Unfavorable geology recognition in front of shallow tunnel face using machine learning
COMPUTERS AND GEOTECHNICS(2023)
摘要
Subsoil profile mapping is typically based on spatially discrete borehole logs obtained from site geotechnical investigations. During the mapping, soil information between two boreholes is indeed unknown and must be interpolated. The interpolation is not always able to identify geological anomalies, posing additional risks to tunneling. To supplement this need, this study develops a time-efficient pattern recognition framework for detecting potential geological anomalies ahead of the tunnel face during the advancement of Tunnel Boring Machine (TBM). Three basic unfavorable geology scenarios are defined, including a soil type change with varying inclination, an interlayer, and a boulder. A series of three-dimensional finite element shallow tunneling models are built to generate synthetic spatial and temporal data of ground settlements and pore water pressures during tunneling with respect to the three basic scenarios. Three machine learning methods, i.e., random forest (RF), support vector machine (SVM), and artificial neural network (ANN) are developed to recognize the geological anomalies using inputs comprised of the synthetic data combined with Gaussian noise that is added to enhance regularization and model robustness. The result shows that the proposed pattern recognition approach exhibits a powerful capability in classifying the unfavorable geology as early as 4.5-8D (D represents tunnel diameter) ahead of the target anomalies. Finally, the efficiency and effectiveness of the proposed pattern recognition framework are demonstrated in a real tunneling project. This study is of value in the mitigation of tunneling risks.
更多查看译文
关键词
Mechanized tunneling,Pattern recognition,Machine learning,Unfavorable geology,Sensor arrangement
AI 理解论文
溯源树
样例
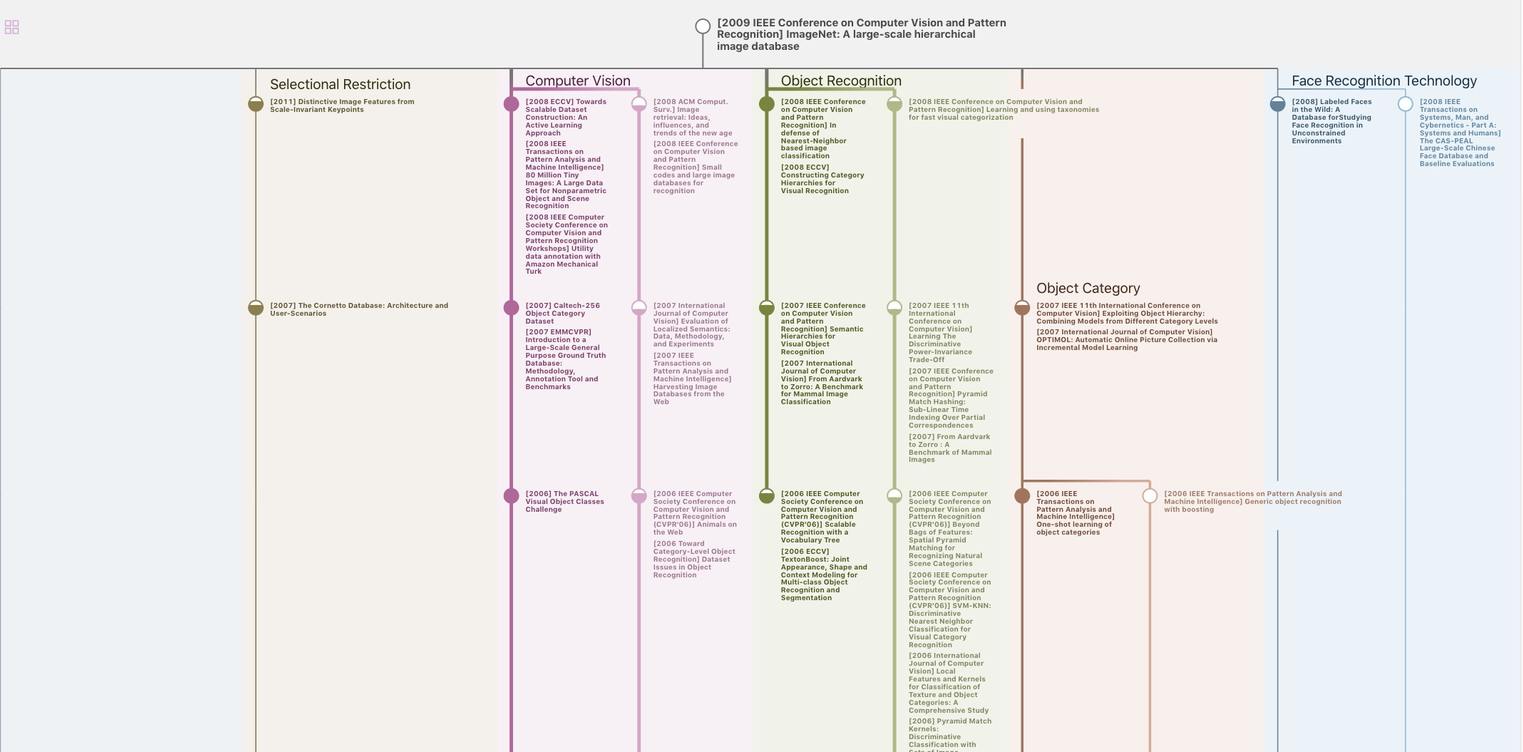
生成溯源树,研究论文发展脉络
Chat Paper
正在生成论文摘要