Automatic and human level Graf's type identification for detecting developmental dysplasia of the hip.
Biomedical journal(2023)
摘要
BACKGROUND:Developmental dysplasia of the hip (DDH) is a common congenital disorder that may lead to hip dislocation and requires surgical intervention if left untreated. Ultrasonography is the preferred method for DDH screening; however, the lack of experienced operators impedes its application in universal neonatal screening.
METHODS:We developed a deep neural network tool to automatically register the five keypoints that mark important anatomical structures of the hip and provide a reference for measuring alpha and beta angles following Graf's guidelines, which is an ultrasound classification system for DDH in infants. Two-dimensional (2D) ultrasonography images were obtained from 986 neonates aged 0-6 months. A total of 2406 images from 921 patients were labeled with ground truth keypoints by senior orthopedists.
RESULTS:Our model demonstrated precise keypoint localization. The mean absolute error was approximately 1 mm, and the derived alpha angle measurement had a correlation coefficient of R = 0.89 between the model and ground truth. The model achieved an area under the receiver operating characteristic curve of 0.937 and 0.974 for classifying alpha <60° (abnormal hip) and <50° (dysplastic hip), respectively. On average, the experts agreed with 96% of the inferenced images, and the model could generalize its prediction on newly collected images with a correlation coefficient higher than 0.85.
CONCLUSIONS:Precise localization and highly correlated performance metrics suggest that the model can be an efficient tool for assisting DDH diagnosis in clinical settings.
更多查看译文
AI 理解论文
溯源树
样例
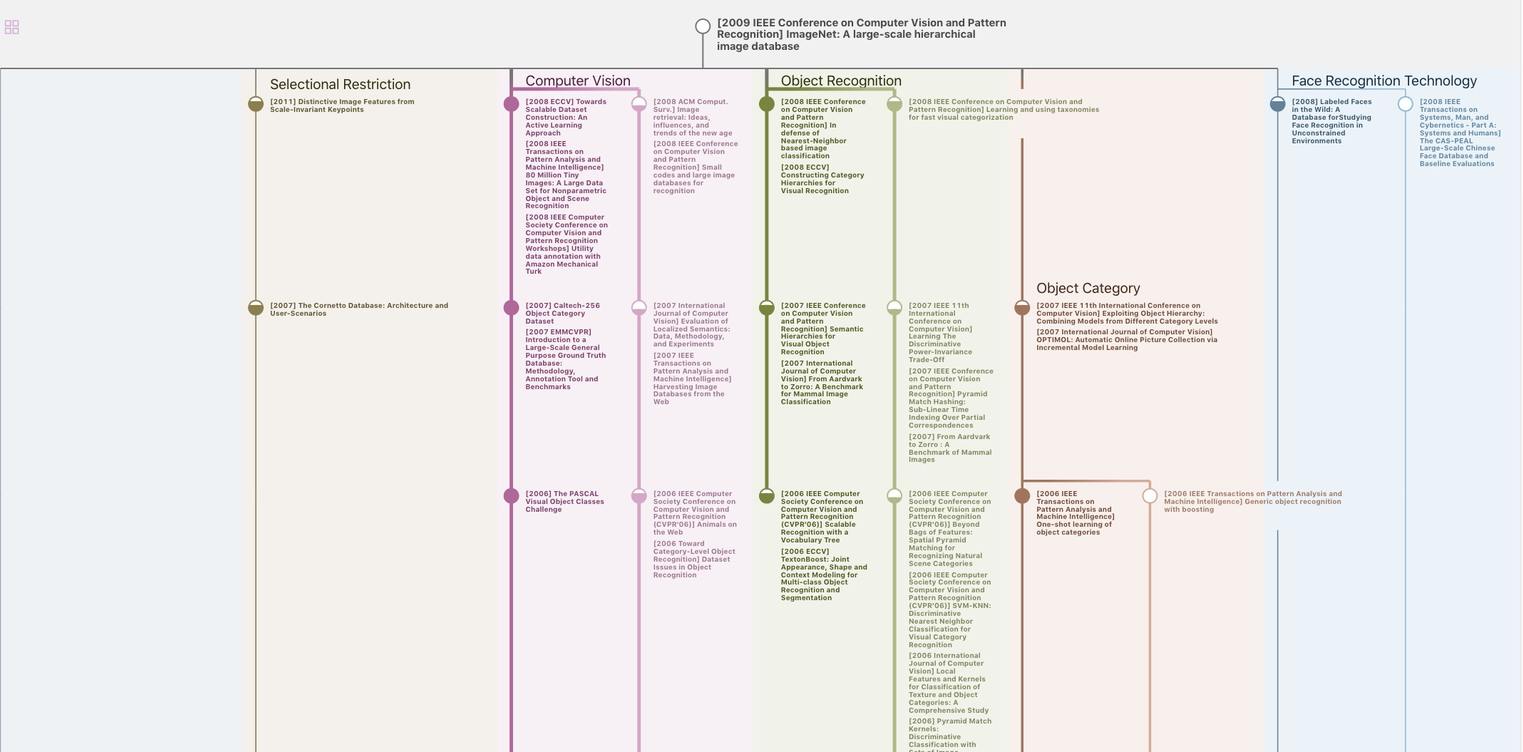
生成溯源树,研究论文发展脉络
Chat Paper
正在生成论文摘要