Cardynet: deep learning based navigation for car-like robots in dynamic environments
PROCEEDINGS OF ASME 2022 INTERNATIONAL MECHANICAL ENGINEERING CONGRESS AND EXPOSITION, IMECE2022, VOL 5(2022)
摘要
Recently, there has been an increased interest in learning based navigation solutions for robots operating in dynamic environments. Navigation in such environments is particularly challenging due to several reasons. The decisions made by other agents may only be partially observable to the robot, and they may have conflicting objectives. The robot may have imperfect or no knowledge of other agents' goals and planned routes. Several of the recent studies focus on differential drive robots. However, unlike the differential drive, car-like robots are subjected to additional motion constraints that make the problem harder. In this work, we first utilize an optimization based planner, namely TEB local planner, as the expert to generate high quality motion commands for a car-like robot operating in a simulated dynamic environment. We utilize these labels to train a deep neural network that learns to navigate. The deep learning based planner is further augmented with safety margins to enhance its effectiveness in collision avoidance. Finally, we experimentally evaluated the performance of our proposed method by comparing it with deployable TEB planner based local planners in the stage_ros simulator as well as real world experiments. Our method demonstrated superior performance in terms of obstacle avoidance as well as successful mission completion.
更多查看译文
关键词
Autonomous Navigation,Car-like Robots,Supervised Learning,Collision Avoidance
AI 理解论文
溯源树
样例
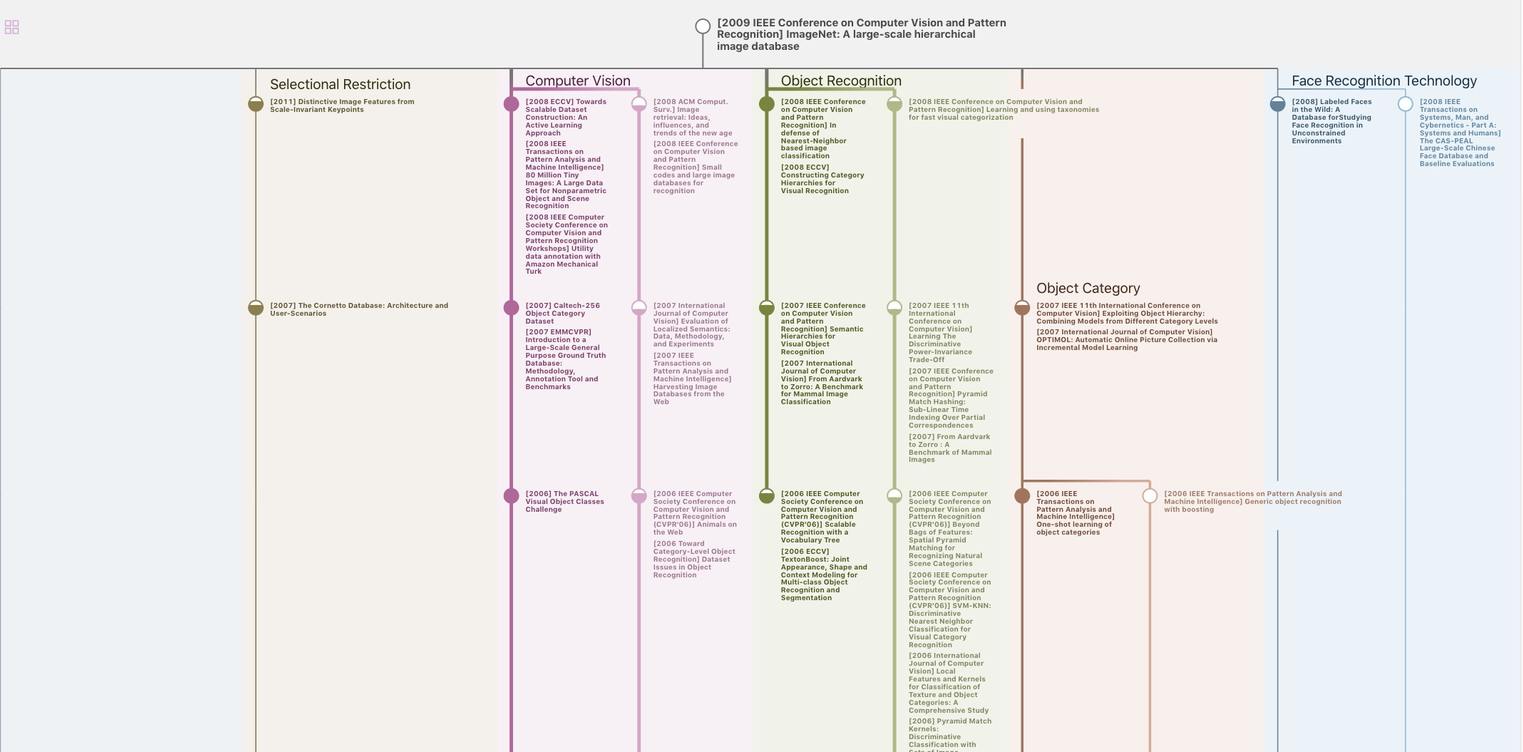
生成溯源树,研究论文发展脉络
Chat Paper
正在生成论文摘要