Segmentation of 4D Flow MRI: Comparison between 3D Deep Learning and Velocity-Based Level Sets
JOURNAL OF IMAGING(2023)
摘要
A thoracic aortic aneurysm is an abnormal dilatation of the aorta that can progress and lead to rupture. The decision to conduct surgery is made by considering the maximum diameter, but it is now well known that this metric alone is not completely reliable. The advent of 4D flow magnetic resonance imaging has allowed for the calculation of new biomarkers for the study of aortic diseases, such as wall shear stress. However, the calculation of these biomarkers requires the precise segmentation of the aorta during all phases of the cardiac cycle. The objective of this work was to compare two different methods for automatically segmenting the thoracic aorta in the systolic phase using 4D flow MRI. The first method is based on a level set framework and uses the velocity field in addition to 3D phase contrast magnetic resonance imaging. The second method is a U-Net-like approach that is only applied to magnitude images from 4D flow MRI. The used dataset was composed of 36 exams from different patients, with ground truth data for the systolic phase of the cardiac cycle. The comparison was performed based on selected metrics, such as the Dice similarity coefficient (DSC) and Hausdorf distance (HD), for the whole aorta and also three aortic regions. Wall shear stress was also assessed and the maximum wall shear stress values were used for comparison. The U-Net-based approach provided statistically better results for the 3D segmentation of the aorta, with a DSC of 0.92 & PLUSMN; 0.02 vs. 0.86 & PLUSMN; 0.5 and an HD of 21.49 & PLUSMN; 24.8 mm vs. 35.79 & PLUSMN; 31.33 mm for the whole aorta. The absolute difference between the wall shear stress and ground truth slightly favored the level set method, but not significantly (0.754 & PLUSMN; 1.07 Pa vs. 0.737 & PLUSMN; 0.79 Pa). The results showed that the deep learning-based method should be considered for the segmentation of all time steps in order to evaluate biomarkers based on 4D flow MRI.
更多查看译文
关键词
segmentation,4D flow MRI,deep learning,aorta
AI 理解论文
溯源树
样例
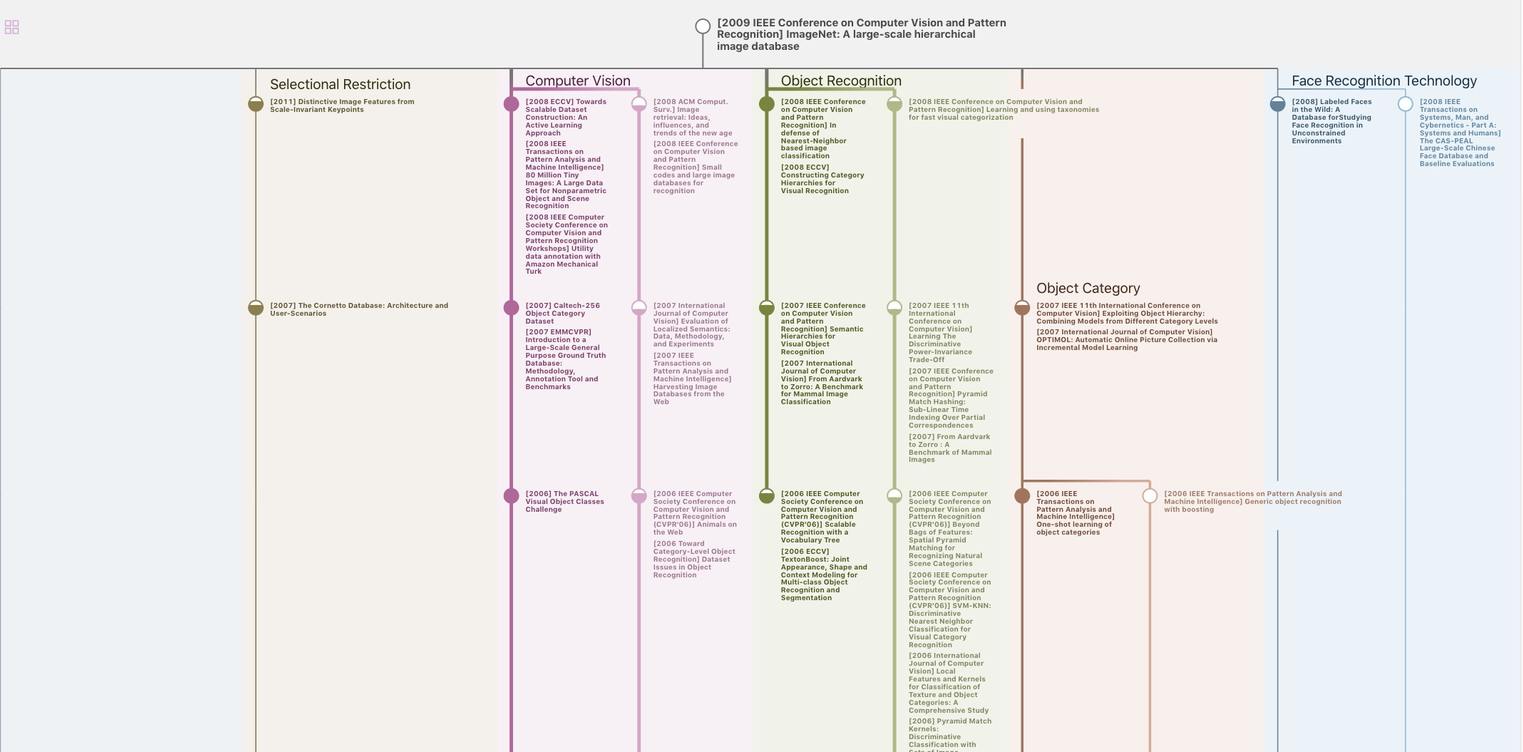
生成溯源树,研究论文发展脉络
Chat Paper
正在生成论文摘要