Solar Irradiance Prediction Using an Optimized Data Driven Machine Learning Models.
Journal of grid computing(2023)
摘要
For a higher degree of penetration of renewable energy into the controls of the existing power system, an accurate solar energy prediction is necessary. Data-driven algorithms may be used to enhance solar generation forecasts as data has now become readily accessible in large quantities. To address these predicting issues in this research article three machine learning models: Support Vector Regressor (SVR), Multilayer Perceptron (MLP) and Random Forest Regressor (RFR) have been incorporated to forecast the Global Horizontal Irradiance (GHI), Diffused Horizontal Irradiance (DHI), Diffused Normal Irradiance (DNI) based on the spatiotemporal factors. In order to improve the prediction accuracy, the parametric tuning of models has been carried out with the two met heuristic algorithms: Moth Flame Optimization (MFO) and Grey Wolf Optimization (GWO) and also validated with the novel application of Evolve Class Topper Optimization (ECTO) method. Corresponding performance measures, including Mean Square Error (MSE), Root Mean Square Error (RMSE), Mean Absolute Error (MAE), Max Error (ME), and Coefficient of Determination (R2), are employed to evaluate each model's performance. The results obtained through a comparative assessment of all machine learning models confirmed that the ECTO based models have outperformed others and the RFR-ECTO model is the best forecasting model having the highest R2 scores of 0.9441, 0.9107 and 0.8882 and the lowest RMSE value of 75.8613 W/m 2 , 40.8714 W/m 2 , 94.8916 W/m 2 for GHI, DHI and DNI respectively which ensures that the designed predictive model can be implemented for prediction of solar energy.
更多查看译文
关键词
SVR,MLP,RFR,MFO,GWO,ECTO,Solar Irradiance,Forecasting
AI 理解论文
溯源树
样例
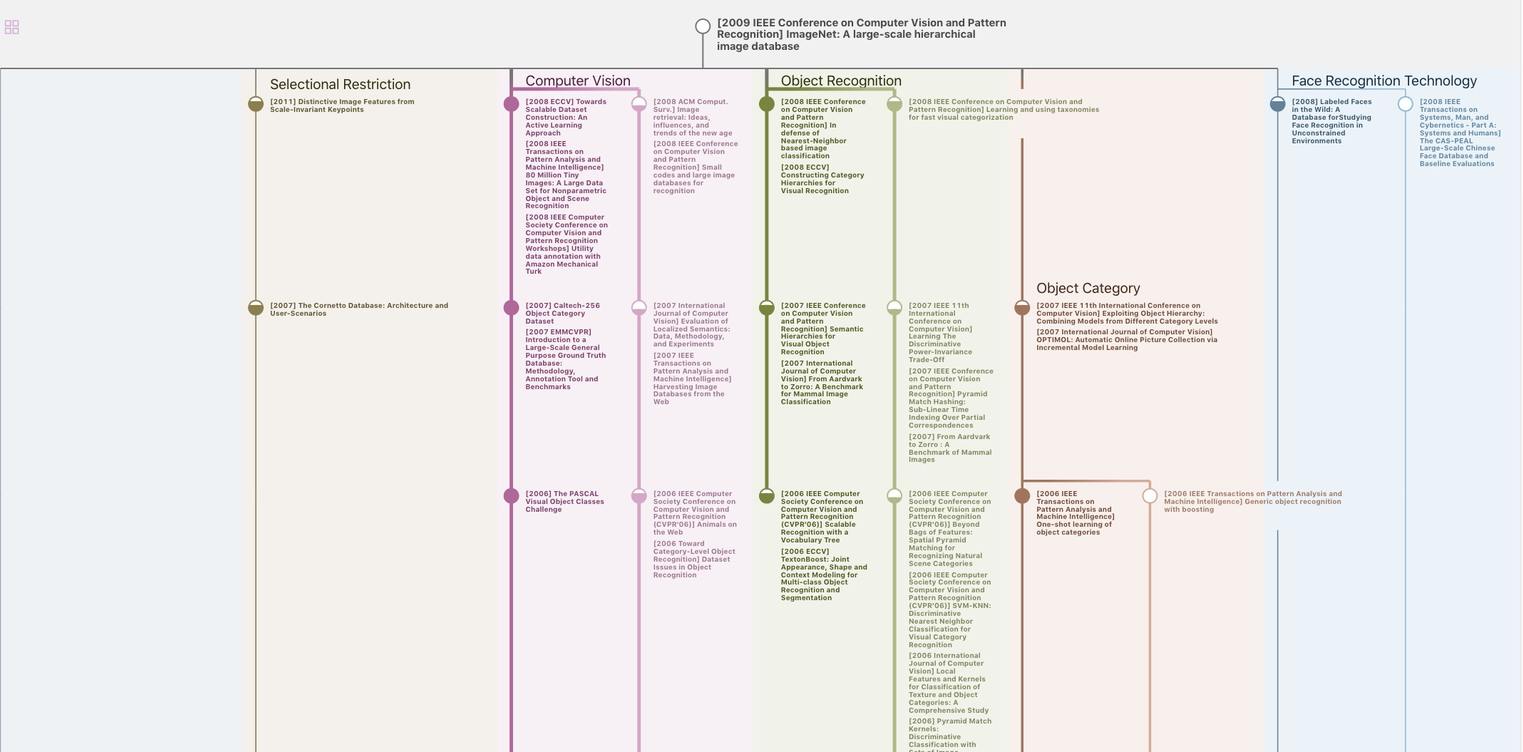
生成溯源树,研究论文发展脉络
Chat Paper
正在生成论文摘要