Can we use a biomarker detection algorithm to measure the effectiveness of 14-channel neurofeedback in dyslexia?
crossref(2022)
摘要
The most common neurological diversity that children experience is dyslexia and it manifests itself in reduced reading ability. There is a genetic predisposition for dyslexia, and more recent theories explain it as a disruption in left hemispheric lateralization that reduces effective reading and writing. A software for smartphones called Auto Train Brain helps children with dyslexia to improve their reading comprehension and reading speed. Measuring the efficacy of the mobile app training was done manually with psychometric tests beforehand and we use a biomarker detection software to measure the efficacy of the neurofeedback. Machine learning (ML) techniques have recently been used to classify children with dyslexia and typically developing children (TDC). The data consists of 100 sessions of 2-minute resting-state eyes-open 14-channel Quantitative Electroencephalography (QEEG) data from 100 children with dyslexia and that of 100 TDC. We used the dyslexia biomarker detection software to assess the efficacy of 14-channel neurofeedback that was applied with Auto Train Brain. The results have shown that 30% of the sessions of children with dyslexia were classified as electrophysiologically normal, and 61% of the children with dyslexia were classified as electrophysiologically normal for at least 1 session after 20th sessions of neurofeedback.
更多查看译文
AI 理解论文
溯源树
样例
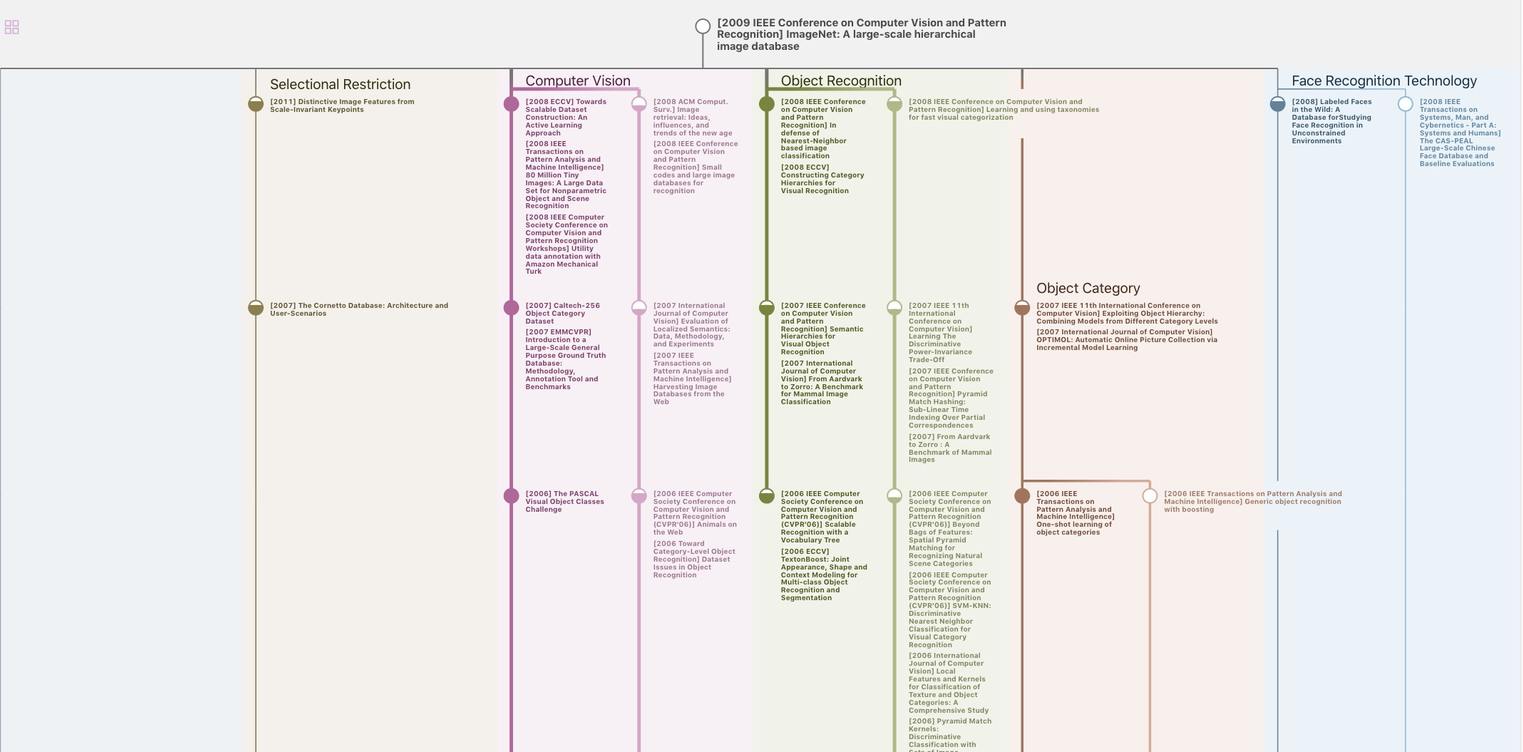
生成溯源树,研究论文发展脉络
Chat Paper
正在生成论文摘要