Real-Time Defect Detection Scheme Based on Deep Learning for Laser Welding System
IEEE Sensors Journal(2023)
摘要
Laser welding, as an important material processing technology, has been widely used in various fields of industry. In most industrial welding production and processing, high precision is required for welding parameters and fixed work pieces. However, in the process of laser welding, serious heat transfer effect will bring unpredictable welding deviations, and even a small deviation will lead to serious welding defects, which will affect the quality of the welded products. Traditional nondestructive testing methods have been widely used, but they have been proved to have some limitations. Existing laser welding defect detection schemes are mainly focused on the detection of postweld defects, which requires a large amount of data, and the real-time detection cannot be guaranteed. In this article, we propose a data acquisition system for collecting changes in physical characteristics during laser welding with the aids of multiple sensors. Based on the data originating from sensors' system, an efficient laser welding defect detection model has been designed and investigated based on the multiscale convolutional neural network (MSCNN), bidirectional long short-term memory (BiLSTM), and attention mechanism (AM). The final proposed MSCNN-BiLSTM-AM fusion detection model can achieve 99.38% detection accuracy, which make the laser welding system more efficient and more suitable.
更多查看译文
关键词
Attention mechanism (AM),data enhancement,defect detection,integrated learning,laser welding
AI 理解论文
溯源树
样例
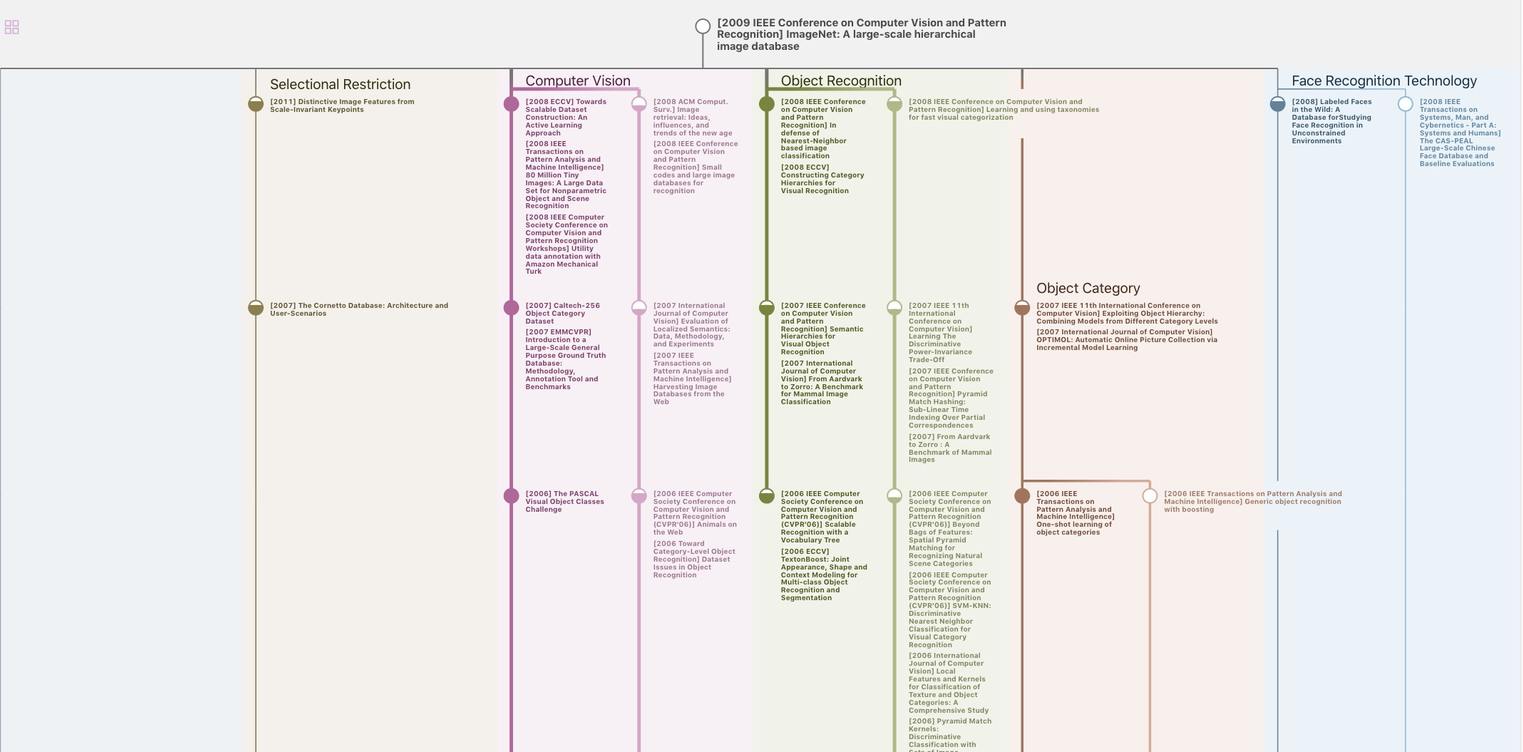
生成溯源树,研究论文发展脉络
Chat Paper
正在生成论文摘要