Leveraging Composition-Based Material Descriptors for Machine Learning Optimization
arXiv (Cornell University)(2023)
摘要
In this study, we evaluate several classifiers and focus on selecting a minimal set of appropriate material features. Our objective is to propose and discuss general strategies for reducing the number of descriptors required for material classification. The first strategy involves testing whether the critical temperature of the target material property is invariant with respect to binary groups of composition-based features. We also propose a multi-objective optimization procedure to reduce the set of composition-based material descriptors. The latter procedure is found to be particularly useful when applied to Bayesian classifiers. We test the proposed strategies focusing on low-temperature superconductors material data extracted from a public database.
更多查看译文
关键词
material descriptors,machine learning
AI 理解论文
溯源树
样例
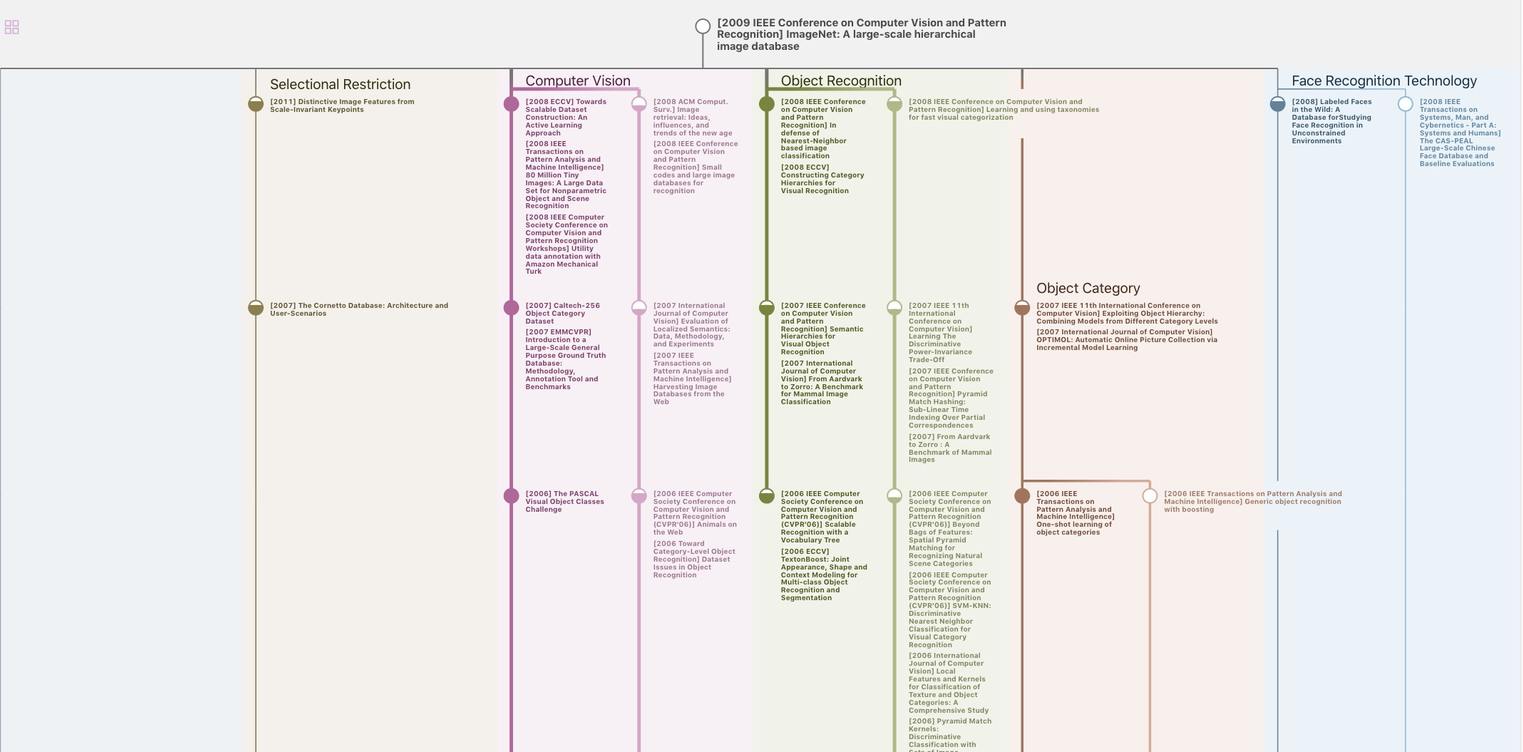
生成溯源树,研究论文发展脉络
Chat Paper
正在生成论文摘要