Deep feature network with multi-scale fusion for highly congested crowd counting
International Journal of Machine Learning and Cybernetics(2024)
摘要
In this paper, we propose a deep feature network with multi-scale fusion (DFNet) for addressing the problem of crowd counting in highly congested noisy scenes. DFNet contains three modules: feature encoder, feature decoder and feature fusion. The feature encoder uses a VGG-16-based convolutional neural network (CNN) that encodes features from images and forms a kind of low-level spatial information. The feature decoder is a multi-column dilated convolutional neural network (McDCNN) with different dilation rates that can capture a multi-scale contextual information, decode the low-level spatial information and generate a kind of high-level semantic information. Furthermore, the multi-column architecture in McDCNN can effectively relieve the “gridding” issue presented in the dilated convolution framework. The feature fusion block uses a simple and effective network architecture to sufficiently incorporate the low-level spatial and the high-level semantic information for facilitating high-quality density map estimation and performing accurate crowd counting. Extensive experiments on several highly challenging crowd counting datasets are conducted. Experimental results show that DFNet is comparable with recent state-of-the-art approaches.
更多查看译文
关键词
Crowd counting,Multi-scale,Dilated convolution framework,Gridding,Low-level spatial information,High-level semantic information
AI 理解论文
溯源树
样例
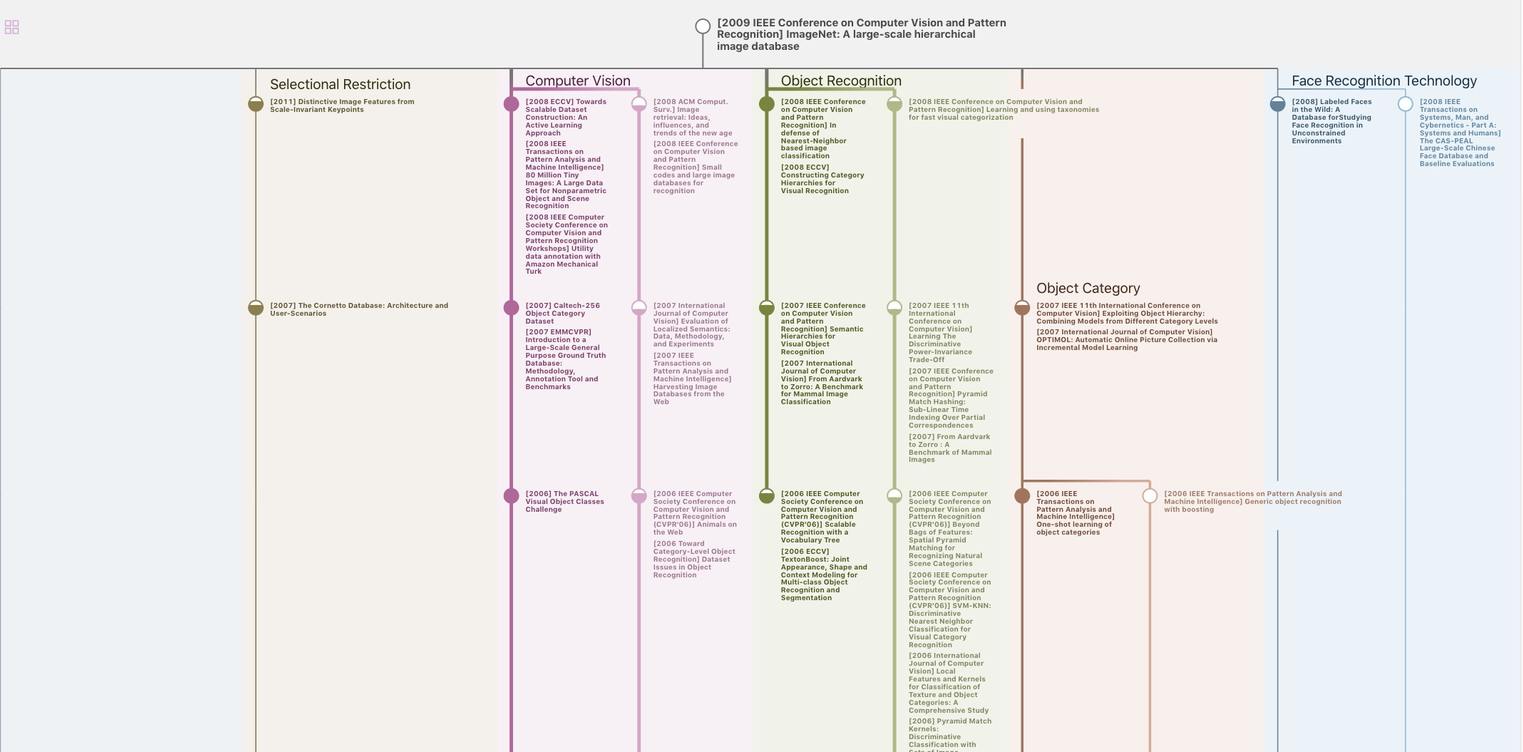
生成溯源树,研究论文发展脉络
Chat Paper
正在生成论文摘要