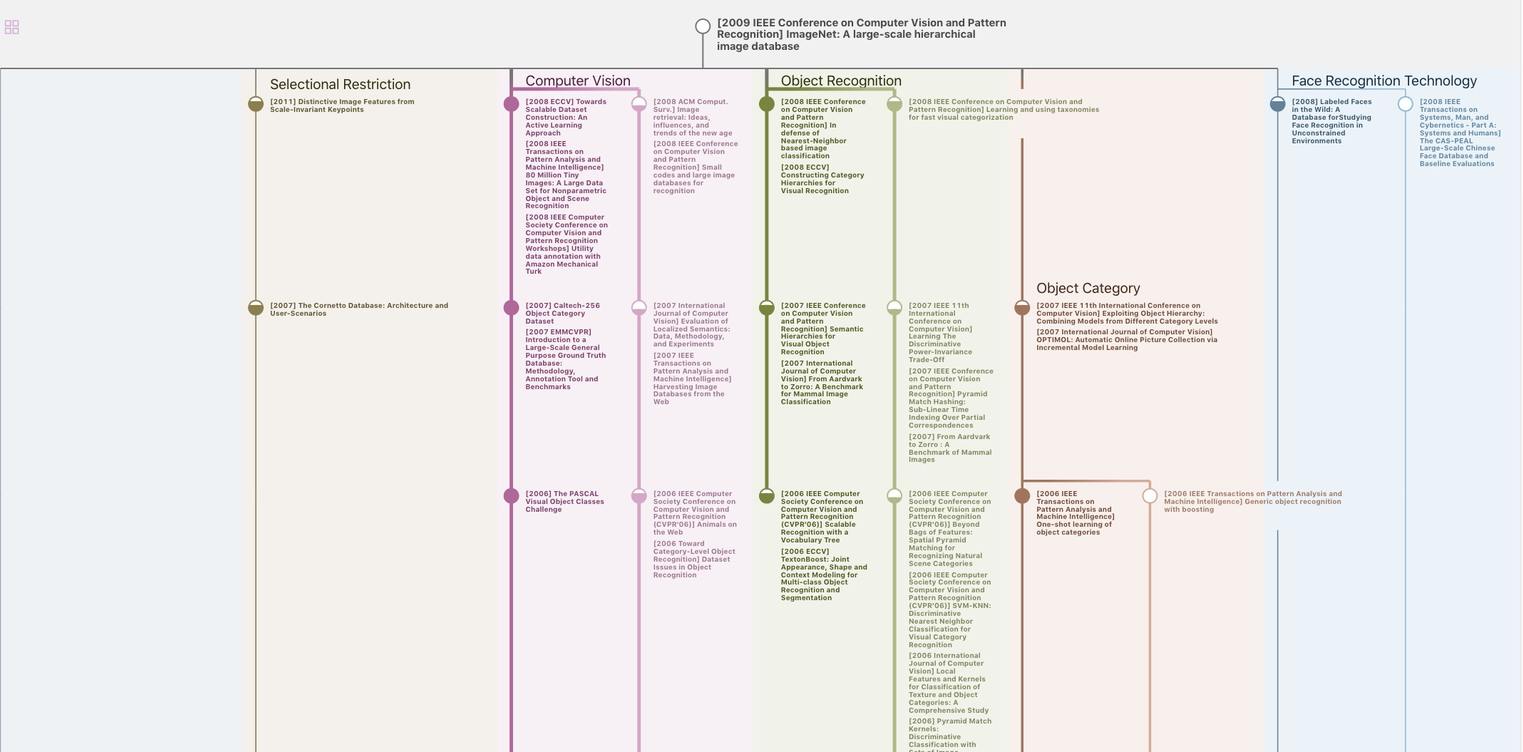
生成溯源树,研究论文发展脉络
Chat Paper
正在生成论文摘要