Optimization and Interpretability of Graph Attention Networks for Small Sparse Graph Structures in Automotive Applications
CoRR(2023)
摘要
For automotive applications, the Graph Attention Network (GAT) is a prominently used architecture to include relational information of a traffic scenario during feature embedding. As shown in this work, however, one of the most popular GAT realizations, namely GATv2, has potential pitfalls that hinder an optimal parameter learning. Especially for small and sparse graph structures a proper optimization is problematic. To surpass limitations, this work proposes architectural modifications of GATv2. In controlled experiments, it is shown that the proposed model adaptions improve prediction performance in a node-level regression task and make it more robust to parameter initialization. This work aims for a better understanding of the attention mechanism and analyzes its interpretability of identifying causal importance.
更多查看译文
关键词
graph attention networks,sparse graph structures
AI 理解论文
溯源树
样例
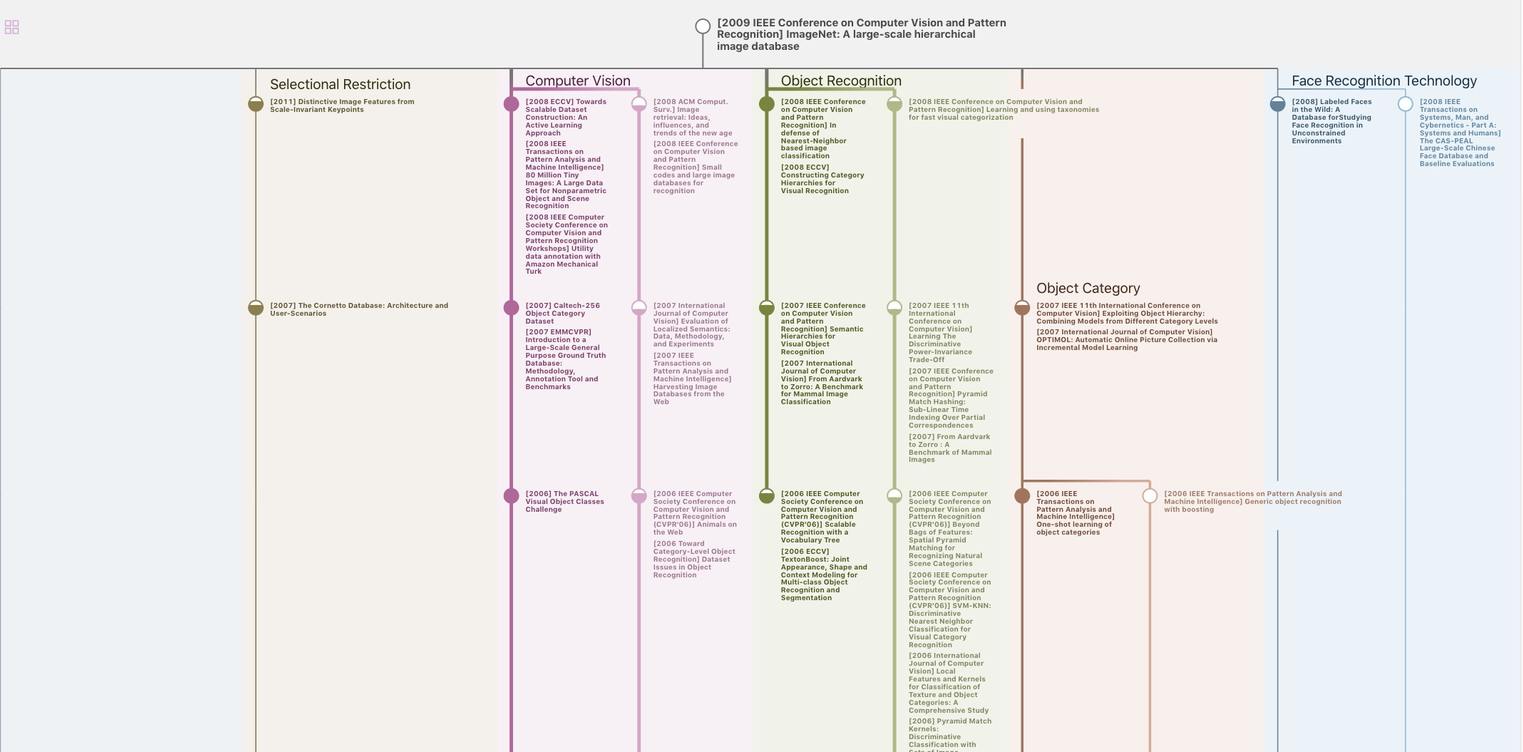
生成溯源树,研究论文发展脉络
Chat Paper
正在生成论文摘要