Automatic extraction of ranked SNP-phenotype associations from text using a BERT-LSTM-based method
BMC bioinformatics(2023)
摘要
Extraction of associations of singular nucleotide polymorphism (SNP) and phenotypes from biomedical literature is a vital task in BioNLP. Recently, some methods have been developed to extract mutation-diseases affiliations. However, no accessible method of extracting associations of SNP-phenotype from content considers their degree of certainty. In this paper, several machine learning methods were developed to extract ranked SNP-phenotype associations from biomedical abstracts and then were compared to each other. In addition, shallow machine learning methods, including random forest, logistic regression, and decision tree and two kernel-based methods like subtree and local context, a rule-based and a deep CNN-LSTM-based and two BERT-based methods were developed in this study to extract associations. Furthermore, the experiments indicated that although the used linguist features could be employed to implement a superior association extraction method outperforming the kernel-based counterparts, the used deep learning and BERT-based methods exhibited the best performance. However, the used PubMedBERT-LSTM outperformed the other developed methods among the used methods. Moreover, similar experiments were conducted to estimate the degree of certainty of the extracted association, which can be used to assess the strength of the reported association. The experiments revealed that our proposed PubMedBERT–CNN-LSTM method outperformed the sophisticated methods on the task.
更多查看译文
关键词
Biomedical relation extraction,Degree of certainty classification,Phenotype,SNP
AI 理解论文
溯源树
样例
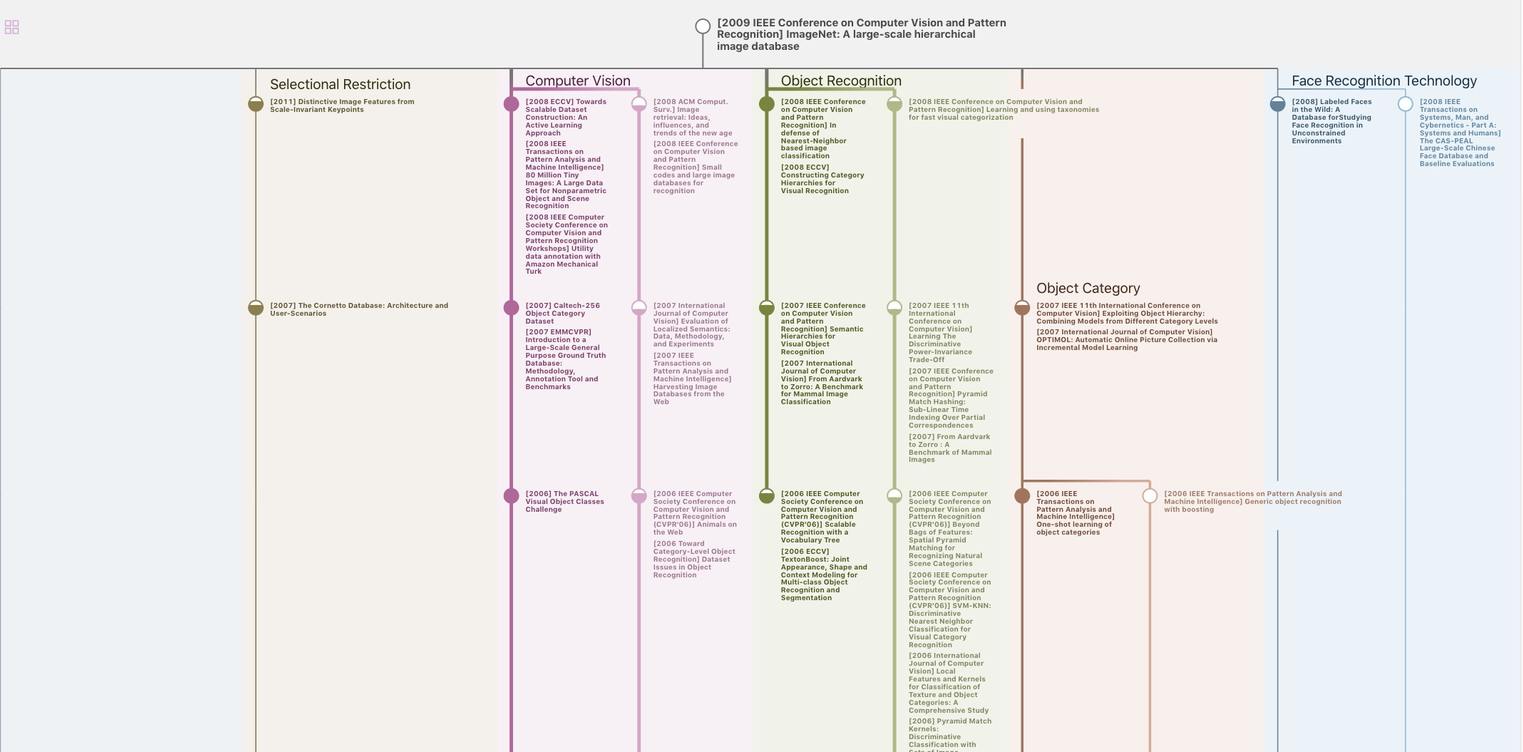
生成溯源树,研究论文发展脉络
Chat Paper
正在生成论文摘要