A New Agent-Based Model Provides Insight Into Assumptions in Modeling Forest Management Under Deep Uncertainty
Research Square (Research Square)(2021)
摘要
Abstract ContextExploratory modeling in forestry uses a variety of approaches to study forest management questions. One key assumption that every approach makes is about the degree of deep uncertainty—the lack of knowledge required for making an informed decision—that future forest managers will face. This assumption can strongly influence simulation results and the conclusions drawn from them, but is rarely studied.ObjectivesOur objective was to measure the degree of deep uncertainty within a forest management simulation to compare alternative modeling approaches and improve understanding of when a specific approach should be applied.MethodsWe first developed a method for measuring the degree of deep uncertainty assumed by approaches to modeling forest management. Next, we developed a new extension to the LANDIS-II model, the SOSIEL Harvest Extension, which simulates alternative approaches to modeling forest management. Finally, we applied the new method and extension to comparing three alternative approaches to modeling forest management in Michigan.ResultsThe degrees of deep uncertainty varied substantially among the three modeling approaches. There is also an overall negative relationship between the degree of deep uncertainty an approach assumes a forest manager will face and the level of flexibility the approach assumes a manager will have in responding to forest change.ConclusionsQuantifying the deep uncertainty inherent in simulated forest management and comparing it across models provides an opportunity to better understand its sources and investigate differences in the assumptions made by alternative modeling approaches.
更多查看译文
关键词
modeling forest management,forest management,agent-based
AI 理解论文
溯源树
样例
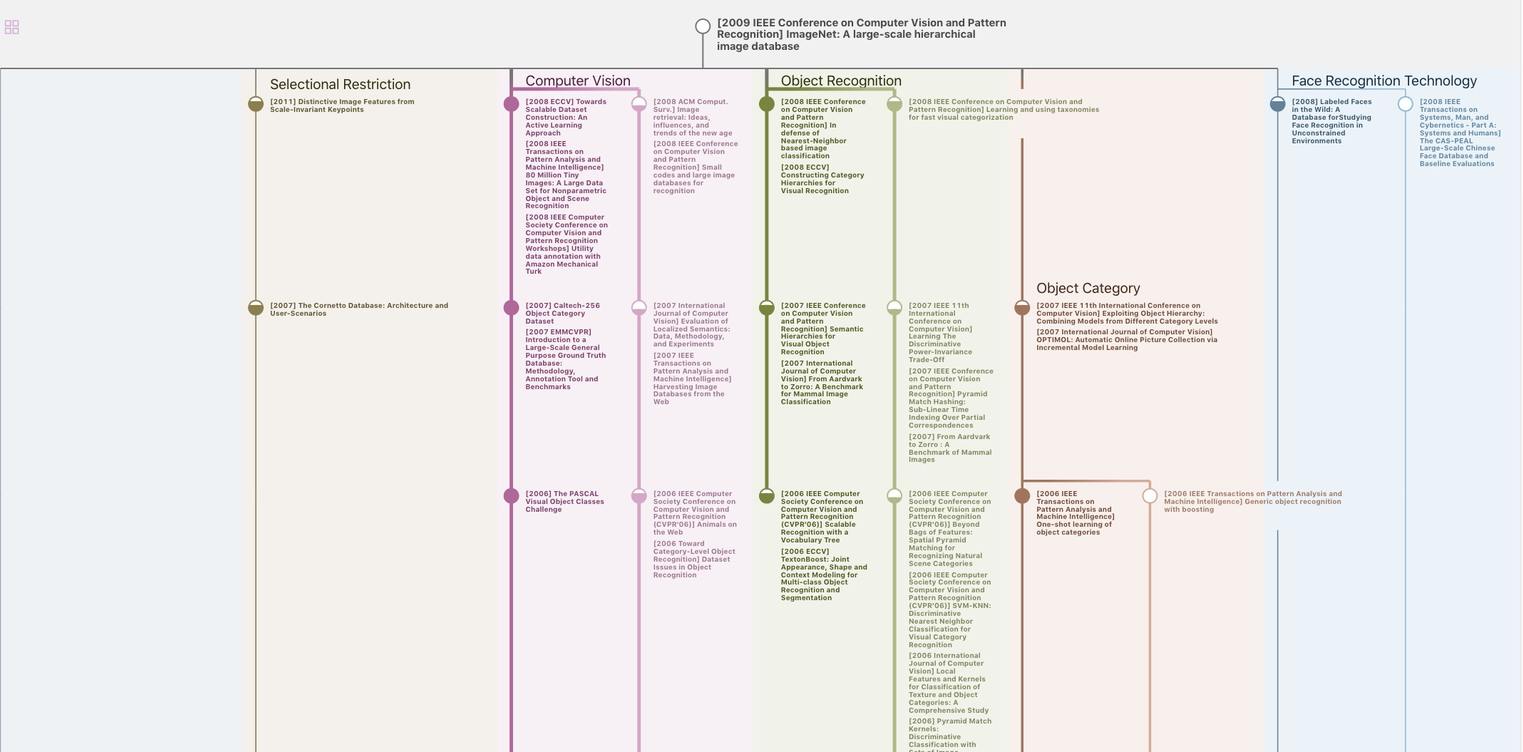
生成溯源树,研究论文发展脉络
Chat Paper
正在生成论文摘要