Epileptic detection in single and multi-lead EEG signals using persistent homology based on bi-directional weighted visibility graphs
Chaos (Woodbury, N.Y.)(2023)
摘要
Epilepsy is a widespread neurological disorder, and its recurrence and suddenness are making automatic detection of seizure an urgent necessity. For this purpose, this paper performs topological data analysis (TDA) of electroencephalographic (EEG) signals by the medium of graphs to explore the potential brain activity information they contain. Through our innovative method, we first map the time series of epileptic EEGs into bi-directional weighted visibility graphs (BWVGs), which give more comprehensive reflections of the signals compared to previous existing structures. Traditional graph-theoretic measurements are generally partial and mainly consider differences or correlations in vertices or edges, whereas persistent homology (PH), the essential part of TDA, provides an alternative way of thinking by quantifying the topology structure of the graphs and analyzing the evolution of these topological properties with scale changes. Therefore, we analyze the PH for BWVGs and then obtain the two indicators of persistence and birth-death for homology groups to reflect the topology of the mapping graphs of EEG signals and reveal the discrepancies in brain dynamics. Furthermore, we adopt neural networks (NNs) for the automatic detection of epileptic signals and successfully achieve a classification accuracy of 99.67 % when distinguishing among three different sets of EEG signals from seizure, seizure-free, and healthy subjects. In addition, to accommodate multi-leads, we propose a classifier that incorporates graph structure to distinguish seizure and seizure-free EEG signals. The classification accuracies of the two subjects used in the classifier are as high as 99.23 % and 94.76 %, respectively, indicating that our proposed model is useful for the analysis of EEG signals.
更多查看译文
关键词
epileptic detection,persistent homology,signals,multi-lead,bi-directional
AI 理解论文
溯源树
样例
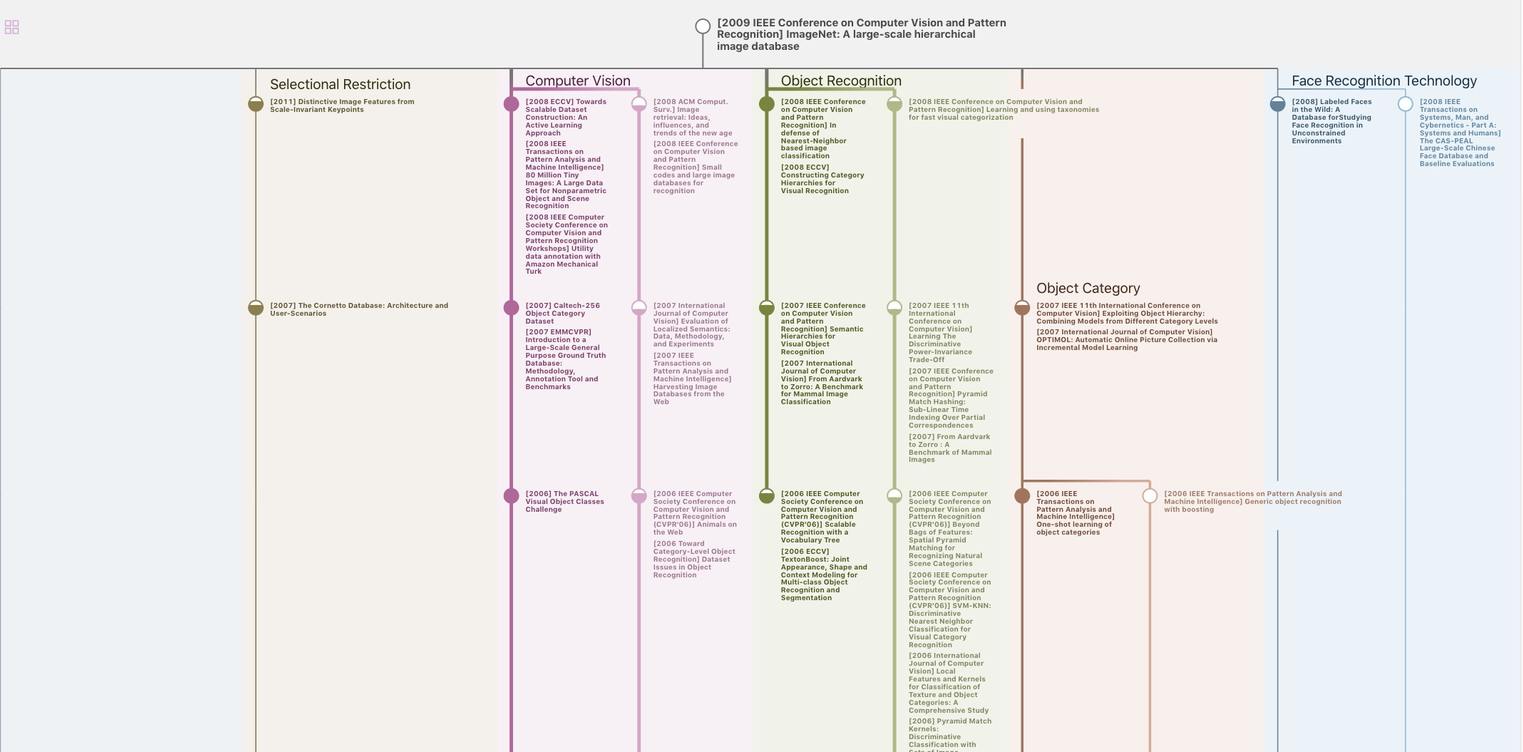
生成溯源树,研究论文发展脉络
Chat Paper
正在生成论文摘要