Connecting metrics for shape-texture knowledge in computer vision
CoRR(2023)
摘要
Modern artificial neural networks, including convolutional neural networks and vision transformers, have mastered several computer vision tasks, including object recognition. However, there are many significant differences between the behavior and robustness of these systems and of the human visual system. Deep neural networks remain brittle and susceptible to many changes in the image that do not cause humans to misclassify images. Part of this different behavior may be explained by the type of features humans and deep neural networks use in vision tasks. Humans tend to classify objects according to their shape while deep neural networks seem to rely mostly on texture. Exploring this question is relevant, since it may lead to better performing neural network architectures and to a better understanding of the workings of the vision system of primates. In this work, we advance the state of the art in our understanding of this phenomenon, by extending previous analyses to a much larger set of deep neural network architectures. We found that the performance of models in image classification tasks is highly correlated with their shape bias measured at the output and penultimate layer. Furthermore, our results showed that the number of neurons that represent shape and texture are strongly anti-correlated, thus providing evidence that there is competition between these two types of features. Finally, we observed that while in general there is a correlation between performance and shape bias, there are significant variations between architecture families.
更多查看译文
关键词
computer vision,metrics,knowledge,shape-texture
AI 理解论文
溯源树
样例
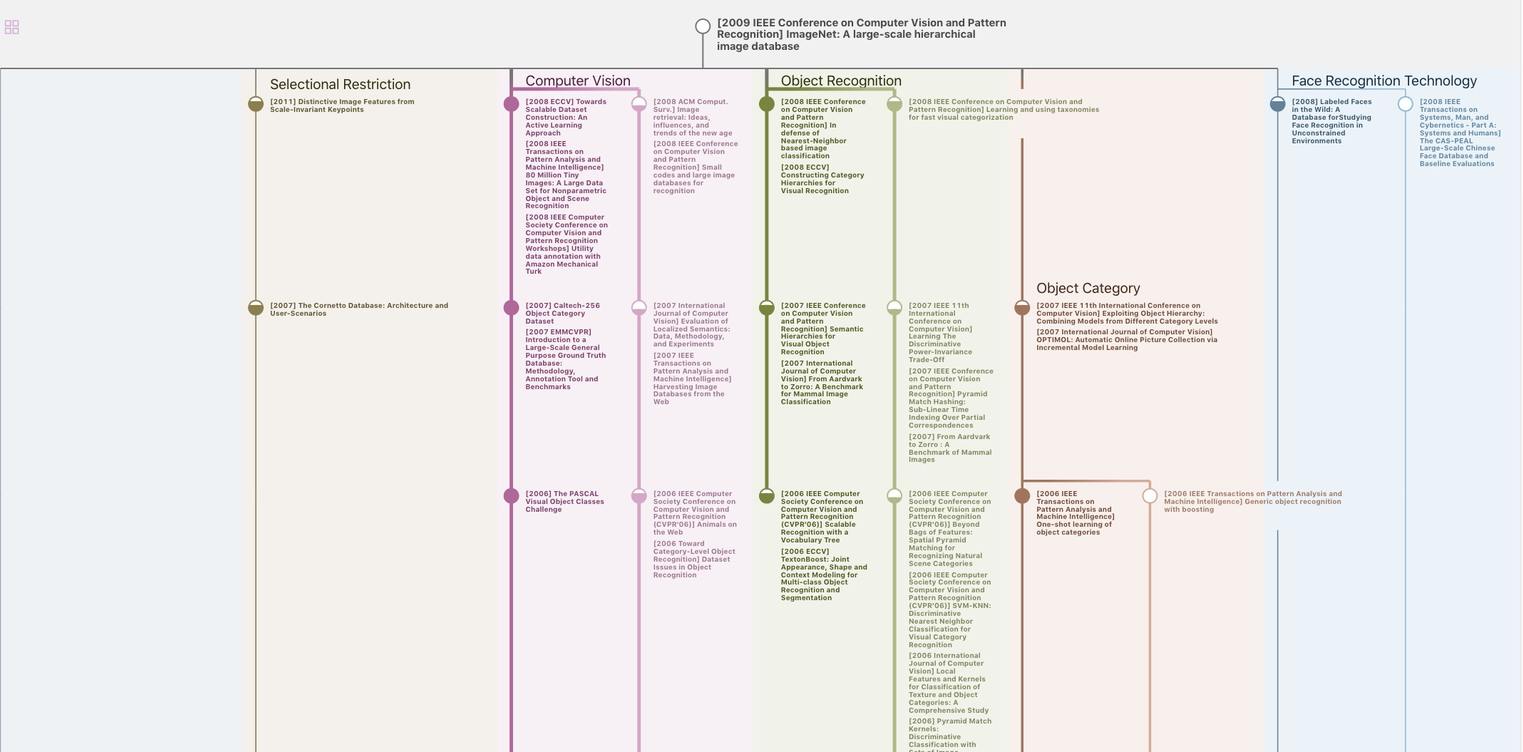
生成溯源树,研究论文发展脉络
Chat Paper
正在生成论文摘要