An Intelligent Method for Tbm Surrounding Rock Classification Based on Time Series Segmentation of Rock-Machine Interaction Data
Tunnelling and underground space technology(2023)
摘要
Accurate, continuous real-time perception of surrounding rock conditions on the tunnel boring machine (TBM) tunnel face provides particularly important prior information to ensure safe, economic, and efficient tunneling. This study presents an intelligent method for assessing the TBM surrounding rock conditions using a combination of time series segmentation and a deep clustering model (SSAE-FCM). In data preprocessing, fifteen operational parameters were selected as input features. And an adaptive piecewise constant approximation method was employed for the segmentation and compression of stable phase data. Then, a total of 69,214 data samples that can characterize the stable rock-machine interaction were obtained to construct a dataset. Thereafter, the SSAEFCM model was established and trained to classify the surrounding rock conditions into four clusters. The distribution result of the main operational parameters and composite indexes (TPI, FPI, and SE) within these clusters demonstrated that the classification results are compatible with rock-machine interaction in engineering practice. Additionally, the rock mass assessment performance of the classification results was compared to BQ classification in terms of various rock mass parameters (UCS, Jv), fault sections, and collapse sections. These results indicate that the proposed classification method accurately reflects the stability and boreability of rock mass and is more sensitive to unfavorable geology than the BQ classification. From the three clustering evaluation indexes (SC, CHI, and DBI) perspective, SSAE-FCM was superior to the other clustering models (BIRCH + kmeans++, FCM, and SSAE + FCM). In conclusion, the proposed intelligent method for TBM surrounding rock classification is reasonable and applicable for the evaluation of rock mass quality, as well as accurate and sensitive in real-time perception.
更多查看译文
关键词
Tunnel boring machine,Classification of rock mass quality,Perception of surrounding rock conditions,Time series segmentation,Deep clustering
AI 理解论文
溯源树
样例
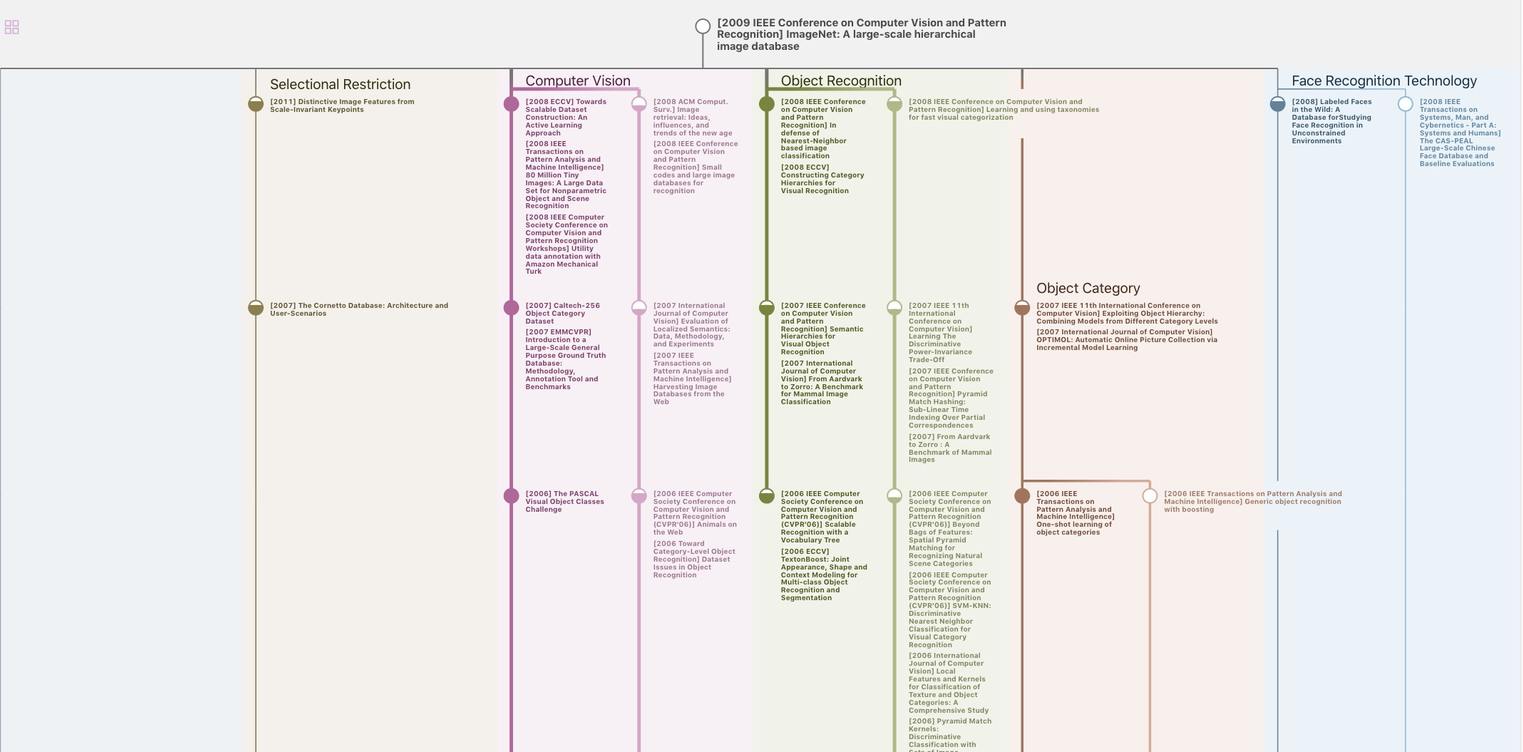
生成溯源树,研究论文发展脉络
Chat Paper
正在生成论文摘要