eFIN: Enhanced Fourier Imager Network for generalizable autofocusing and pixel super-resolution in holographic imaging
CoRR(2023)
Abstract
The application of deep learning techniques has greatly enhanced holographic imaging capabilities, leading to improved phase recovery and image reconstruction. Here, we introduce a deep neural network termed enhanced Fourier Imager Network (eFIN) as a highly generalizable framework for hologram reconstruction with pixel super-resolution and image autofocusing. Through holographic microscopy experiments involving lung, prostate and salivary gland tissue sections and Papanicolau (Pap) smears, we demonstrate that eFIN has a superior image reconstruction quality and exhibits external generalization to new types of samples never seen during the training phase. This network achieves a wide autofocusing axial range of 0.35 mm, with the capability to accurately predict the hologram axial distances by physics-informed learning. eFIN enables 3x pixel super-resolution imaging and increases the space-bandwidth product of the reconstructed images by 9-fold with almost no performance loss, which allows for significant time savings in holographic imaging and data processing steps. Our results showcase the advancements of eFIN in pushing the boundaries of holographic imaging for various applications in e.g., quantitative phase imaging and label-free microscopy.
MoreTranslated text
Key words
Digital holography,deep learning,phase retrieval,autofocusing,pixel super-resolution,physics-informed learning,computational imaging,hologram reconstruction
AI Read Science
Must-Reading Tree
Example
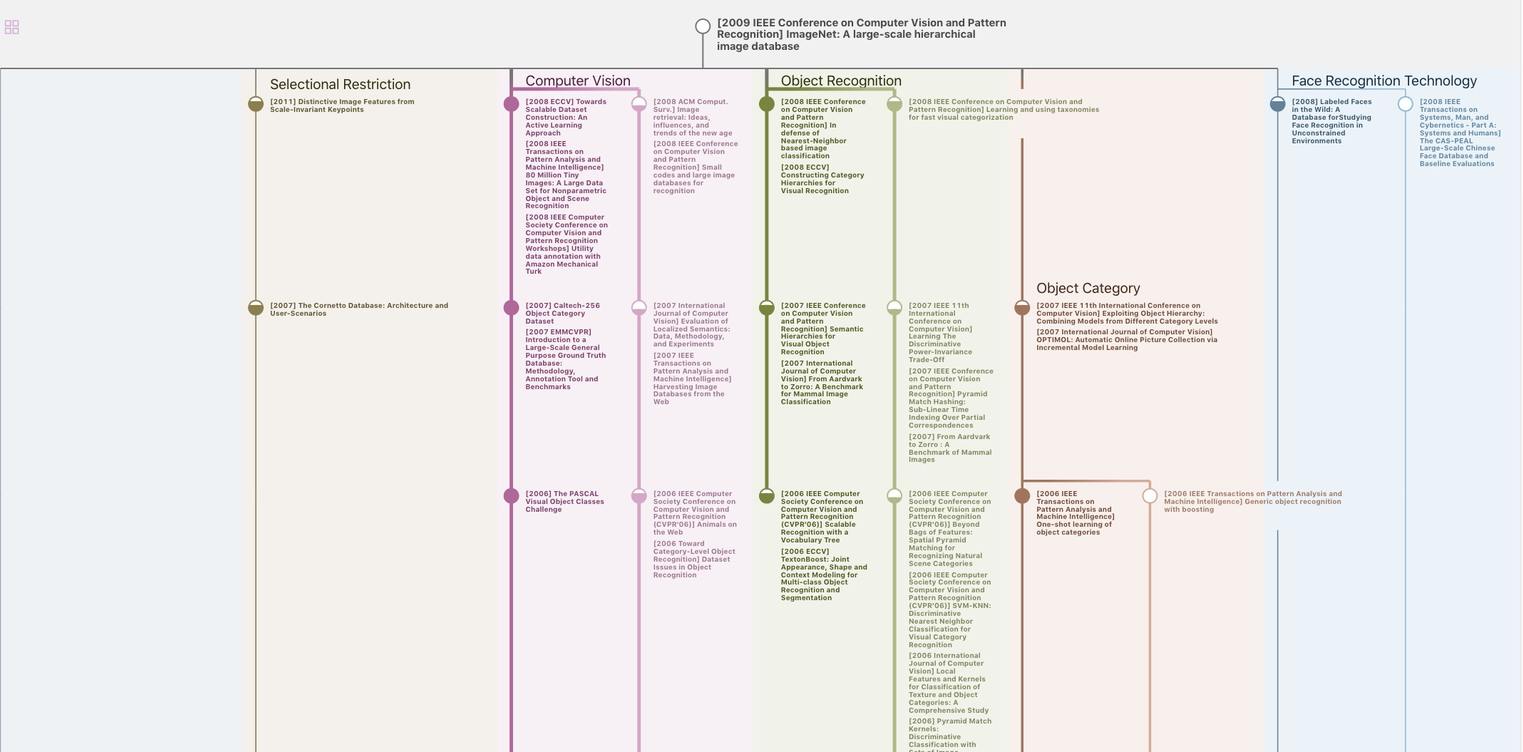
Generate MRT to find the research sequence of this paper
Chat Paper
Summary is being generated by the instructions you defined