Tuple Bubbles: Learned Tuple Representations for Tunable Approximate Query Processing
aiDM@SIGMOD(2023)
摘要
We propose a versatile approach to lightweight, approximate query processing by learning compact but tunably precise representations of larger quantities of original tuples, coined bubbles. Instead of working with tables of tuples, the query processing operates on bubbles but leaves the traditional query processing paradigms conceptually applicable. We believe this is a natural and viable approach to render approximate query processing feasible for large data in disaggregated cloud settings or resource-limited scenarios. Bubbles are tunable regarding the compactness of enclosed tuples as well as the granularity of statistics and the way they are instantiated. We present a first working solution that models bubble contents using Bayesian networks and unsupervised deep learning models, respectively, and discuss the pros and cons of these models. In an experimental evaluation, we demonstrate the performance benefits and limitations of the proposed approaches against state-of-the-art competitors over synthetic and real-world datasets.
更多查看译文
AI 理解论文
溯源树
样例
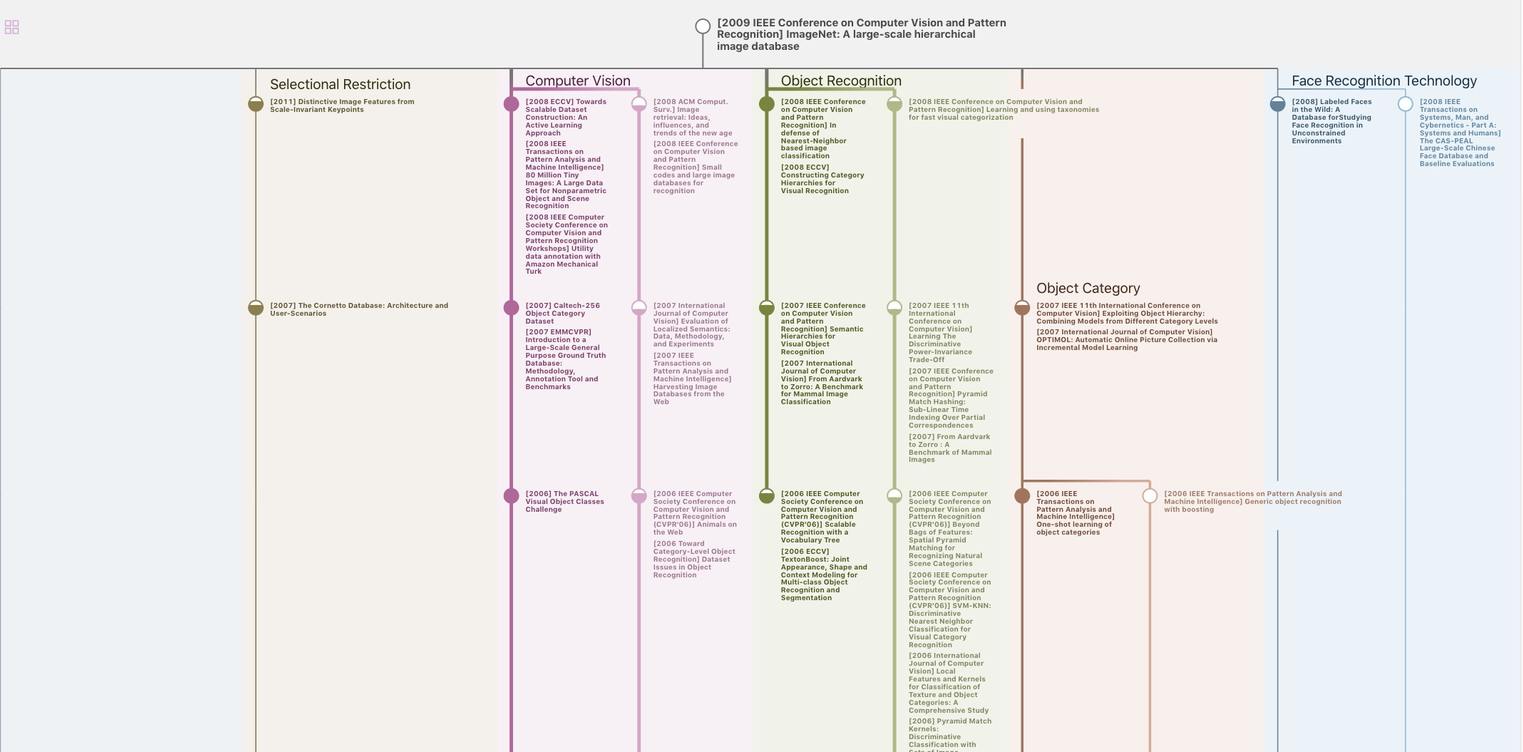
生成溯源树,研究论文发展脉络
Chat Paper
正在生成论文摘要