Flow field reconstruction and shock train leading edge position detection of scramjet isolation section based on a small amount of CFD data
Research Square (Research Square)(2022)
摘要
Abstract In scramjet, the position of shock train is affected by the change of back pressure. When the leading edge of the shock train is pushed out from the inlet of the isolation section, the engine will not start. Therefore, it is very important to detect the flow field structure in the isolation section and the leading edge position of the shock train. The traditional shock train detection method has low detection accuracy and slow detection speed. This paper describes a method based on deep learning to reconstruct the flow field in the isolation section and detect the leading edge of the shock train. Under various back pressure conditions, the flow field images of computational fluid dynamics (CFD) data and the corresponding upper and lower wall pressure data were obtained, and a data set corresponding to pressure and flow field was constructed. By constructing and training convolution neural network, a mapping model with pressure information as input and flow field image as output is obtained, and then the leading edge position of shock train is detected on the output flow field image. The experimental results show that the average structure similarity (SSIM) between the reconstructed flow field image and the CFD flow field image is 0.902, the average peak signal-to-noise ratio (PSNR) is 25.289, the average correlation coefficient (CORR) is 0.956, and the root mean square error of shock train leading edge detection is 3.28mm. Finally, in order to improve the detection accuracy of the leading edge position, we fine tuned the model and obtained another detection method, which reduced the root mean square error of the detection results to 1.87mm.
更多查看译文
关键词
scramjet isolation section,flow field reconstruction,edge position detection,shock train
AI 理解论文
溯源树
样例
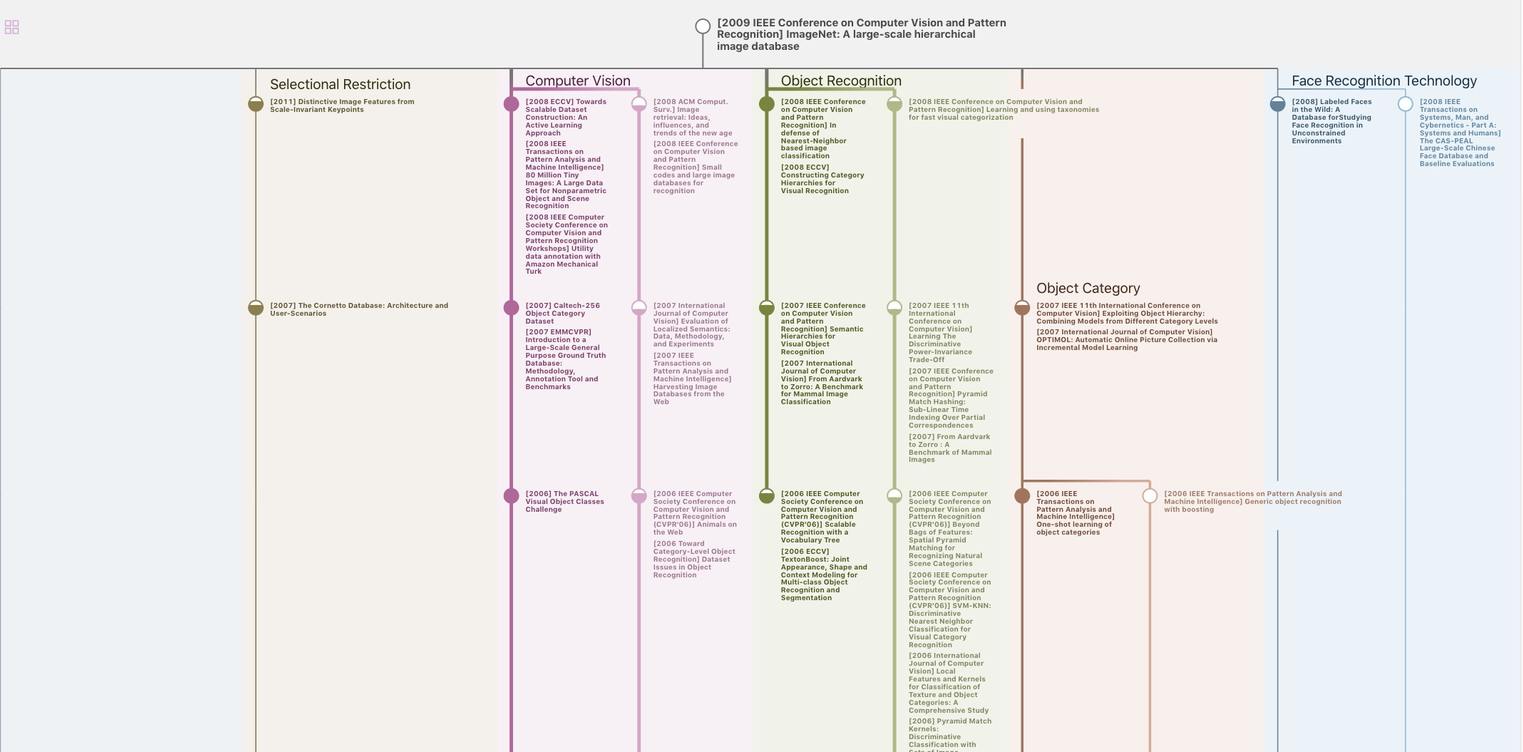
生成溯源树,研究论文发展脉络
Chat Paper
正在生成论文摘要