ERGCN: Enhanced Relational Graph Convolution Network, an Optimization for Entity Prediction Tasks on Temporal Knowledge Graphs
Future internet(2022)
摘要
Reasoning on temporal knowledge graphs, which aims to infer new facts from existing knowledge, has attracted extensive attention and in-depth research recently. One of the important tasks of reasoning on temporal knowledge graphs is entity prediction, which focuses on predicting the missing objects in facts at current time step when relevant histories are known. The problem is that, for entity prediction task on temporal knowledge graphs, most previous studies pay attention to aggregating various semantic information from entities but ignore the impact of semantic information from relation types. We believe that relation types is a good supplement for our task and making full use of semantic information of facts can promote the results. Therefore, a framework of Enhanced Relational Graph Convolution Network (ERGCN) is put forward in this paper. Rather than only considering representations of entities, the context semantic information of both relations and entities is considered and merged together in this framework. Experimental results show that the proposed approach outperforms the state-of-the-art methods.
更多查看译文
关键词
graph convolutional network,temporal knowledge graphs,entity prediction
AI 理解论文
溯源树
样例
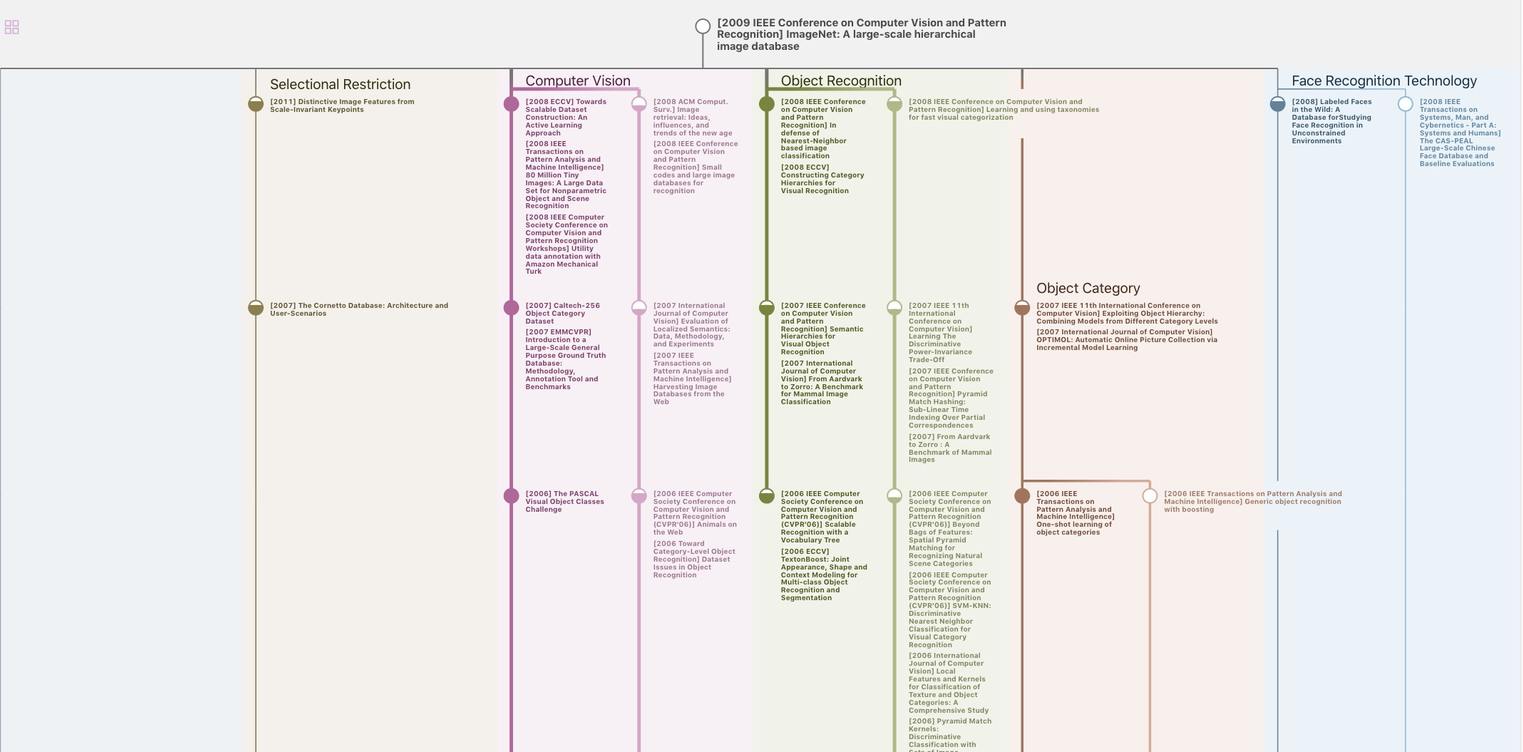
生成溯源树,研究论文发展脉络
Chat Paper
正在生成论文摘要