Active Continual Learning: On Balancing Knowledge Retention and Learnability
CoRR(2023)
摘要
Acquiring new knowledge without forgetting what has been learned in a
sequence of tasks is the central focus of continual learning (CL). While tasks
arrive sequentially, the training data are often prepared and annotated
independently, leading to the CL of incoming supervised learning tasks. This
paper considers the under-explored problem of active continual learning (ACL)
for a sequence of active learning (AL) tasks, where each incoming task includes
a pool of unlabelled data and an annotation budget. We investigate the
effectiveness and interplay between several AL and CL algorithms in the domain,
class and task-incremental scenarios. Our experiments reveal the trade-off
between two contrasting goals of not forgetting the old knowledge and the
ability to quickly learn new knowledge in CL and AL, respectively. While
conditioning the AL query strategy on the annotations collected for the
previous tasks leads to improved task performance on the domain and task
incremental learning, our proposed forgetting-learning profile suggests a gap
in balancing the effect of AL and CL for the class-incremental scenario.
更多查看译文
关键词
labelling queries,learning,tasks
AI 理解论文
溯源树
样例
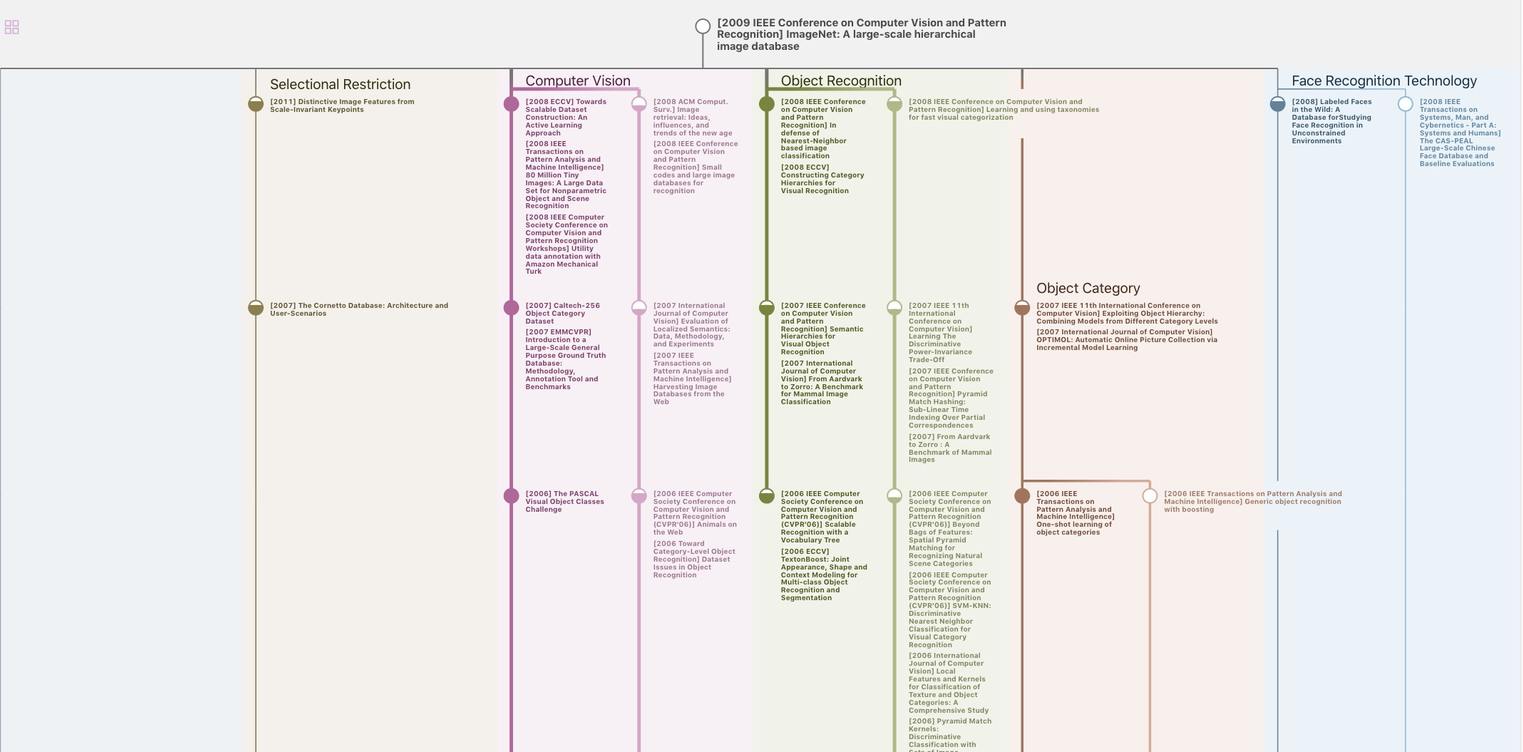
生成溯源树,研究论文发展脉络
Chat Paper
正在生成论文摘要