Machine learning enables intelligent screening of interface materials towards minimizing voltage losses for p-i-n type perovskite solar cells
Journal of Energy Chemistry(2023)
摘要
Interface engineering is proved to be the most important strategy to push the device performance of the perovskite solar cell (PSC) to its limit, and numerous works have been conducted to screen efficient materials. Here, on the basis of the previous studies, we employ machine learning to map the relationship between the interface material and the device performance, leading to intelligently screening interface materials towards minimizing voltage losses in p-i-n type PSCs. To enhance the explainability of the machine learning models, molecular descriptors are used to represent the materials. Furthermore, experimental analysis with different characterization methods and device simulation based on the drift-diffusion physical model are conducted to get physical insights and validate the machine learning models. Accordingly, 3-thiophene ethylamine hydrochloride (ThEACl) is screened as an example, which enables remarkable improvements in VOC and PCE of the PSCs. Our work reveals the critical role of data-driven analysis in the high throughput screening of interface materials, which will significantly accelerate the exploration of new materials for high-efficiency PSCs.
更多查看译文
关键词
perovskite solar cells,solar cells,interface materials,machine learning
AI 理解论文
溯源树
样例
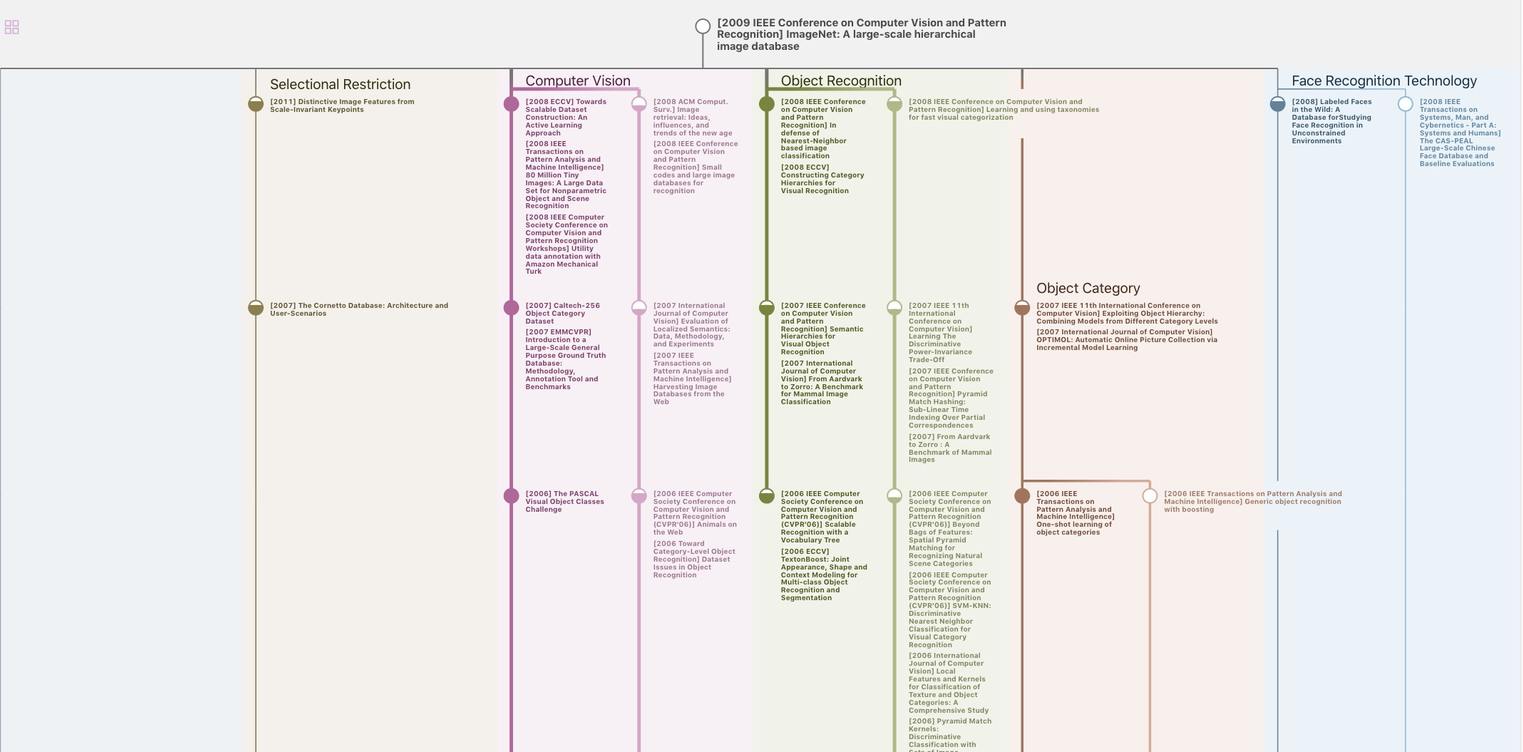
生成溯源树,研究论文发展脉络
Chat Paper
正在生成论文摘要