BundDLe-Net: Neuronal Manifold Learning Meets behaviour
bioRxiv (Cold Spring Harbor Laboratory)(2023)
摘要
Neuronal manifold learning techniques represent high-dimensional neuronal dynamics in low-dimensional embeddings to reveal the intrinsic structure of neuronal manifolds. Common to these techniques is their goal to learn low-dimensional embeddings that preserve all dynamic information in the high-dimensional neuronal data, i.e., embeddings that allow for reconstructing the original data. We introduce a novel neuronal manifold learning technique, BundDLe-Net, that learns a low-dimensional Markovian embedding of the neuronal dynamics which preserves only those aspects of the neuronal dynamics that are relevant for a given behavioural context. In this way, BundDLe-Net eliminates neuronal dynamics that are irrelevant to decoding behaviour, effectively de-noising the data to reveal better the intricate relationships between neuronal dynamics and behaviour. We demonstrate the quantitative superiority of BundDLe-Net over commonly used and state-of-the-art neuronal manifold learning techniques in terms of dynamic and behavioural information in the learned manifold on calcium imaging data recorded in the nematode C. elegans . Qualitatively, we show that BundDLe-Net learns highly consistent manifolds across multiple worms that reveal the neuronal and behavioural motifs that form the building blocks of the neuronal manifold.
### Competing Interest Statement
The authors have declared no competing interest.
更多查看译文
AI 理解论文
溯源树
样例
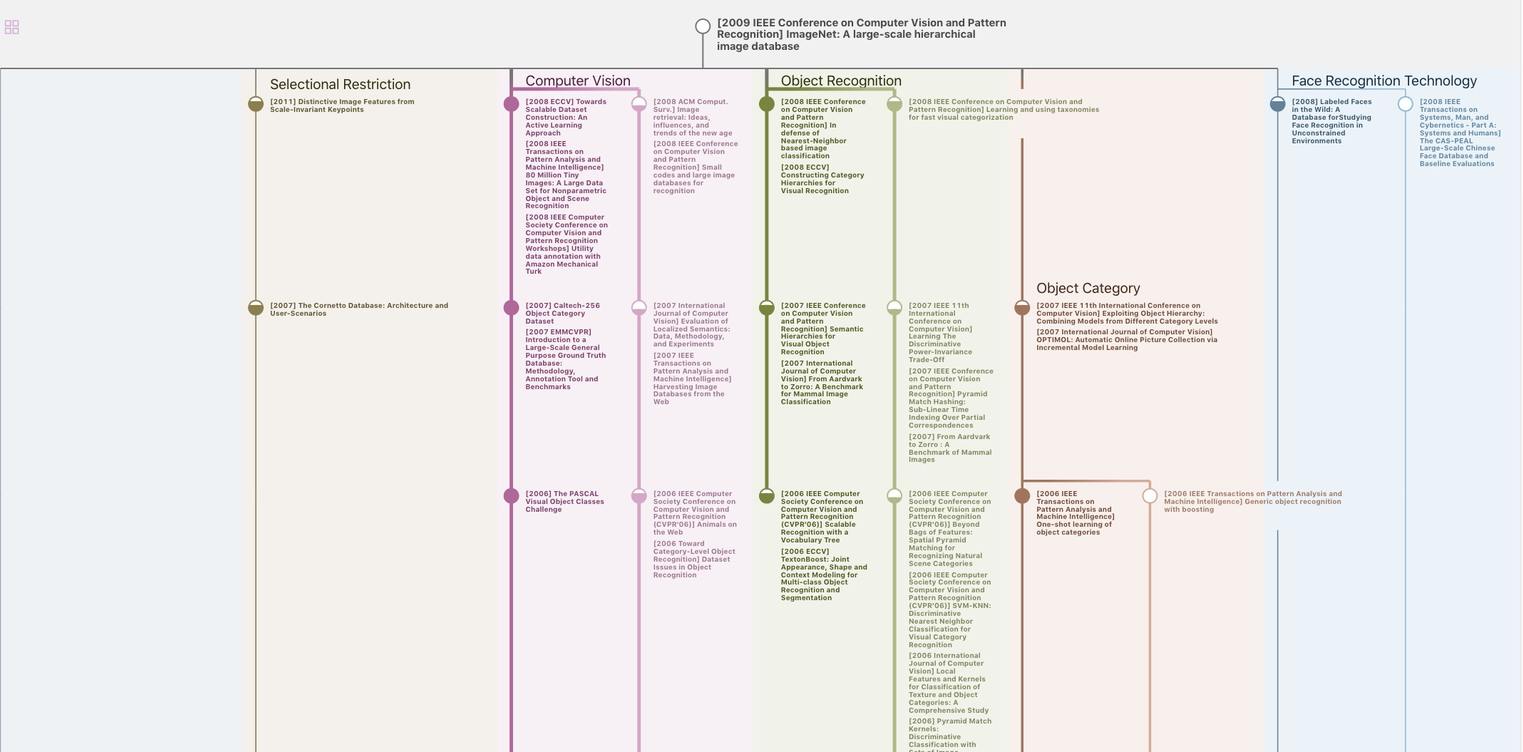
生成溯源树,研究论文发展脉络
Chat Paper
正在生成论文摘要