Farm3D: Learning Articulated 3D Animals by Distilling 2D Diffusion
2024 International Conference on 3D Vision (3DV)(2023)
摘要
We present Farm3D, a method to learn category-specific 3D reconstructors for articulated objects entirely from "free" virtual supervision from a pre-trained 2D diffusion-based image generator. Recent approaches can learn, given a collection of single-view images of an object category, a monocular network to predict the 3D shape, albedo, illumination and viewpoint of any object occurrence. We propose a framework using an image generator like Stable Diffusion to generate virtual training data for learning such a reconstruction network from scratch. Furthermore, we include the diffusion model as a score to further improve learning. The idea is to randomise some aspects of the reconstruction, such as viewpoint and illumination, generating synthetic views of the reconstructed 3D object, and have the 2D network assess the quality of the resulting image, providing feedback to the reconstructor. Different from work based on distillation which produces a single 3D asset for each textual prompt in hours, our approach produces a monocular reconstruction network that can output a controllable 3D asset from a given image, real or generated, in only seconds. Our network can be used for analysis, including monocular reconstruction, or for synthesis, generating articulated assets for real-time applications such as video games.
更多查看译文
关键词
3D,diffusion,self-supervised
AI 理解论文
溯源树
样例
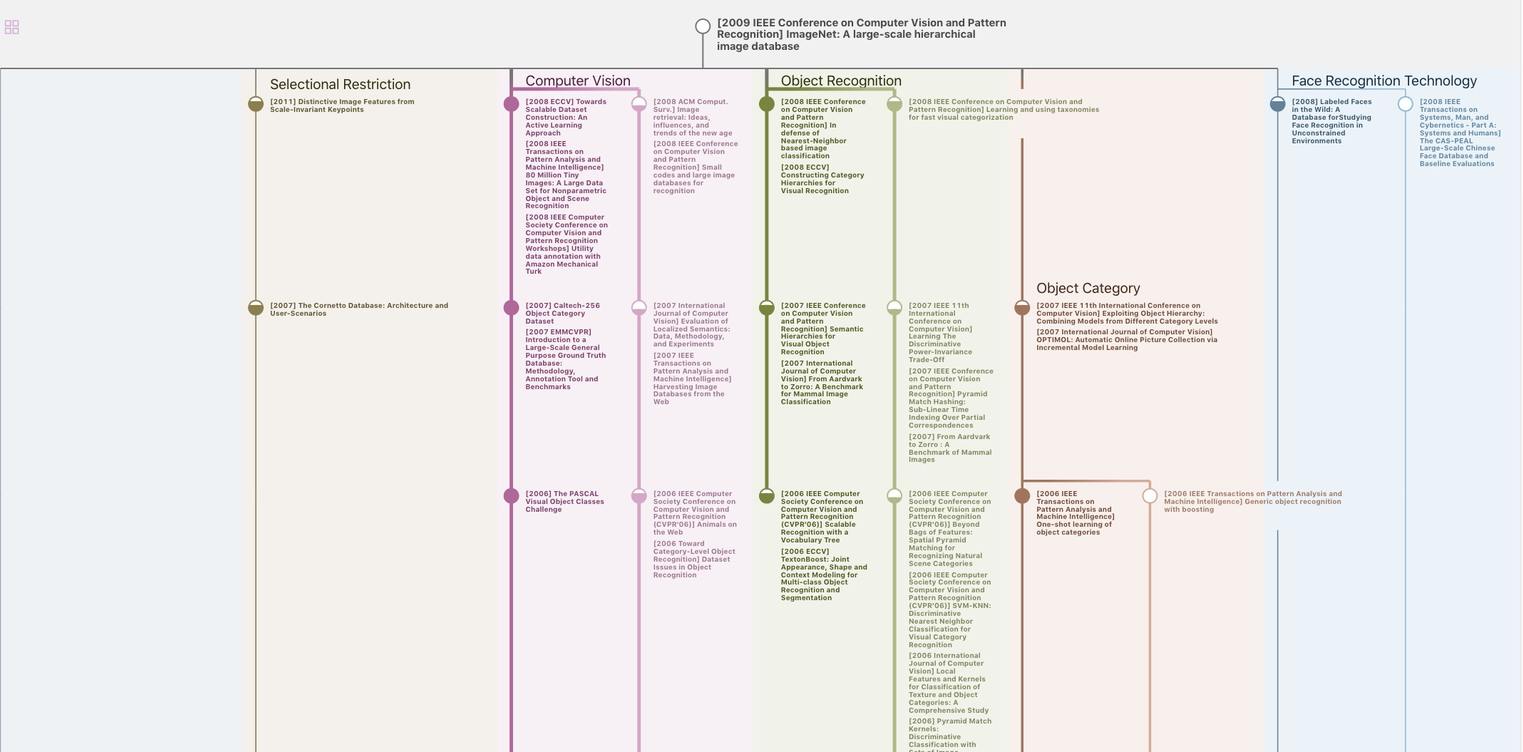
生成溯源树,研究论文发展脉络
Chat Paper
正在生成论文摘要