A Fast and Time-Efficient Glitch Classification Method: A Deep Learning-Based Visual Feature Extractor for Machine Learning Algorithms
Astronomy and computing(2023)
摘要
Glitches are non-Gaussian transient waves that mimic gravitational-wave signals. As the glitches are abundant in the detector, the data quality is significantly impacted. Thus, it is crucial to identify glitch types to eliminate from the data and unveil the true astrophysical events. In this work, we explore the performance of machine learning algorithms, logistic regression, extreme gradient boost, and support vector machines for glitch classification using features extracted by employing the transfer learning method on Inception-v3 and ResNet-50 deep learning models. The transfer learning approach is carried out using two different methods. The first strategy relies on fine-tuning a pre-trained model using our dataset, whereas the second approach employs pre-trained models as feature extractor modules. Our findings demonstrate a significant improvement in training time with the transfer learning method followed by machine learning algorithms compared to the fine-tuning approach. With the approach having the lowest training time of 37.6 s, we were able to acquire a classification accuracy of 93.98%. Compared to the model trained with fine-tuning, transfer learning provides 24 and 31 times faster training time in ResNet-50 and Inception-v3, respectively, which could be enormously advantageous for the glitch classification process in the LIGO experiment.(c) 2022 Elsevier B.V. All rights reserved.
更多查看译文
关键词
Deep learning,Glitch classification,Gravitational waves,LIGO,VIRGO,Visual feature extractor
AI 理解论文
溯源树
样例
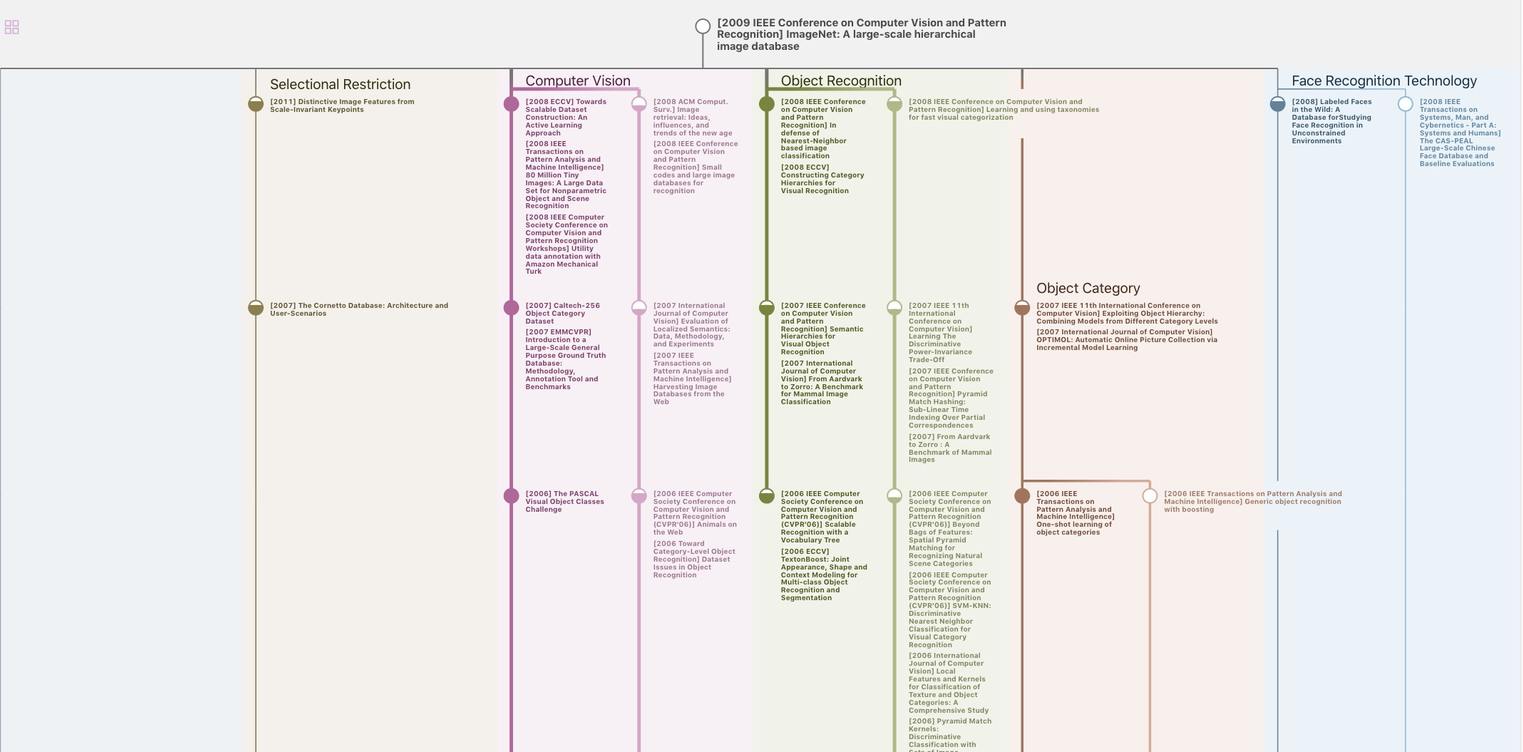
生成溯源树,研究论文发展脉络
Chat Paper
正在生成论文摘要