CoRaL: Continual Representation Learning for Overcoming Catastrophic Forgetting
AAMAS '23: Proceedings of the 2023 International Conference on Autonomous Agents and Multiagent Systems(2023)
Abstract
Humans have the ability to acquire, retain and transfer knowledge over their lifespan. For intelligent agents to achieve fluent longitudinal interaction, they need to continually retain, refine and acquire new knowledge. However, current learning approaches, in particular Deep Neural Networks, are prone to catastrophic forgetting, a phenomenon where the network forgets its past representation as the data distribution changes. To address this challenge, in this work, we propose CoRaL, a novel continual learning framework that considers the past response of the network when learning a new task. CoRaL comprises a Representation Learning module that learns representations that are robust to distribution shifts and a Knowledge Distillation module that encourages the network to retain past knowledge. The Representation Learning module is a Siamese Network setup that maximizes the similarity between two augmented versions of the input. The Knowledge Distillation module buffers past inputs and penalizes divergence between past and current network output. We evaluated CoRaL on three challenging Continual Learning scenarios across four datasets. The results suggest that CoRaL outperformed all evaluated state-of-the-art methods, achieving the highest accuracy and lowest forgetting. Finally, we conducted extensive ablation studies to highlight the importance of the proposed modules in addressing catastrophic forgetting.
MoreTranslated text
AI Read Science
Must-Reading Tree
Example
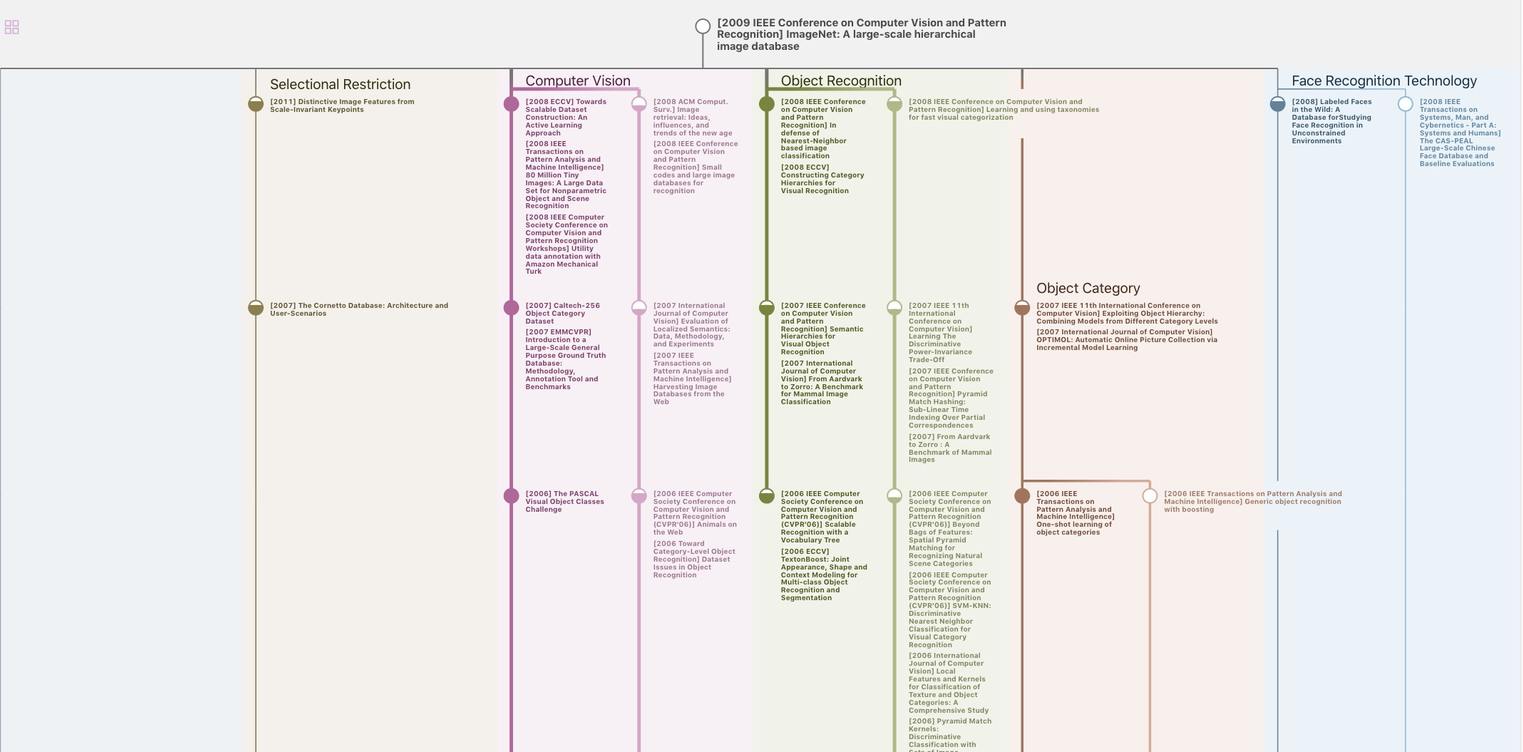
Generate MRT to find the research sequence of this paper
Chat Paper
Summary is being generated by the instructions you defined