Uncertainty-Aware Model-Based Offline Reinforcement Learning for Automated Driving
IEEE robotics & automation letters(2023)
摘要
Offline reinforcement learning (RL) provides a framework for learning decision-making from offline data and therefore constitutes a promising approach for real-world applications such as automated driving (AD). Especially in safety-critical applications, interpretability and transferability are crucial to success. That motivates model-based offline RL approaches, which leverage planning. However, current state-of-the-art (SOTA) methods often neglect the influence of aleatoric uncertainty arising from the stochastic behavior of multi-agent systems. Further, while many algorithms state that they are suitable for AD, there is still a lack of evaluation in challenging scenarios. This work proposes a novel approach for Uncertainty-aware Model-Based Offline REinforcement Learning Leveraging pl A nning (UMBRELLA), which jointly solves the prediction, planning, and control problem of the self-driving vehicle (SDV) in an interpretable learning-based fashion. A trained action-conditioned stochastic dynamics model captures distinctively different future evolutions of the traffic scene. The analysis provides empirical evidence for the effectiveness of our approach and SOTA performance in challenging AD simulations and using a real-world public dataset.
更多查看译文
关键词
Planning,Uncertainty,Behavioral sciences,Stochastic processes,Predictive models,Reinforcement learning,Vehicle dynamics,Human-aware motion planning,intelligent transportation systems,machine learning for robot control,planning under uncertainty,reinforcement learning
AI 理解论文
溯源树
样例
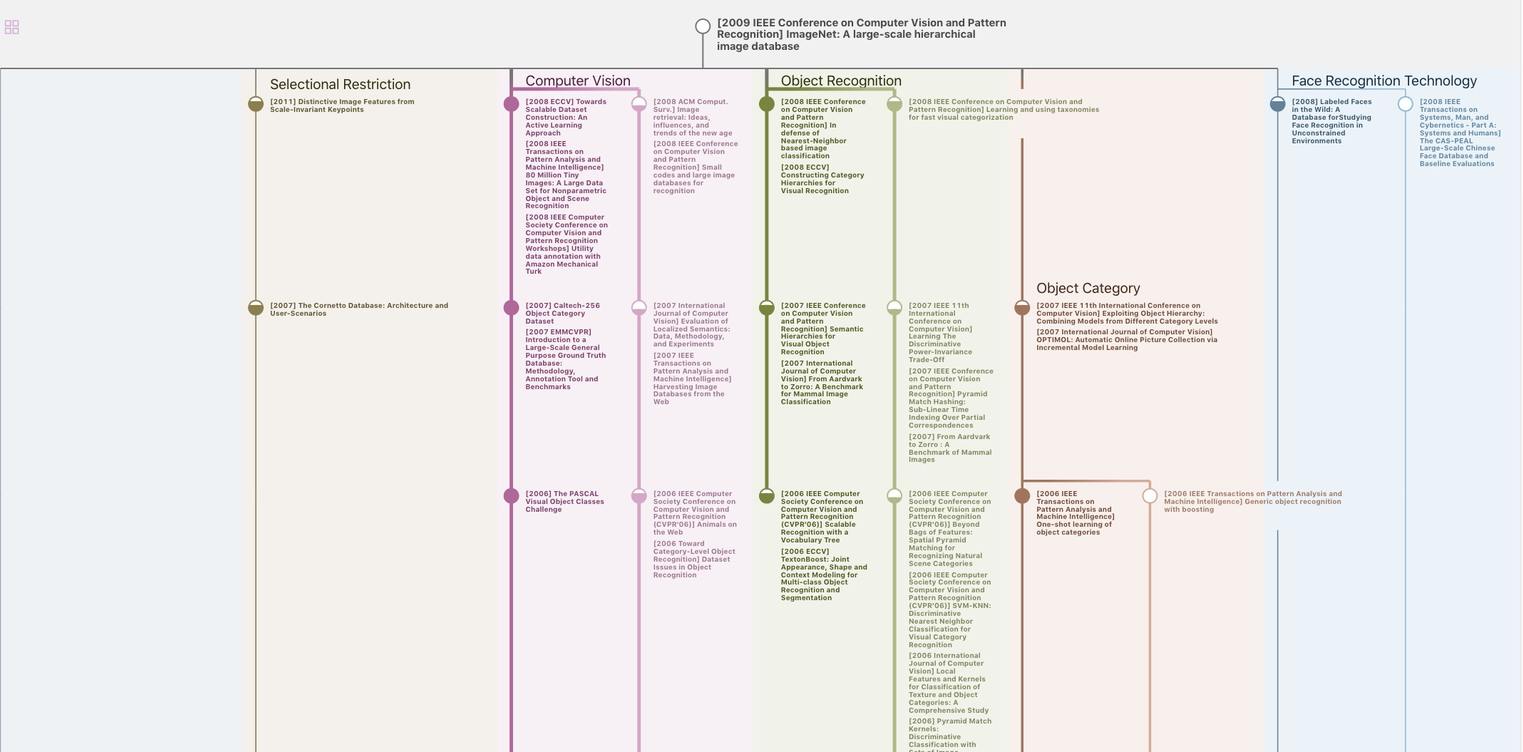
生成溯源树,研究论文发展脉络
Chat Paper
正在生成论文摘要