Entailment as Robust Self-Learner
PROCEEDINGS OF THE 61ST ANNUAL MEETING OF THE ASSOCIATION FOR COMPUTATIONAL LINGUISTICS (ACL 2023): LONG PAPERS, VOL 1(2023)
摘要
Entailment has been recognized as an important metric for evaluating natural language understanding (NLU) models, and recent studies have found that entailment pretraining benefits weakly supervised fine-tuning. In this work, we design a prompting strategy that formulates a number of different NLU tasks as contextual entailment. This approach improves the zero-shot adaptation of pretrained entailment models. Secondly, we notice that self-training entailment-based models with unlabeled data can significantly improve the adaptation performance on downstream tasks. To achieve more stable improvement, we propose the Simple Pseudo-Label Editing (SimPLE) algorithm for better pseudo-labeling quality in self-training. We also found that both pretrained entailment-based models and the self-trained models are robust against adversarial evaluation data. Experiments on binary and multi-class classification tasks show that SimPLE leads to more robust self-training results, indicating that the self-trained entailment models are more efficient and trustworthy than large language models on language understanding tasks.
更多查看译文
关键词
Language Modeling,Topic Modeling,Natural Language Processing,Sequence-to-Sequence Learning,Semantic Simplification
AI 理解论文
溯源树
样例
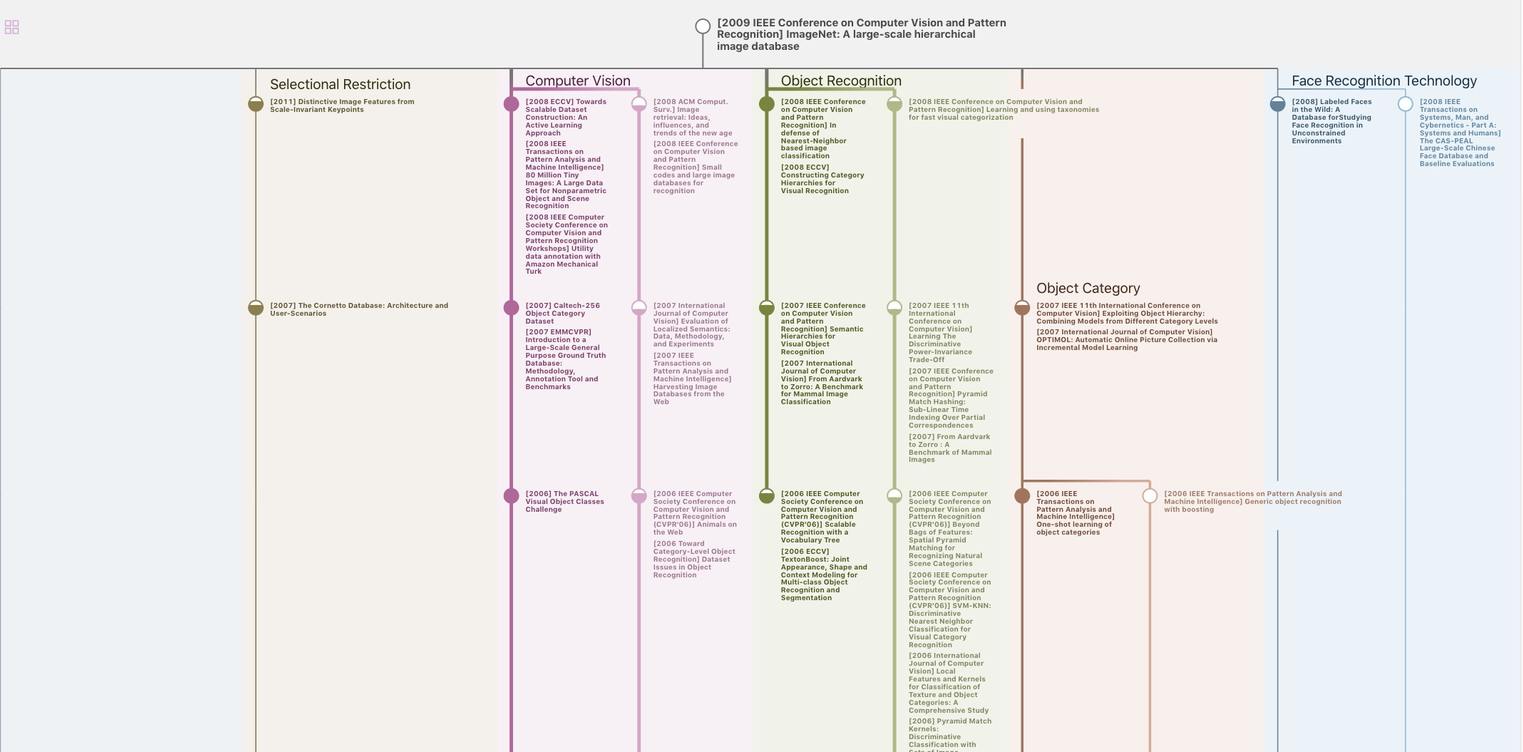
生成溯源树,研究论文发展脉络
Chat Paper
正在生成论文摘要