Memory-based Deep Reinforcement Learning for Humanoid Locomotion under Noisy Scenarios
2022 Latin American Robotics Symposium (LARS), 2022 Brazilian Symposium on Robotics (SBR), and 2022 Workshop on Robotics in Education (WRE)(2022)
Abstract
This paper proposes a model-free memory augmented Deep Reinforcement Learning (DRL) method that can deal with noisy sensors in humanoid locomotion. DRL-based agents are promising for automatically learning how to control robots in complex simulated environments. However, they are still not fully addressed with model-free control algorithms in challenging noisy scenarios for humanoid robots. This work shows how the Soft Actor-Critic (SAC) algorithm can benefit from the memory effect introduced by LSTMs to mitigate the side effects of Partially Observed Markov Decision Processes (POMDP). We demonstrate that LSTM-SAC is a viable path towards DRL for POMDP by applying it in a bipedal locomotion task with the NAO Robot in various noisy scenarios.
MoreTranslated text
AI Read Science
Must-Reading Tree
Example
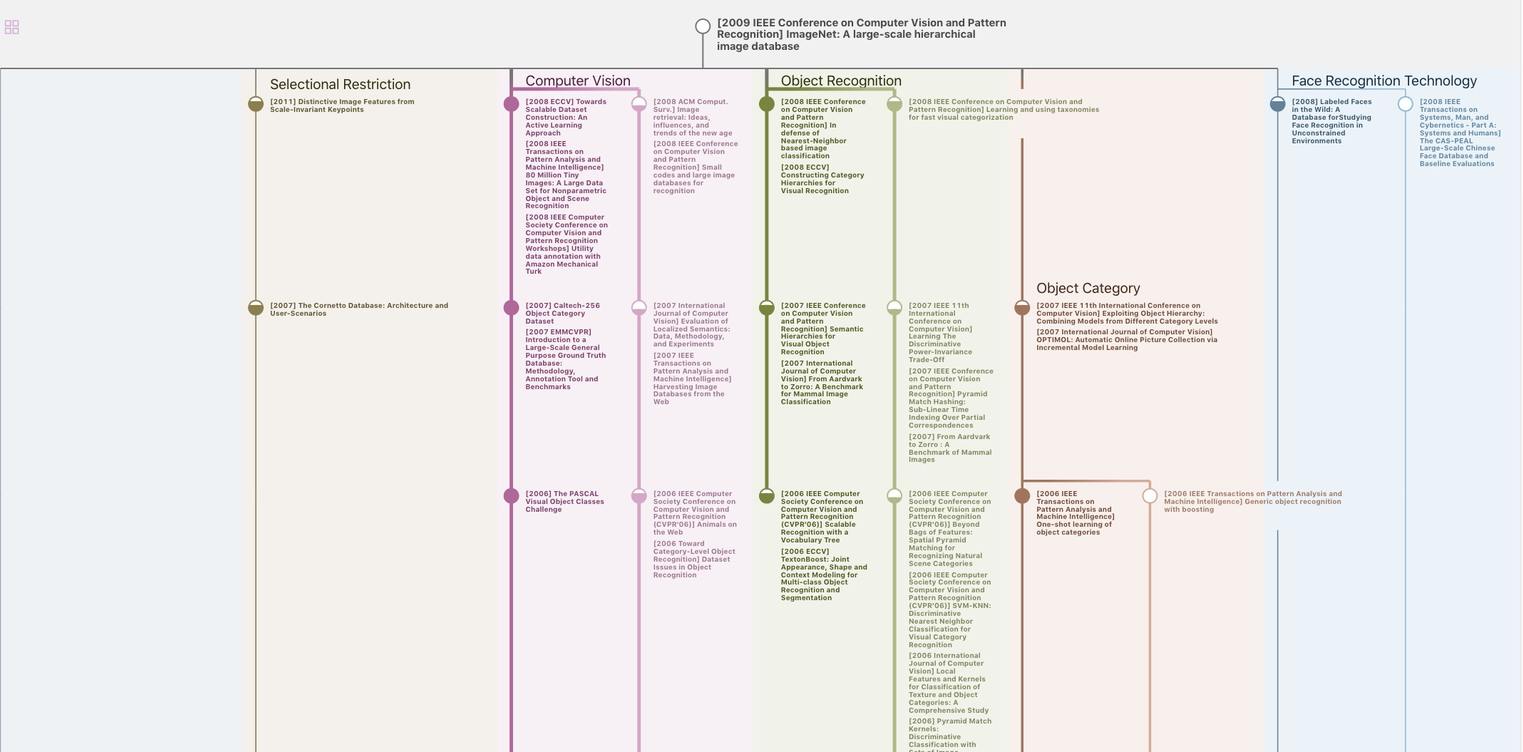
Generate MRT to find the research sequence of this paper
Chat Paper
Summary is being generated by the instructions you defined