Discrete-time Contraction Constrained Nonlinear Model Predictive Control Using Graph-based Geodesic Computation
AIChE journal(2022)
摘要
Modern chemical processes need to operate around time-varying operating conditions to optimize plant economy, in response to dynamic supply chains (e.g., time-varying specifications of product and energy costs). As such, the process control system needs to handle a wide range of operating conditions whilst optimizing system performance and ensuring stability during transitions. This article presents a reference-flexible nonlinear model predictive control approach using contraction based constraints. Firstly, a contraction condition that ensures convergence to any feasible state trajectories or setpoints is constructed. This condition is then imposed as a constraint on the optimization problem for model predictive control with a general (typically economic) cost function, utilizing Riemannian weighted graphs and shortest path techniques. The result is a reference flexible and fast optimal controller that can trade-off between the rate of target trajectory convergence and economic benefit (away from the desired process objective). The proposed approach is illustrated by a simulation study on a CSTR control problem.
更多查看译文
关键词
contraction theory,discrete-time nonlinear systems,graph theory,nonlinear model predictive control,stability design
AI 理解论文
溯源树
样例
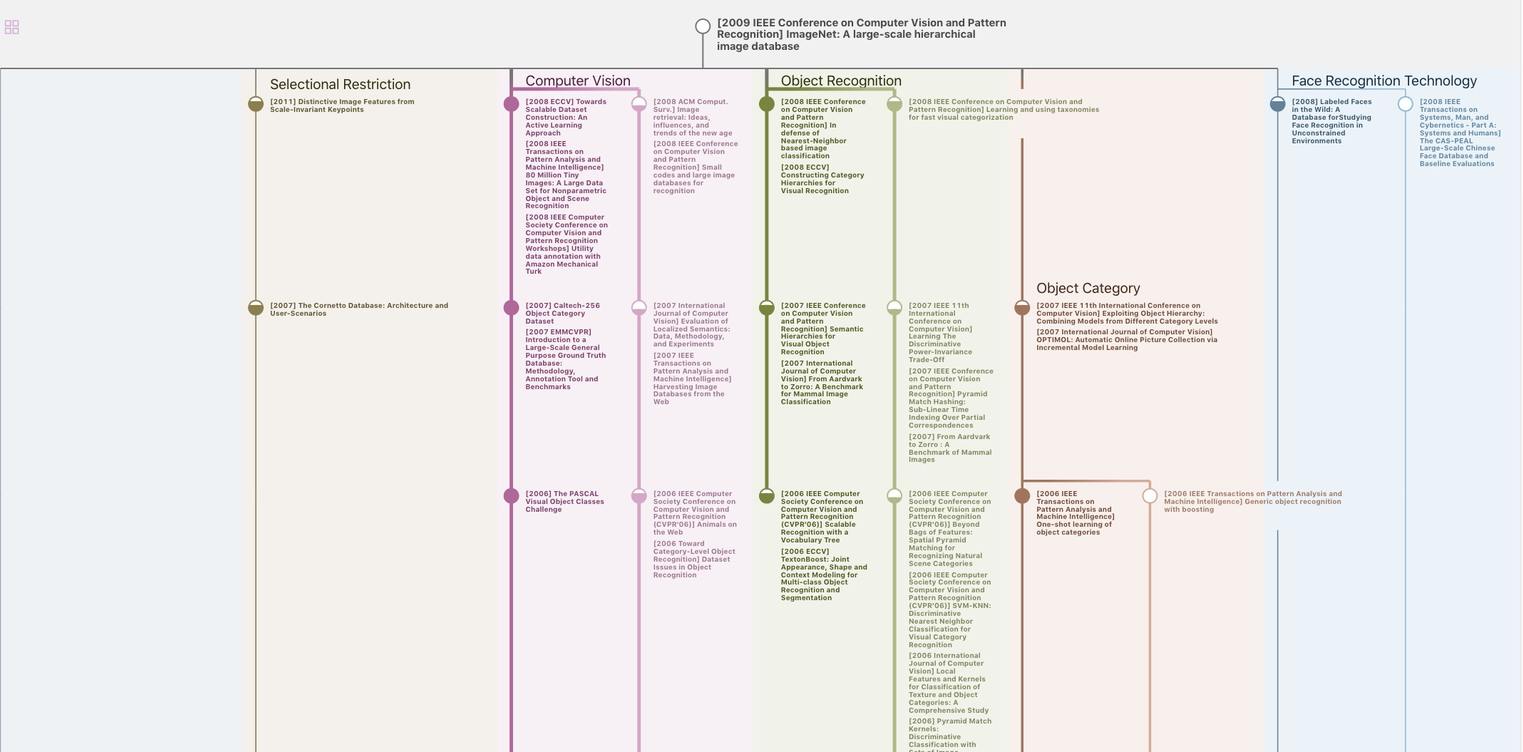
生成溯源树,研究论文发展脉络
Chat Paper
正在生成论文摘要