Active Learning-Driven Siamese Network for Hyperspectral Image Classification
REMOTE SENSING(2023)
Abstract
Hyperspectral image (HSI) classification has recently been successfully explored by using deep learning (DL) methods. However, DL models rely heavily on a large number of labeled samples, which are laborious to obtain. Therefore, finding a way to efficiently embed DL models in limited labeled samples is a hot topic in the field of HSI classification. In this paper, an active learning-based siamese network (ALSN) is proposed to solve the limited labeled samples problem in HSI classification. First, we designed a dual learning-based siamese network (DLSN), which consists of a contrastive learning module and a classification module. Secondly, in view of the problem that active learning is difficult to effectively sample under the extremely limited labeling cost, we proposed an adversarial uncertainty-based active learning (AUAL) method to query valuable samples, and to promote DLSN to learn a more complete feature distribution by fine-tuning. Finally, an active learning architecture, based on inter-class uncertainty (ICUAL), is proposed to construct a lightweight sample pair training set, fully extracting the inter-class information of sample pairs and improving classification accuracy. Experiments on three generic HSI datasets strongly demonstrated the effectiveness of ALSN for HSI classification, with performance improvements over other related DL methods.
MoreTranslated text
Key words
hyperspectral image (HSI) classification,limited labeled samples problem,active learning,siamese network
AI Read Science
Must-Reading Tree
Example
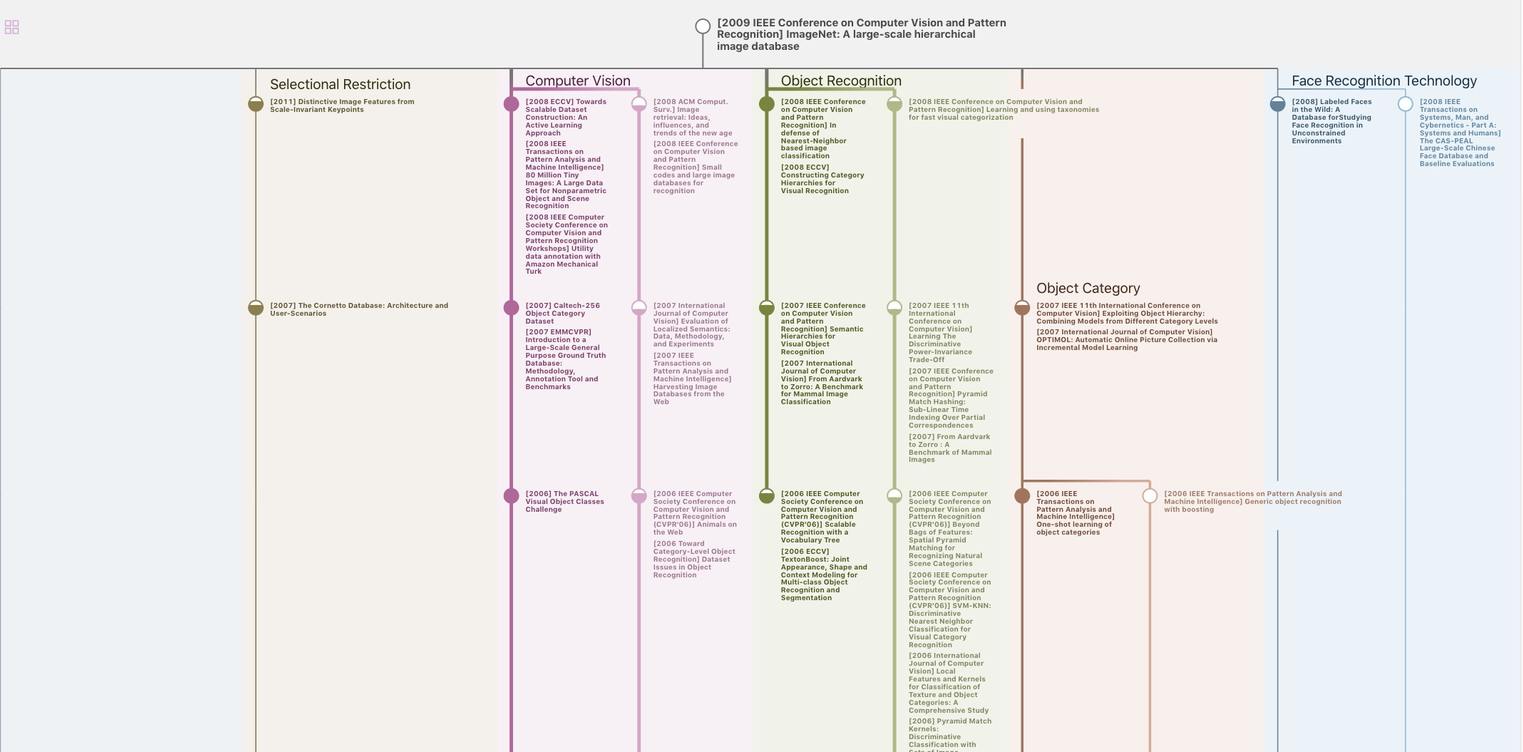
Generate MRT to find the research sequence of this paper
Chat Paper
Summary is being generated by the instructions you defined