Network Balance Scaling Improves Performance and Explainability of Machine Learning Models in Early Drug Discovery
crossref(2022)
Abstract
Abstract Explainable and accurate predictions of bioactivity values are indispensable to accelerate drug design and reduce attrition during drug development. To enhance the accuracy of predictions, a new algorithmic approach was designed, which unites the advantages of matched molecular series and supervised machine learning (ML) techniques. This approach named Network Balance Scaling (NBS) employs convex optimization on matched molecular series networks enriched with ML data. By applying NBS, the performance of supervised ML methods can be improved, and predictions can be rationalized following the network of similar compounds. The approach was validated on the freely available MoleculeNet benchmark with respective ML models as well as on real-world targets and corresponding ML models provided by Merck KGaA. In all cases, when combining NBS with supervised ML models, we observe a substantial improvement in the different activity and physicochemical data sets and bioactivity-related application scenarios. The open-source code of NBS can be found freely available at https://github.com/rareylab/NetworkBalanceScaling.
MoreTranslated text
AI Read Science
Must-Reading Tree
Example
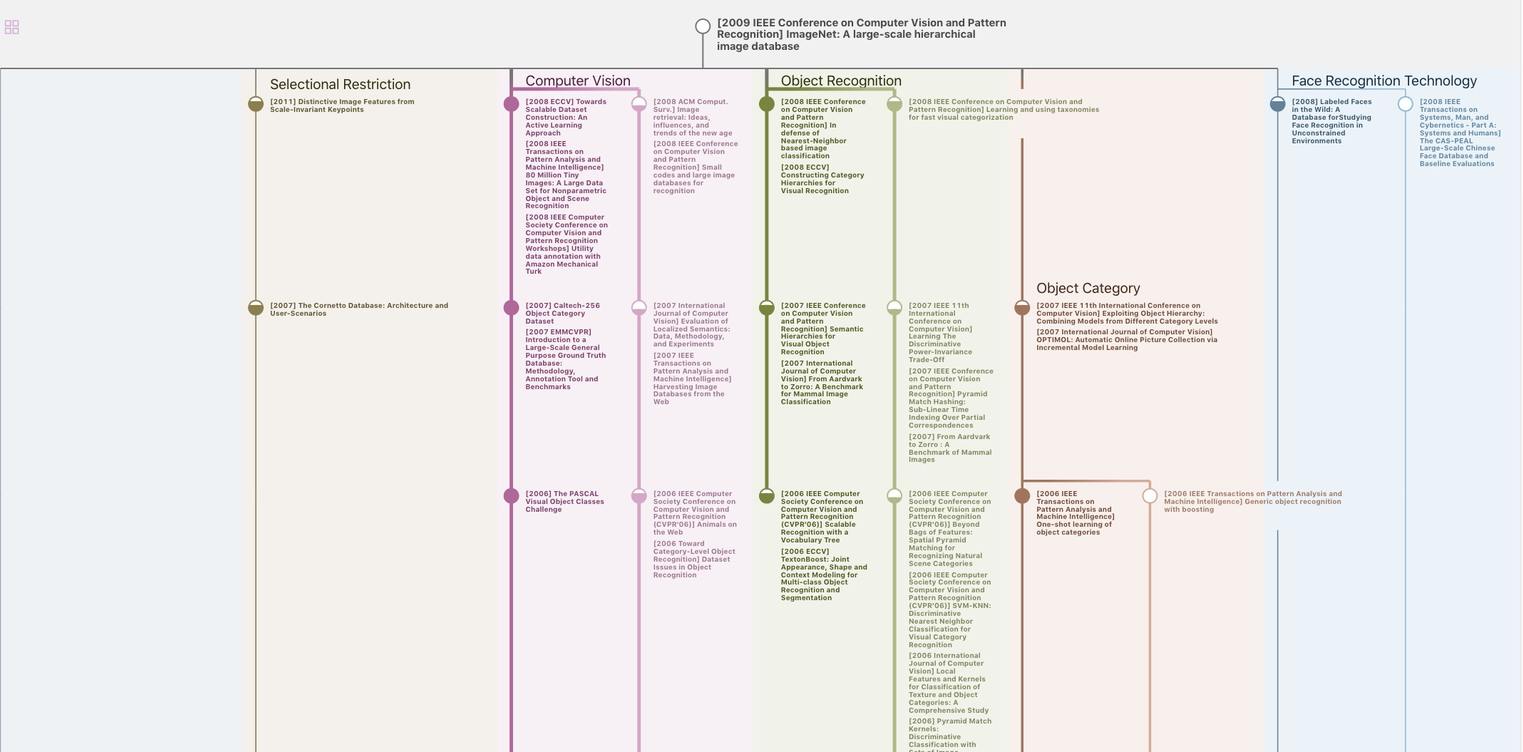
Generate MRT to find the research sequence of this paper
Chat Paper
Summary is being generated by the instructions you defined