DeoT: an End-to-end Encoder-Only Transformer Object Detector
Journal of real-time image processing(2023)
摘要
At present, with the rapid development of Transformer in object detection tasks, the object detection performance has been significantly improved. However, Transformer-based object detectors generally suffer from high complexity and slow learning convergence, and there is still a certain gap in performance compared to some convolutional neural network (CNN)-based object detectors. Therefore, to improve the existing problems of Transformer in object detection framework and make its detector performance reach the state-of-the-art level, this paper proposes an end-to-end encoder-only Transformer object detector, called DeoT. First, we design a feature pyramid fusion module (FPFM) to generate fusion features with rich semantic information. The proposal of the FPFM not only improves the detection accuracy of objects, but also solves the detection problem of objects of different sizes. Second, we propose an encoder-only Transformer module (E-OTM) to achieve a global representation of features by exploiting deformable multi-head self-attention (DMHSA). Furthermore, we design a Transformer block residual structure (TBRS) in the E-OTM, which refines the output features of the transformer module by using the channel attention and spatial attention in the channel refinement module (CRM) and spatial refinement module (SRM). The proposal of encoder-only Transformer module not only effectively alleviates the complexity and learning convergence problems of the model, but also improves the detection accuracy. We conduct sufficient experiments on the MS COCO object detection dataset and Cityscapes object detection dataset, and achieve 50.9 AP with 34 Epochs on the COCO 2017 tes-dev set, 30.1 AP with 38 FPS on the Cityscapes dataset. Therefore, DeoT not only achieves high efficiency in the training phase, but also ensures real time and accuracy in the detection process.
更多查看译文
关键词
Object detector,Encoder-only Transformer,Convolutional neural network,Deformable attention
AI 理解论文
溯源树
样例
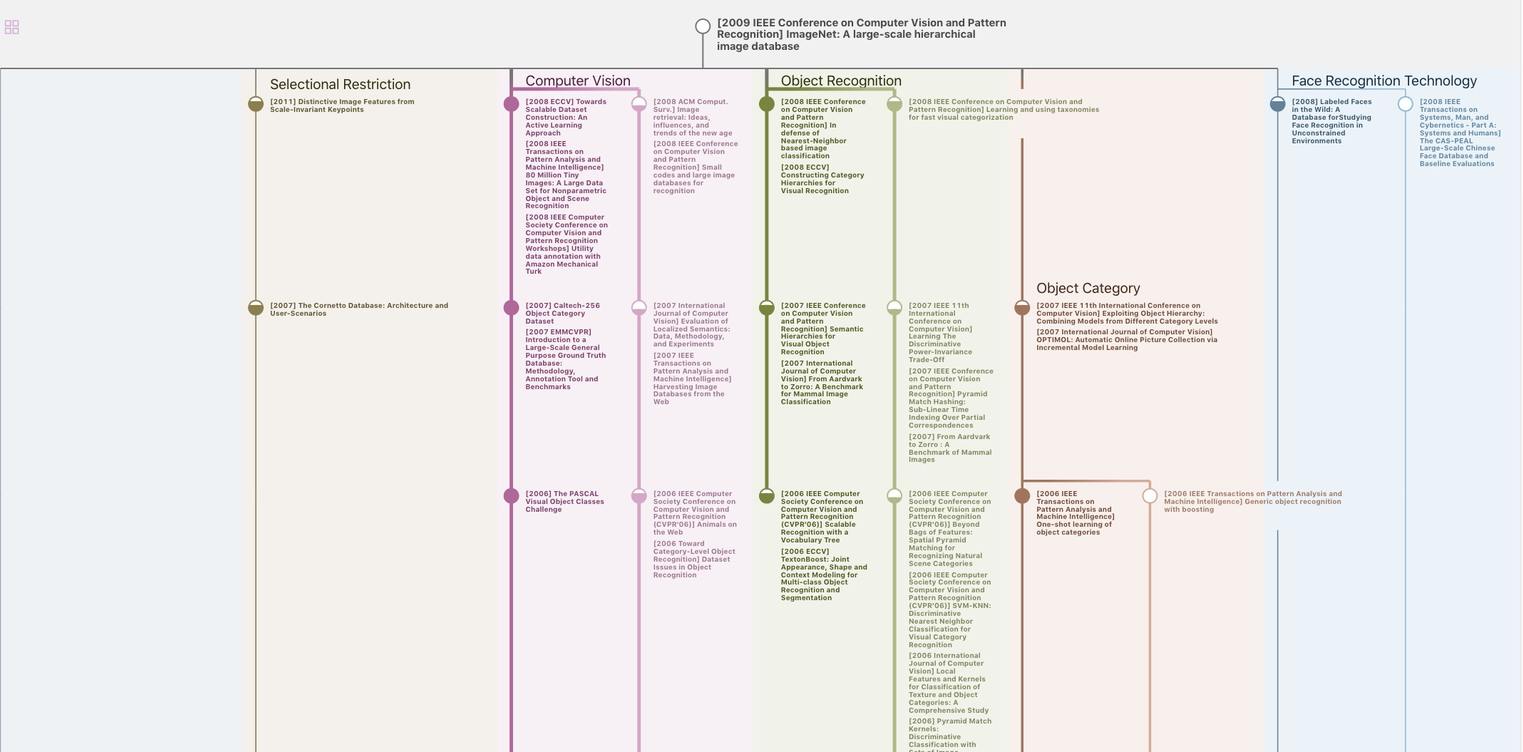
生成溯源树,研究论文发展脉络
Chat Paper
正在生成论文摘要