Musical Genre Recognition Based on Deep Descriptors of Harmony, Instrumentation, and Segments
Artificial Intelligence in Music, Sound, Art and Design(2023)
摘要
Deep learning has recently established itself as a cluster of methods of choice for almost all classification tasks in music information retrieval. However, despite very good classification performance, it sometimes brings disadvantages including long training times and higher energy costs, lower interpretability of classification models, or an increased risk of overfitting when applied to small training sets due to a very large number of trainable parameters. In this paper, we investigate the combination of both deep and shallow algorithms for recognition of musical genres using a transfer learning approach. We train deep classification models once to predict harmonic, instrumental, and segment properties from datasets with respective annotations. Their predictions for another dataset with annotated genres are used as features for shallow classification methods. They can be trained over and again for different categories, and are particularly useful when the training sets are small, in a real world scenario when listeners define various musical categories selecting only a few prototype tracks. The experiments show the potential of the proposed approach for genre recognition. In particular, when combined with evolutionary feature selection which identifies the most relevant deep feature dimensions, the classification errors became significantly lower in almost all cases, compared to a baseline based on MFCCs or results reported in the previous work.
更多查看译文
关键词
Musical genre recognition, Deep neural networks, Transfer learning, Interpretable features, Evolutionary feature selection
AI 理解论文
溯源树
样例
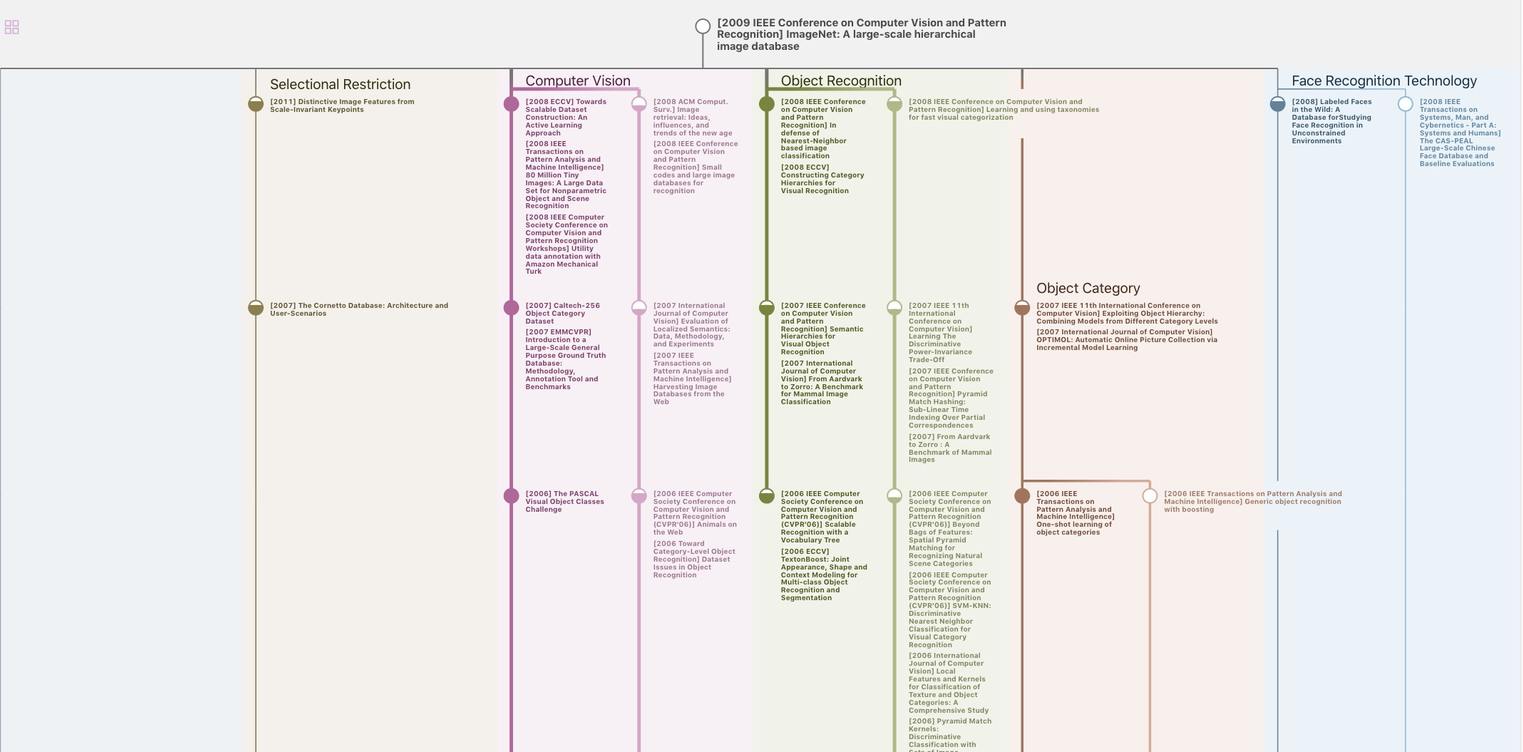
生成溯源树,研究论文发展脉络
Chat Paper
正在生成论文摘要