Retrieving Relevant Context to Align Representations for Cross-lingual Event Detection.
ACL (Findings)(2023)
摘要
We study the problem of cross-lingual transfer learning for event detection (ED) where models trained on a source language are expected to perform well on data for a new target language.Among a few recent works for this problem, the main approaches involve representation matching (e.g., adversarial training) that aims to eliminate language-specific features from the representations to achieve the language-invariant representations.However, due to the mix of language-specific features with event-discriminative context, representation matching methods might also remove important features for event prediction, thus hindering the performance for ED.To address this issue, we introduce a novel approach for cross-lingual ED where representations are augmented with additional context (i.e., not eliminating) to bridge the gap between languages while enriching the contextual information to facilitate ED.At the core of our method involves a retrieval model that retrieves relevant sentences in the target language for an input sentence to compute augmentation representations.Experiments on three languages demonstrate the state-of-the-art performance of our model for cross-lingual ED.
更多查看译文
AI 理解论文
溯源树
样例
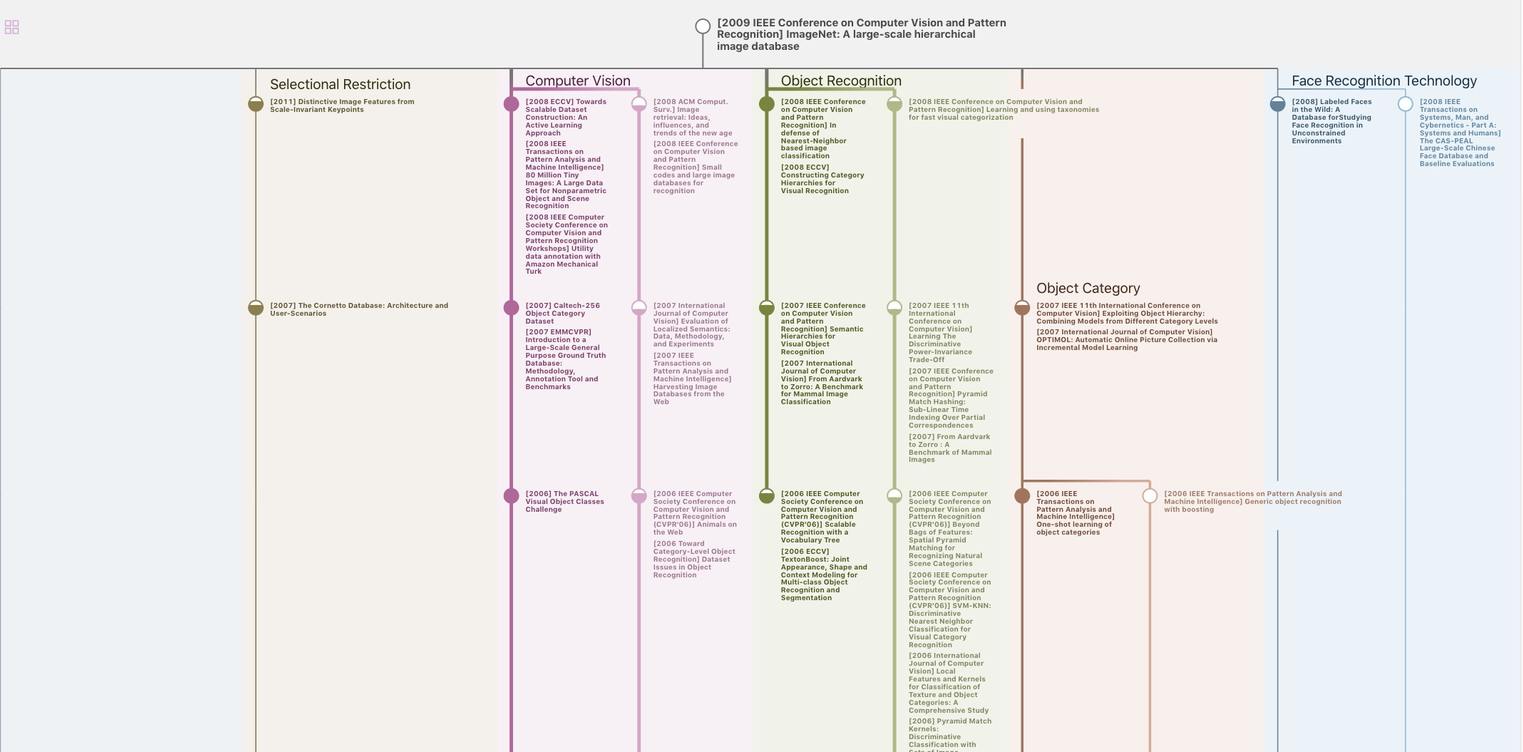
生成溯源树,研究论文发展脉络
Chat Paper
正在生成论文摘要