Individualized Dynamic Patient Monitoring Under Alarm Fatigue.
Oper. Res.(2022)
Abstract
Individualized Patient Monitoring Under Alarm Fatigue Hospitals are rife with alarms, many of which are false. This leads to alarm fatigue, in which clinicians become desensitized and may inadvertently ignore real threats. “Individualized Dynamic Patient Monitoring Under Alarm Fatigue” by Piri, Huh, Shechter, and Hudson studies the problem of personalizing alarm thresholds for vital signs at a hospital while considering the ”boy who cried wolf” effect of false alarms. The authors create a model that learns patients’ personal alarm thresholds during their hospital stay and updates their alarm settings dynamically. They formulate the problem as a partially observable Markov decision process. They provide structural properties of the optimal policy and perform a numerical case study based on clinical data from an intensive care unit. They show that dynamic methods of alarm settings that explicitly consider the feedback loop of false positives can significantly reduce patient harm when compared with current methods of alarm settings. Hospitals are rife with alarms, many of which are false. This leads to alarm fatigue, in which clinicians become desensitized and may inadvertently ignore real threats. We develop a partially observable Markov decision process model for recommending dynamic, patient-specific alarms in which we incorporate a cry-wolf feedback loop of repeated false alarms. Our model takes into account patient heterogeneity in safety limits for vital signs and learns a patient’s safety limits by performing Bayesian updates during a patient’s hospital stay. We develop structural results of the optimal policy and perform a numerical case study based on clinical data from an intensive care unit. We find that compared with current approaches of setting patients’ alarms, our dynamic patient-centered model significantly reduces the risk of patient harm.
MoreTranslated text
Key words
Policy Modeling and Public Sector OR,warning systems,alarm fatigue,cry-wolf effect,partially observable Markov decision process,threshold policy,neural networks
AI Read Science
Must-Reading Tree
Example
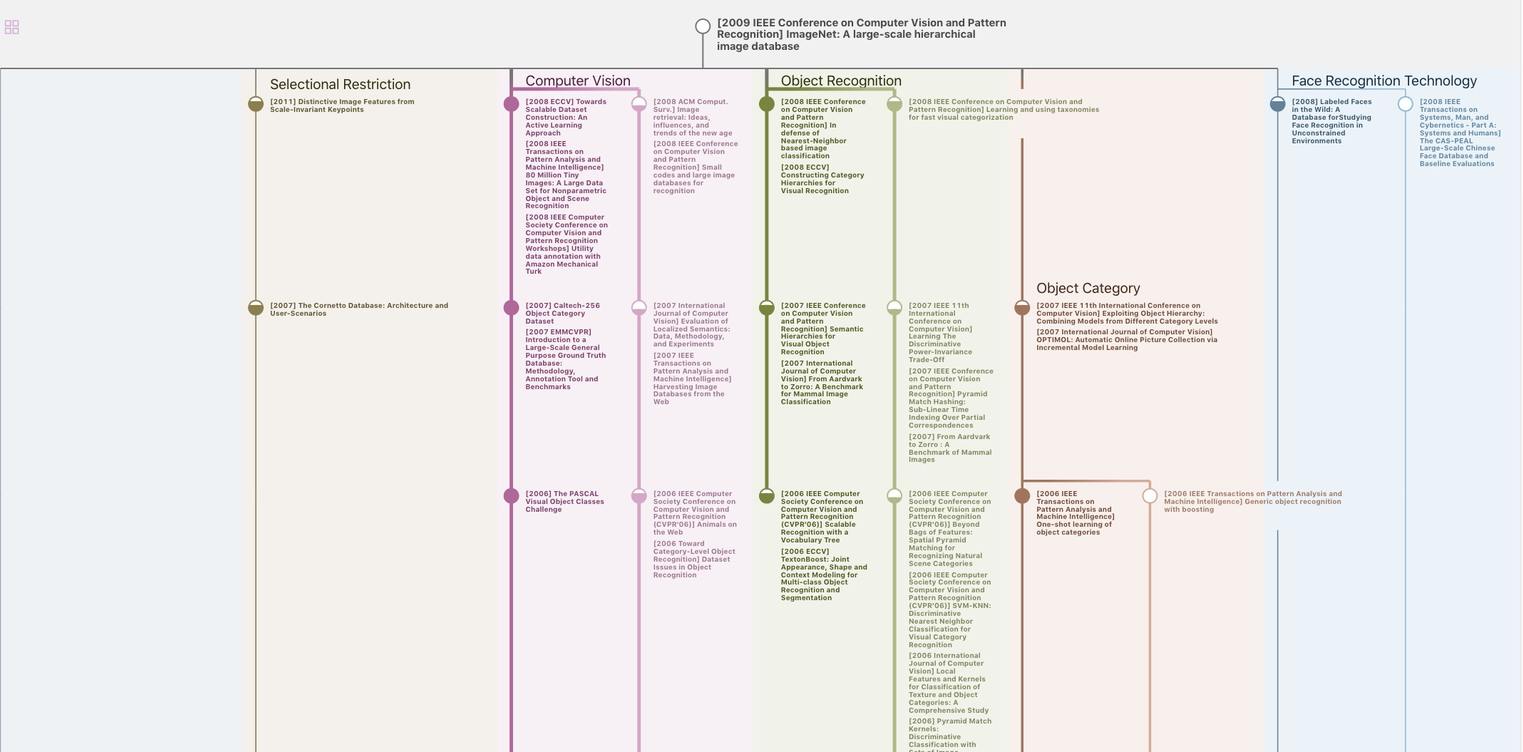
Generate MRT to find the research sequence of this paper
Chat Paper
Summary is being generated by the instructions you defined