Integrated Decision Gradients: Compute Your Attributions Where the Model Makes Its Decision
AAAI 2024(2024)
摘要
Attribution algorithms are frequently employed to explain the decisions of neural network models. Integrated Gradients (IG) is an influential attribution method due to its strong axiomatic foundation. The algorithm is based on integrating the gradients along a path from a reference image to the input image. Unfortunately, it can be observed that gradients computed from regions where the output logit changes minimally along the path provide poor explanations for the model decision, which is called the saturation effect problem. In this paper, we propose an attribution algorithm called integrated decision gradients (IDG). The algorithm focuses on integrating gradients from the region of the path where the model makes its decision, i.e., the portion of the path where the output logit rapidly transitions from zero to its final value. This is practically realized by scaling each gradient by the derivative of the output logit with respect to the path. The algorithm thereby provides a principled solution to the saturation problem. Additionally, we minimize the errors within the Riemann sum approximation of the path integral by utilizing non-uniform subdivisions determined by adaptive sampling. In the evaluation on ImageNet, it is demonstrated that IDG outperforms IG, Left-IG, Guided IG, and adversarial gradient integration both qualitatively and quantitatively using standard insertion and deletion metrics across three common models.
更多查看译文
关键词
CV: Interpretability, Explainability, and Transparency,ML: Transparent, Interpretable, Explainable ML,PEAI: Accountability, Interpretability & Explainability
AI 理解论文
溯源树
样例
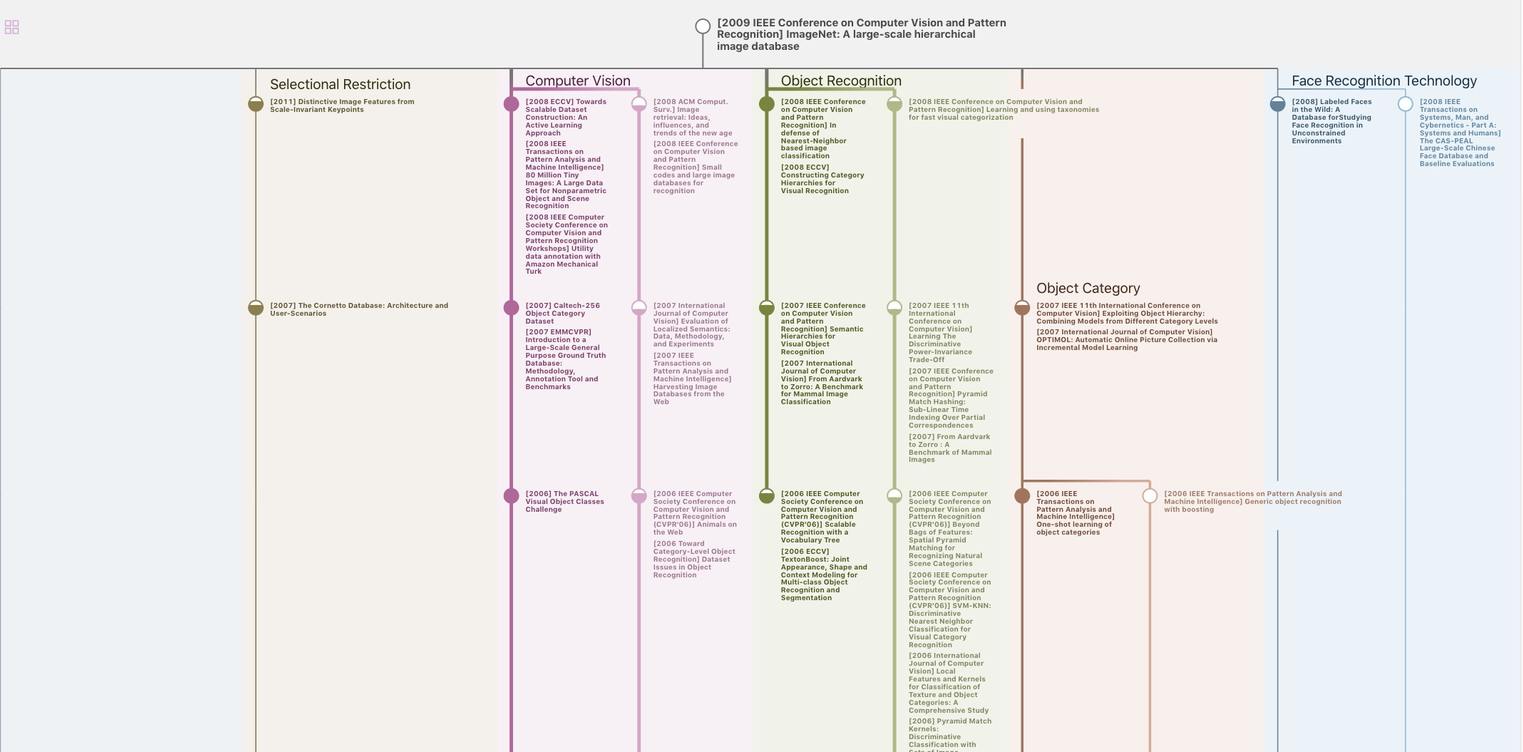
生成溯源树,研究论文发展脉络
Chat Paper
正在生成论文摘要