Learning Invariant Representation via Contrastive Feature Alignment for Clutter Robust SAR Target Recognition
CoRR(2023)
摘要
The deep neural networks (DNNs) have freed the synthetic aperture radar automatic target recognition (SAR ATR) from expertise-based feature designing and demonstrated superiority over conventional solutions. There has been shown the unique deficiency of ground vehicle benchmarks in shapes of strong background correlation results in DNNs overfitting the clutter and being non-robust to unfamiliar surroundings. However, the gap between fixed background model training and varying background application remains underexplored. Inspired by contrastive learning, this letter proposes a solution called Contrastive Feature Alignment (CFA) aiming to learn invariant representation for robust recognition. The proposed method contributes a mixed clutter variants generation strategy and a new inference branch equipped with channel-weighted mean square error (CWMSE) loss for invariant representation learning. In specific, the generation strategy is delicately designed to better attract clutter-sensitive deviation in feature space. The CWMSE loss is further devised to better contrast this deviation and align the deep features activated by the original images and corresponding clutter variants. The proposed CFA combines both classification and CWMSE losses to train the model jointly, which allows for the progressive learning of invariant target representation. Extensive evaluations on the MSTAR dataset and six DNN models prove the effectiveness of our proposal. The results demonstrated that the CFA-trained models are capable of recognizing targets among unfamiliar surroundings that are not included in the dataset, and are robust to varying signal-to-clutter ratios.
更多查看译文
关键词
contrastive feature alignment,learning invariant representation,recognition,target
AI 理解论文
溯源树
样例
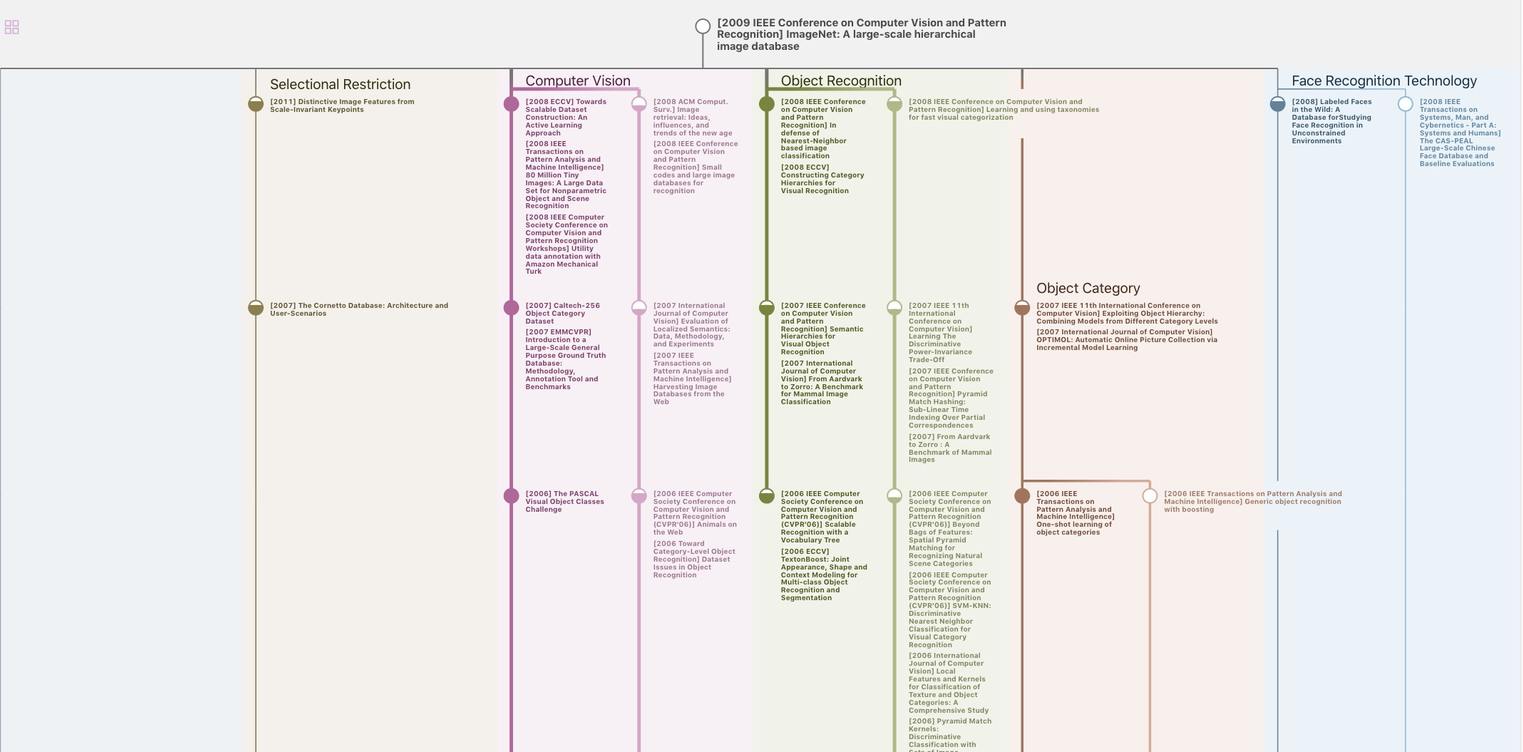
生成溯源树,研究论文发展脉络
Chat Paper
正在生成论文摘要