2-Bit Conformer Quantization for Automatic Speech Recognition
INTERSPEECH 2023(2023)
Abstract
Large speech models are rapidly gaining traction in research community. As a result, model compression has become an important topic, so that these models can fit in memory and be served with reduced cost. Practical approaches for compressing automatic speech recognition (ASR) model use int8 or int4 weight quantization. In this study, we propose to develop 2-bit ASR models. We explore the impact of symmetric and asymmetric quantization combined with sub-channel quantization and clipping on both LibriSpeech dataset and large-scale training data. We obtain a lossless 2-bit Conformer model with 32% model size reduction when compared to state of the art 4-bit Conformer model for LibriSpeech. With the large-scale training data, we obtain a 2-bit Conformer model with over 40% model size reduction against the 4-bit version at the cost of 17% relative word error rate degradation
MoreTranslated text
Key words
speech recognition,model quantization,low-bit quantization
PDF
View via Publisher
AI Read Science
Must-Reading Tree
Example
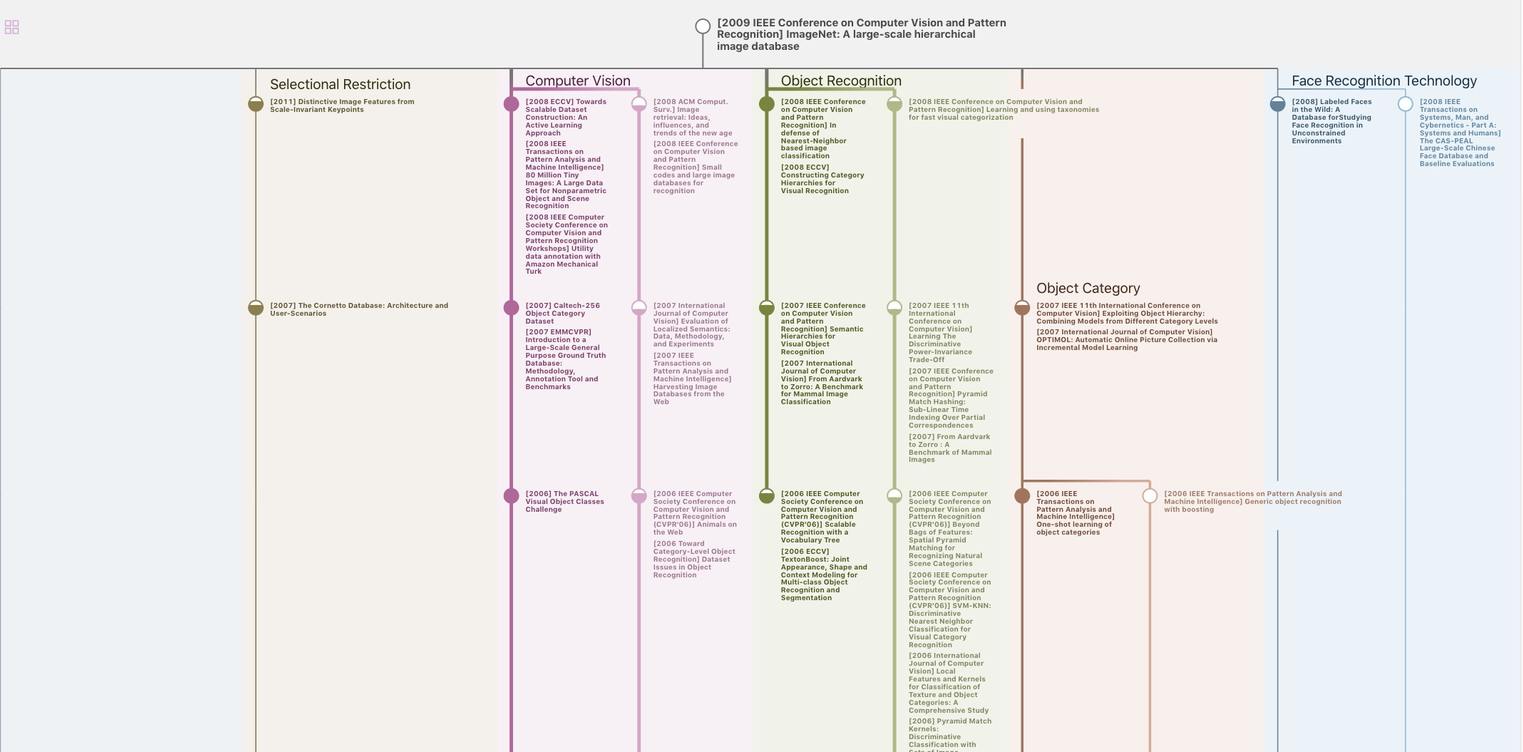
Generate MRT to find the research sequence of this paper
Related Papers
2016
被引用11619 | 浏览
2015
被引用4005 | 浏览
2016
被引用2430 | 浏览
2017
被引用8 | 浏览
A Streaming On-Device End-to-End Model Surpassing Server-Side Conventional Model Quality and Latency
2020
被引用239 | 浏览
2020
被引用68 | 浏览
2020
被引用35 | 浏览
2023
被引用1 | 浏览
Data Disclaimer
The page data are from open Internet sources, cooperative publishers and automatic analysis results through AI technology. We do not make any commitments and guarantees for the validity, accuracy, correctness, reliability, completeness and timeliness of the page data. If you have any questions, please contact us by email: report@aminer.cn
Chat Paper
去 AI 文献库 对话