Minority-prediction-probability-based oversampling technique for imbalanced learning
Information Sciences(2023)
Abstract
In this study, we propose an oversampling method called the minority-predictive-probability-based synthetic minority oversampling technique (MPP-SMOTE) for imbalanced learning. First, MPP-SMOTE removes noisy samples from minority classes. Subsequently, it divides minority samples into two types (hard-to-learn and easy-to-learn) by predicting the probability of samples belonging to the minority class. For both sample types, we adopt a divide-and-conquer strategy. We separately calculate the probability of each sample being selected to generate a new synthetic sample. The relative density of a sample in both the majority and minority classes is considered in the method for calculating the selection probability of hard-to-learn samples, and the relative density of a sample in only the minority class is considered in that of easy-to-learn samples. Finally, according to the types and selection probabilities, MPP-SMOTE separately selects samples and generates synthetic samples based on them by using different sample-generation schemes. Experimental results reveal that the proposed method outperforms other oversampling methods in terms of three imbalanced-learning metrics for three common classifiers. According to the results, when a support vector machine classifier is applied, the area under the curve performance of the MPP-SMOTE improves by a factor of 1.44%.
MoreTranslated text
Key words
Imbalanced learning,Oversampling technique,Prediction probability,Selection probability,Sample generation
AI Read Science
Must-Reading Tree
Example
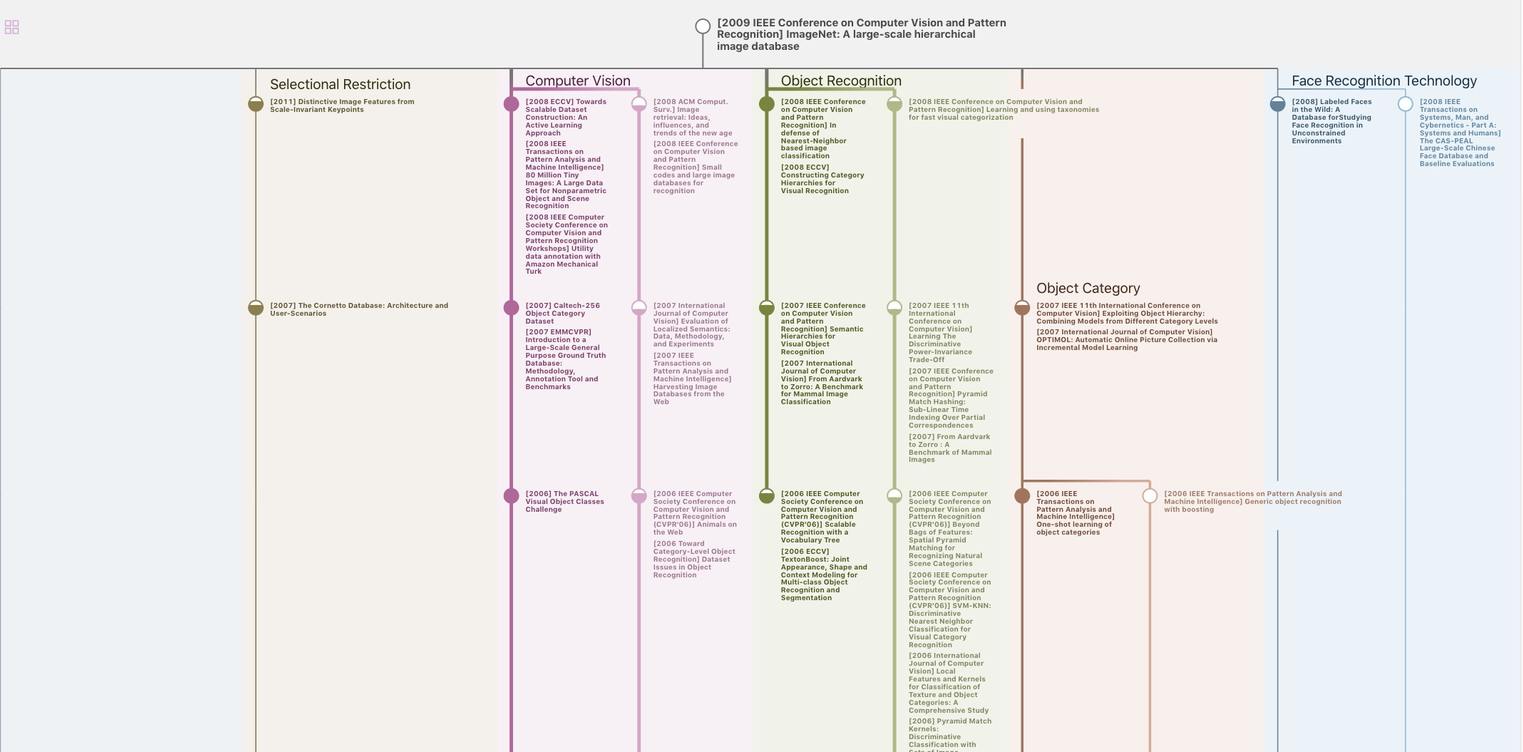
Generate MRT to find the research sequence of this paper
Chat Paper
Summary is being generated by the instructions you defined