Collaborative Contrastive Learning for Hyperspectral and LiDAR Classification.
IEEE Trans. Geosci. Remote. Sens.(2023)
Abstract
Using single-source remote sensing (RS) data for classification of ground objects has certain limitations; however, multimodal RS data contain different types of features, such as spectral features and spatial features of hyperspectral image (HSI) and elevation information of light detection and ranging (LiDAR) data, which can be used to extract and fuse high-quality features to improve the classification accuracy. Nevertheless, the existing fusion techniques are mostly limited by the number of labeled samples due to the difficulty of label collection in the multimodal RS data. In this article, a fusion method of collaborative contrastive learning (CCL) is proposed to tackle the abovementioned issues for HSI and LiDAR data classification. The proposed CCL approach includes two stages of pretraining (CCL-PT) and fine-tuning (CCL-FT). In the CCL-PT stage, a collaborative strategy is introduced into contrastive learning (CL), which can extract features from HSI and LiDAR data separately and achieve the coordinated feature representation and matching between the two-modal RS data without labeled samples. In the CCL-FT stage, a multilevel fusion network is designed to optimize and fuse the unsupervised collaborative features, which are extracted in the CCL-PT stage for the classification tasks. Experimental results on three real-world datasets show that the developed CCL approach can perform excellently on the small sample classification tasks, and CL is feasible for the fusion of multimodal RS data.
MoreTranslated text
Key words
collaborative contrastive learning,hyperspectral,classification
AI Read Science
Must-Reading Tree
Example
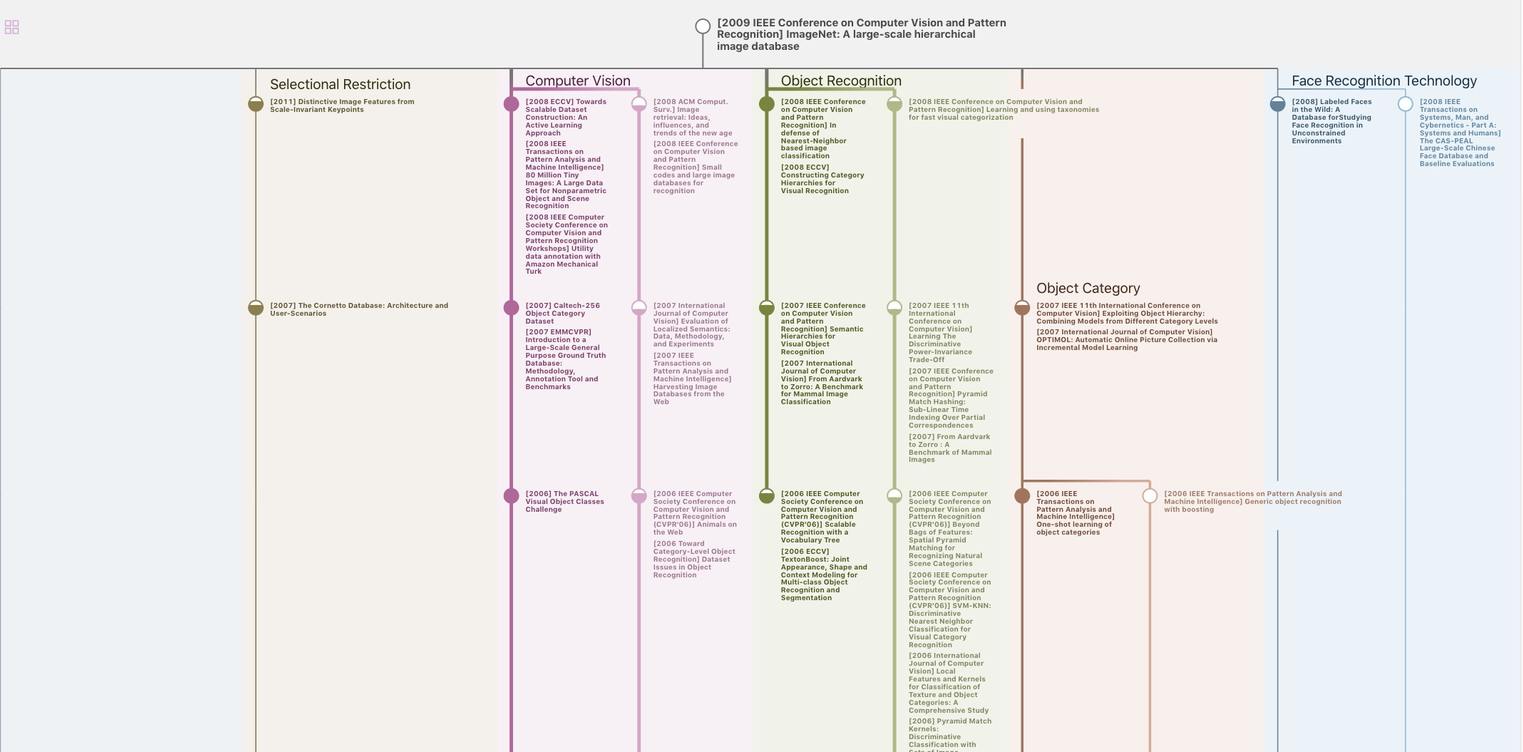
Generate MRT to find the research sequence of this paper
Chat Paper
Summary is being generated by the instructions you defined