Space and Level Cooperation Framework for Pathological Cancer Grading.
2022 IEEE International Conference on Visual Communications and Image Processing (VCIP)(2022)
摘要
Clinically, the pathological images are intuitive for cancer diagnosis and have been considered as the ‘gold standard’. There are two challenges for applying deep learning into the pathological images analysis: the ultra-large size and the noisy annotations. A pathological image usually contains billions of pixels, which is unsuitable for normal classification models. Furthermore, the ultra-large size and mixed cancerous cells compel the doctor to draw rough boundaries of lesion area according to the cancerous level, which brings two kinds of noisy labels: space noise (annotating inaccurate scope of cancerous area) and level noise (annotating inaccurate cancerous level). Based on the above findings, we propose the space and level cooperation framework, comprising a space-aware branch and a level-aware branch, for pathological cancer grading with noisy annotations. The space-aware branch first turns the ultra-large image into a Multilayer Superpixel (MS) graph, significantly reducing the size and preserving the global features. Then, a global-to-local rectifying strategy is adopted to solve the space noise. The level-aware branch adopts different grouped kernels and a novel grading loss function to handle level noise. Mean-while, two branches cooperate through complementing missing features of each other for handling the above two challenges. Extensive experiments demonstrate that with noisy annotations, the proposed framework achieves SOTA performance on our HCC dataset and two public datasets.
更多查看译文
AI 理解论文
溯源树
样例
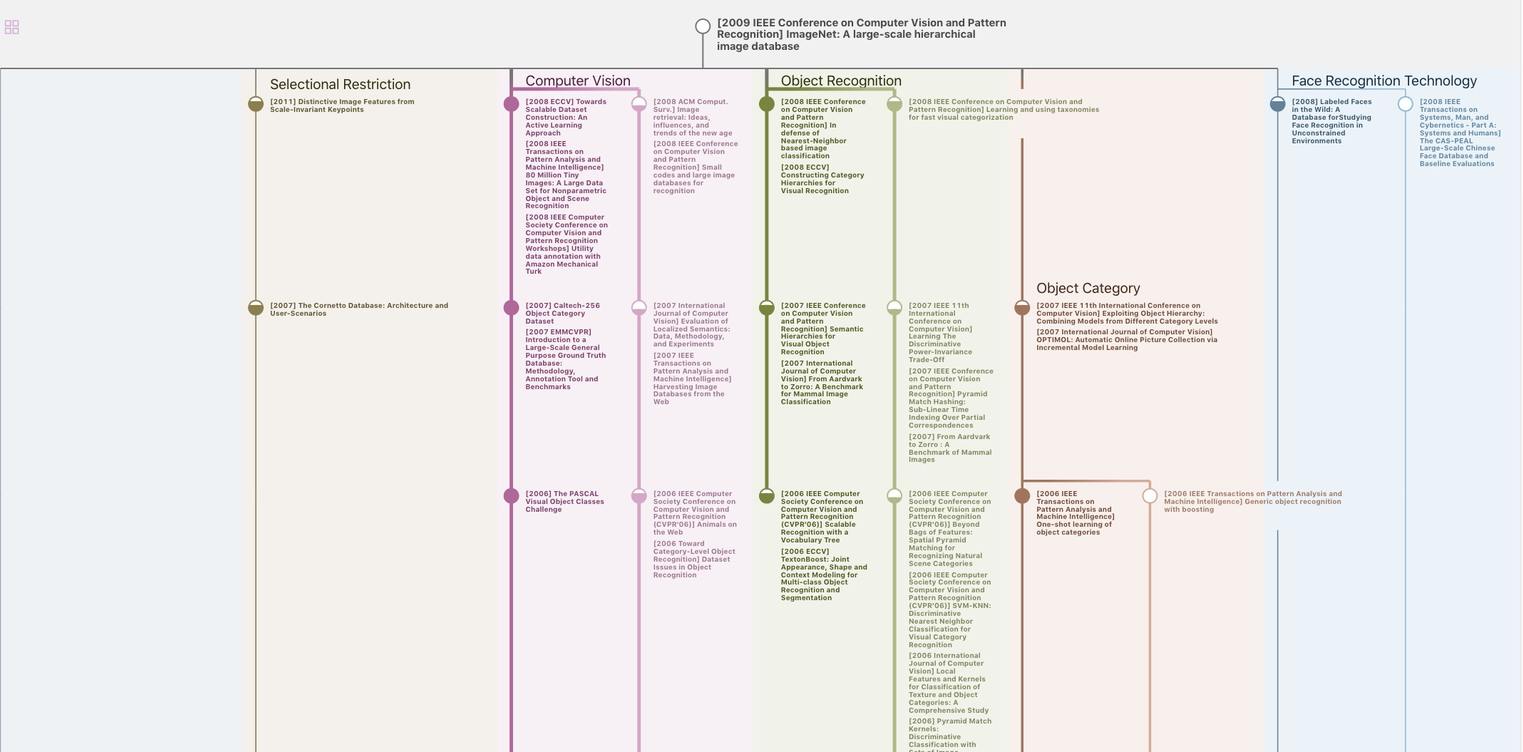
生成溯源树,研究论文发展脉络
Chat Paper
正在生成论文摘要